The digital transformation in the past decade has revolutionized the importance of data across all industries. The normal perception of big data has been altered from mega and giga to tera and peta. The masses of unexplored big data have created a demand on data experts to discover hidden trends, derive meaningful insights, and define strategies for offering new pathways or modifying existing approaches. Over the last few years, the market opportunities for data scientists have increased exponentially.
Data scientist has become one of the hottest jobs in the marketplace, leaving behind positions such as software engineers. As results of the rapid growth, demand, and the high-range salaries, data science and big data have become a trending fashion where everyone wants a piece of the pie. Consequently, it is not surprising to find a lot of posers suffering from FOMO (fear of missing out) who claim to be data scientists without having the right set of qualifications. The trending big data fashion does not justify the hiring of talent without the proper skill set or qualifications.
Nowadays the title of a data scientist is used loosely as a reference for a conventional data analyst or someone in a business intelligence role. Yet, the qualifications and differences are vast among these positions. A data scientist should have a solid foundation in the following areas: mathematics, statistics, modeling, problem-solving, validation, and visual analytics. Data science is a dynamic and extensive field which is rapidly evolving. In this field there is a need for quick learning which requires a great deal of effort to stay relevant in your knowledge of your business applications.
There are some telltale signs for data science posers. First is the lack of mathematical, computational, and a statistical modeling background. Real data science problems are complicated and finding the right solution requires a well-structured mathematical/statistical model as well as a thought-out procedure for validating the proposed model. Candidates with statistical, engineering, or computer science backgrounds (a combination of academic and professional experiences) may have the right qualifications to tackle these problems.
Besides a technical background, experience in dealing with data from scratch is equally important. Cleaning and organizing unstructured data sets are often the most time-consuming steps in data science problems. Previous experience in dealing with raw data is a key qualification and can help a candidate have a realistic proposal for addressing data science problems as well as selecting the right set of tools.
The Danger of FOMO in Data Science
There is a high risk for hiring an unqualified data poser for a data science role. Data scientists have to tell a story based on the given raw data and find a meaningful and scientific reason to defend the data story. These data stories are the foundation of many strategic decisions ranging from engineering design projects, to marketing, to medical research.
Normally, data story consumers are focused on summarized results and highlights instead of details of the analysis. It’s a data scientist’s responsibility to identify the significance of the data and to present it in a simple but scientific manner. This is where the risk of data posers in a data science role magnifies—misrepresenting a data story spiced with complexities of scientific terms which do not represent the truth behind the data. The rest of the story and consequences are clear: Failed engineering designs, and unsuccessful marketing strategies. There are a lot of relevant examples in the petroleum industry. With advances in subsurface logging methods and real-time monitoring systems for drilling or production operations, there are a lot of data available from the full cycle of petroleum development projects, which can be used as a foundation for great data stories. Examples include utilizing a wide range of subsurface data for reservoir modeling and reserve estimates, geomechanical modeling based on log-derived rock properties for designing a stable wellbore while drilling, or analyzing production data for predicting future production trends.
Consequences of inaccurate data stories in any of the above examples can be costly, such as a failed wellbore while drilling because of a failed geomechanical modeling design or a failed production strategy based on an inaccurate prediction. Even though these engineering challenges may have existed before, the importance of data has magnified with the boom in data-generating technology and as the demands for real-time approaches increase. Resolving these engineering challenges requires experts with true data science skills in addition to engineering knowledge.
Data science is a fast growing and dynamic field where boundaries are rapidly expanding by new findings and technology advances. It is an exciting field which attracts a lot of people from different backgrounds. If this is what you really like, follow your passion—however be up for the challenges. Nobody expects you to know everything but you have to be honest about your skill sets and stay humble to constantly educate yourself. Never hesitate to share your thoughts, ideas, and approaches with seasoned data scientists and peers as their experience can add priceless value to your work.
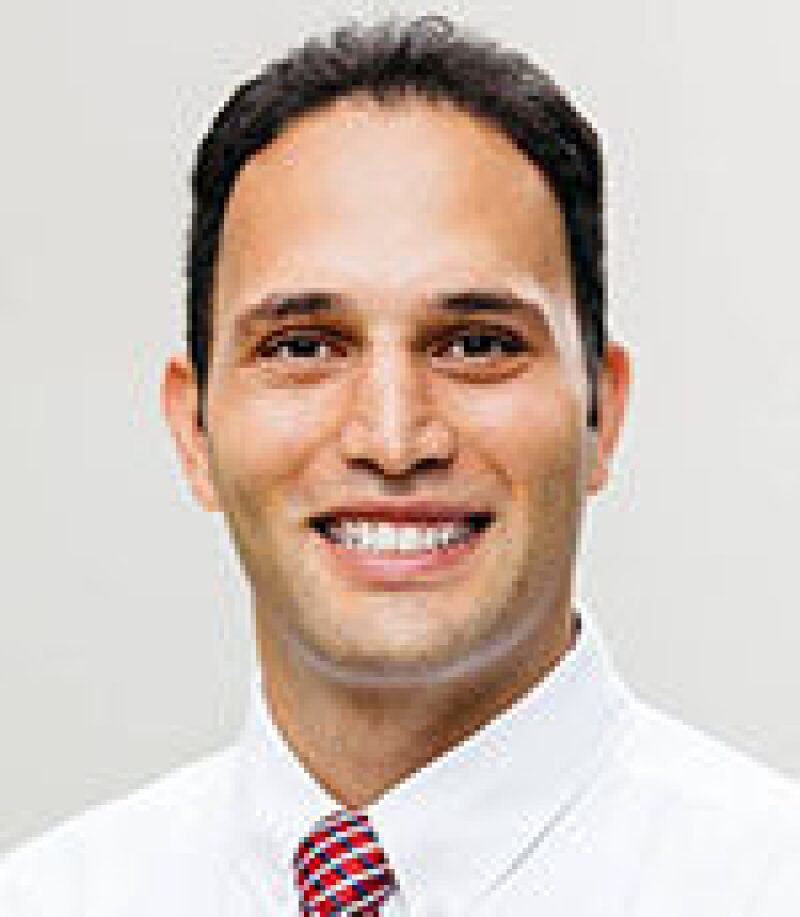
Reza Rahimi is a sidetracked petroleum engineer working with Mastercard as a senior data scientist. Over the past 8 years, Rahimi held various research, engineering, and operational positions in areas of offshore drilling, well construction, and geomechanics. He received his PhD and master’s degrees in petroleum engineering from the Missouri University of Science and Technology, and a bachelor’s degree in petroleum engineering from the University of Petroleum Technology. Rahimi is a member of the TWA Editorial Committee and has been an active member of SPE since 2005.
Related Content
1. Sidetrack Is Not Always an Expensive Choice: Job Hunting Strategies for Young Professionals
2. Day in the Life of a Data Scientist