Why Do We Test Wells?
The main reason for testing an exploration well is to take a fluid sample. Further reasons are to measure the initial pressure, estimate a minimum reservoir volume, evaluate the well permeability and skin effect, and identify heterogeneities and boundaries. Testing producing wells aims at verifying permeability and skin effect, identifying fluid behavior, estimating the average reservoir pressure, confirming heterogeneities and boundaries, and assessing hydraulic connectivity.
How Do We Test Wells?
We create a step change in rate—for instance, by closing a flowing well or an injection well (buildup or falloff, respectively); by opening a well previously shut in (drawdown); or by injecting in a well previously closed (injection). This rate change creates a change in pressure in the same well (exploration or production testing) or in a different well (interference testing). In layered reservoirs, there is also a change in the rates from each individual layer, which can be measured with a production logging tool (PLT).
A change in rate can be created at the surface by shutting or opening the master valve or at the bottom of the well with a special downhole shut-in device. Wellhead shut-in is commonly used in wells already in production, whereas bottomhole shut-in is standard practice after drilling [a drillstem test (DST)]. The way the rate signal is created is not important as far as well test analysis is concerned. The same interpretation methods are used for production tests, DSTs, analysis of wireline formation tests, and now for testing while drilling. What is most important for analysis is the quality of the rate input signal—which must be of the proper shape and duration—and the quality of the measured pressure output signal.
How Do We Interpret Well Tests?
We try to identify an interpretation model that relates the measured pressure change to the induced rate change and is consistent with other information about the well and reservoir. This is an inverse problem without a unique solution. Petroleum professionals are confronted with the inverse problem whenever they interpret data and model processes (for instance, in geophysical interpretation, in geological interpretation, in log interpretation, and in the reservoir modeling aspect of reservoir simulation). The problem of nonuniqueness is well recognized in the oil industry and accounts for the increasing use of stochastic modeling techniques, which aim at providing alternative equiprobable representations of the reservoir to capture the uncertainty associated with predictions. Nonuniqueness decreases as the amount of information increases.
As illustrated in Fig. 1, there are two possible signals we can use to identify an interpretation model. One is the difference Δp=[p(Δt)−p(Δt=0)] between the pressure p(Δt) at an elapsed time Δt in a flow period and the pressure p(Δt=0) at the start of the flow period (a flow period is a period during which the rate is constant). This signal and its derivative with respect to the superposition time are plotted on a log-log graph. In such a graph, various flow regimes (e.g., linear, bilinear, spherical, radial) exhibit distinctive shapes and occur at different times, and this is used to identify them (log-log pressure and derivative analysis). The existence of the flow regimes can be verified on flow-regime-specialized graphs by plotting Δp=[p(Δt)−p(Δt=0)] vs. f(Δt) on a Cartesian graph (specialized analysis), where f is a flow-regime-specific function. f(Δt) is equal to Δt for wellbore storage and pseudosteady-state flow, for linear flow, for spherical flow, log(Δt) for radial flow, etc.
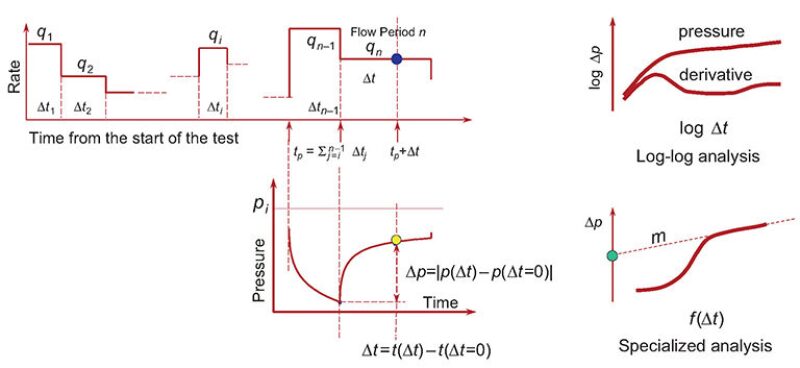
The other signal is [pi−p(Δt)], where pi is the initial pressure (Fig. 2). Because pi is usually not known, the signal is actually p(Δt), to be plotted against a flow-regime-specific superposition time, on a Cartesian plot (Horner analysis). f(Δt) is the same as for specialized analyses. In both specialized and Horner analyses, a straight line is obtained where the flow regime dominates and the straight-line slope and intercept provide the well and reservoir parameters that control this flow regime.
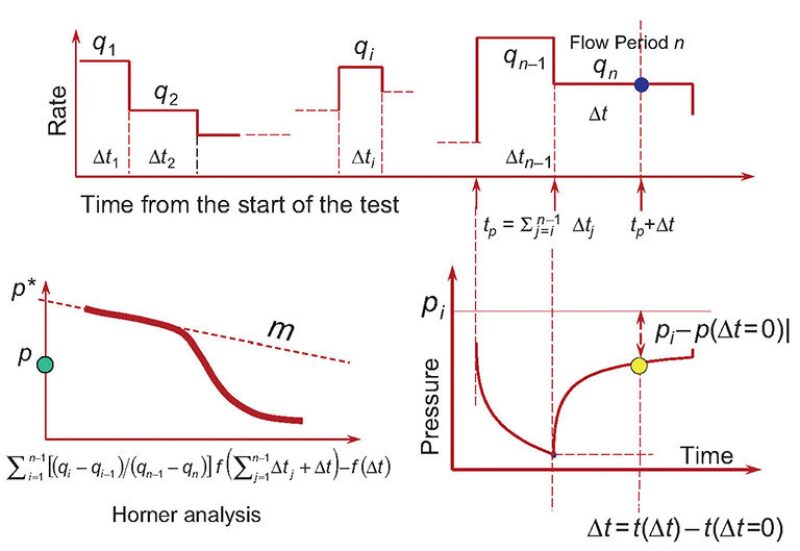
What Is a Well Test Interpretation Model?
The interpretation model is made of the combination of the individual flow regime components that dominate the flow period at different times. The number of interpretation model components is limited to three types (Fig. 3), namely
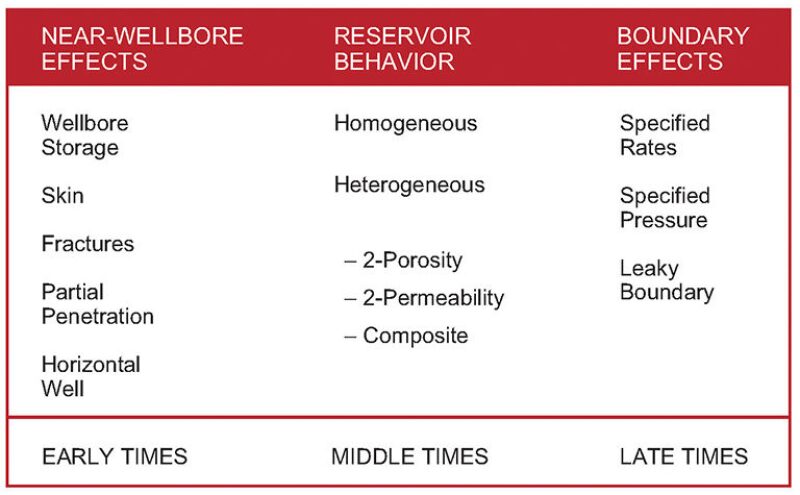
- The basic dynamic behavior of the reservoir during middle times, which is usually the same for all the wells in a given reservoir
- Near-wellbore effects at early times resulting from the well completion that may vary from well to well or from test to test
- Boundary effects at late times, determined by the nature of the reservoir boundaries, which is the same for all the wells in a given reservoir, and by the distance from the well to these boundaries, which may differ from well to well
Although there are few possible interpretation model components, their combination can yield several thousand different interpretation models to match all observed well behavior. The challenge for the well test interpreter is to diagnose from the observed well behavior which components should be included in the interpretation model. A schematic of the complete interpretation process is shown in Fig. 4.
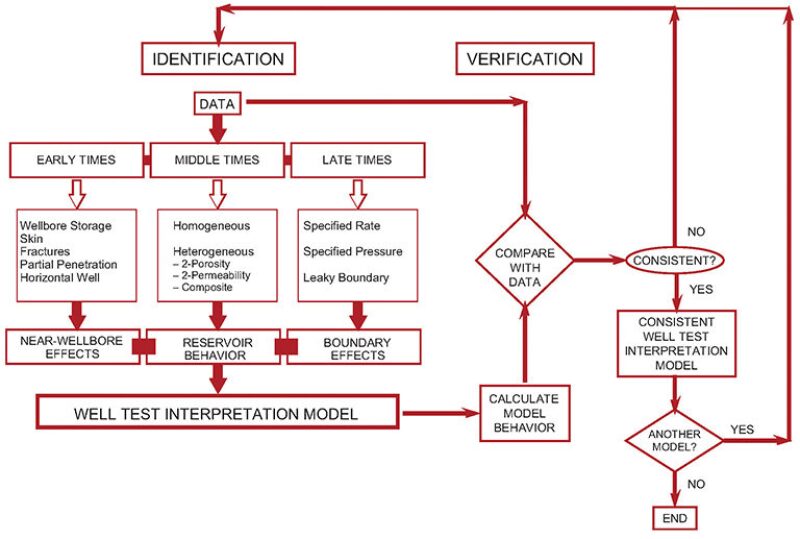
What Is the Difference Between the Various Interpretation Methods?
The main difference between the available analysis techniques is their ability to diagnose and verify an interpretation model efficiently. In this respect, the derivative log-log analysis method is much better than the log-log pressure analysis method. Both are significantly better than straight-line-based techniques used in specialized and Horner analyses. Specifically, straight-line techniques, although simple to use, are poor at selecting the very straight lines on which they are to be applied. And, once a straight line has been selected, there is no rule to indicate if it is indeed the correct one (i.e., the one corresponding to the flow regime being analyzed). This is why, when powerful personal computers became available, the derivative approach superseded log-log pressure analysis, which before had superseded straight-line techniques. This does not mean that new techniques have eliminated previous ones. These are still used, but they are integrated in a methodology that allows them to be applied correctly.
Pressure derivatives combine great diagnosis and verification capabilities with the accuracy of straight-line methods. Derivative shapes for various flow regimes at early, middle, and late times in a flow period are distinctly different, which is not necessarily the case with pressure change. For instance, spherical flow is easy to identify on the derivative, whereas it is invisible on the pressure drop curve. The main drawback of derivatives, however, is that, contrary to pressure data, they are not measured but must be calculated. A number of factors can affect the shape of the derivative curve and, therefore, mislead the interpreter. Some can be easily identified: derivation algorithm, sampling frequency of the data acquisition, gauge resolution, time or pressure errors at the start of the period, erratic raw data points, or multiphase flow. Others are more difficult to see and may affect the analysis. These include end effects (if the last pressure in a flow period is too high or too low, the derivative shows an upward or downward trend, which must not be confused with a boundary effect), phase redistribution in the wellbore, and a pressure trend in the reservoir. But the most impact by far comes from the rate history. Oversimplifying the flow-rate history can jeopardize the reliability of the pressure derivative as a diagnostic tool (this holds true also for Horner analysis).
What Is Well Test Deconvolution?
Deconvolution transforms variable-rate pressure data into a constant-rate initial drawdown with a duration equal to the total duration of the test and directly yields the corresponding pressure derivative, normalized to a unit rate. This derivative is free from the distortions caused by the pressure-derivative calculation algorithm and from errors introduced by incomplete or truncated rate histories.
Deconvolution is not a new interpretation method but rather is a new tool to process pressure and rate data in order to increase the amount of data that can be analyzed with derivative, pressure, and straight-line analyses. The gain is clearly greater in long tests, such as with permanent downhole pressure gauges, in which the total test duration is one or two orders of magnitude greater than the duration of the longest flow period at constant rate. Deconvolution, however, is also useful in short tests such as DSTs because it gives access to a greater radius of investigation and enables differentiation between true test behavior and artifacts of the derivative calculation.
An example of deconvolution is shown in Fig. 5. The red curve in Fig. 5b is the deconvolved derivative obtained by deconvolution of the entire rate history shown in Fig. 5a. Its duration, equal to the total production time, is two orders of magnitude greater than the longest buildups, represented by discrete points in Fig. 5b. The shift between the deconvolved derivative and the buildup data in Fig. 5b is from the rate history before the respective buildups. In this particular example, the extended derivative showed contribution to production from a lower layer after 104 hours. This could not be seen from the longest buildups, limited to 103 hours.
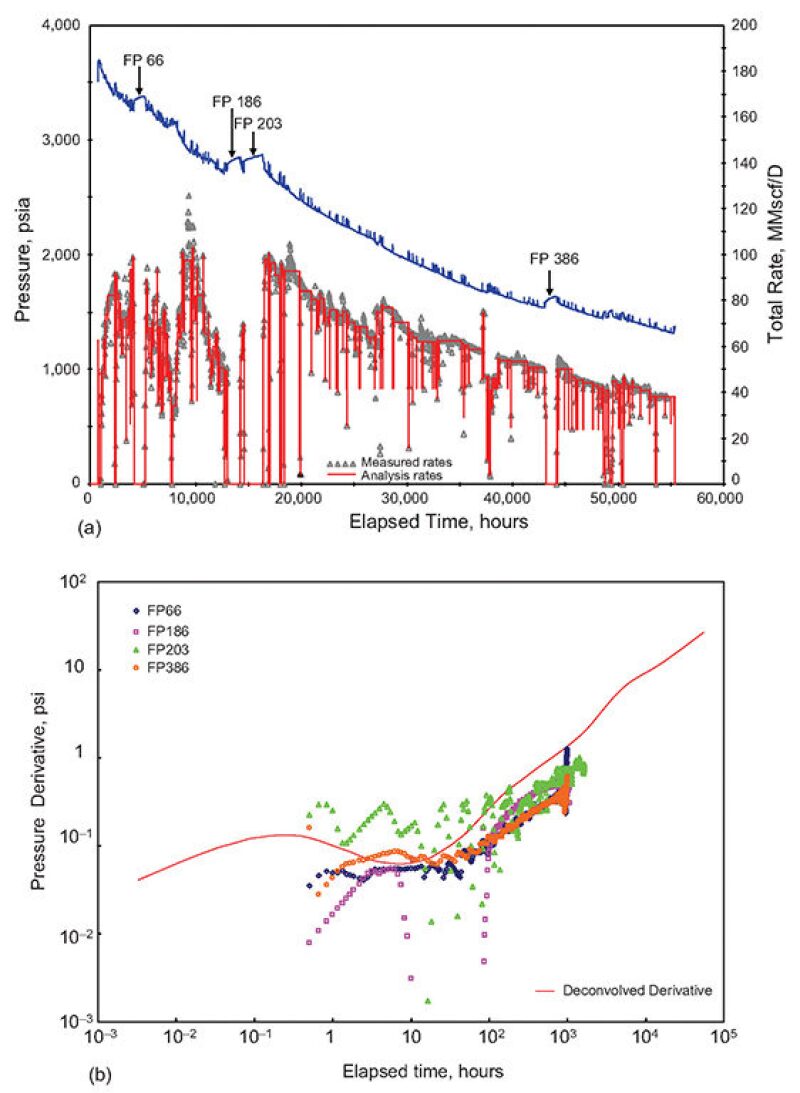
Deconvolution actually blurs the difference between conventional well test and production-data analysis. During the course of many years, several methods have been proposed to analyze production data to extract all the information that is usually obtained from conventional well test analysis without the constraint of shutting in wells. These methods have been attempting to convert variable rate and pressure into variable pressure at constant rate or into variable rate at constant pressure. Examples are the decline curve analysis by use of material balance time, the reciprocal productivity index method, and the rate/time type curve. The aim of all these methods is achieved with deconvolution, which produces much cleaner transformed data and much better results when estimating permeability and distances to boundaries.
What’s Next?
Improvements in well test analysis will essentially come from three areas: richer signals (i.e., those containing more information), better interpretation techniques (providing significant improvements in the identification and validation of the interpretation model), and more-complex models that represent the geology better. Reservoir geology is very complex, whereas well test interpretation models are rather simple. Some of the geological complexity can be seen and quantified from well test analysis with more-complex interpretation models that represent geological bodies more closely. For instance, vertical permeability and meander information in a fluvial meandering channel can be found from well test data in the transition between radial flow in middle times and channel flow at late times. The corresponding data are ignored when the analysis is performed with the usual simple interpretation models.
Efforts to reduce costs and environmental impact are also likely to impose additional changes. Well testing in exploration and appraisal wells has become increasingly unpopular in recent years. Reasons include cost, safety, and environmental impact. Well testing also has become rare in production wells because of the potential revenue loss during buildups. Whether suitable alternatives can be found is the subject of regular debate. Alternatives to DSTs include wireline formation tests and mini-DSTs for sampling, permeability, and initial reservoir pressure; core and log analyses for permeability; and geology, seismic analysis, and geochemistry for reservoir heterogeneities, boundaries, and fluid contacts. However, there is no suitable well-testing replacement for finding skin (well damage), effective permeability, and hydraulic connectivity throughout large reservoir volumes and obtaining the large fluid samples required for sizing surface processing facilities or for determining the quality of the fluids from a commercial viewpoint. Production tests, on the other hand, tend to be replaced by continuous recording with permanent pressure and rate gauges in production wells. These data are particularly well suited for analysis with deconvolution.
Conclusions
Well test analysis has come a long way since the 1950s when the interpretation methods on the basis of straight lines gave unreliable results. We now have a methodology that provides repeatability and techniques with derivatives and deconvolution that enable a high level of confidence in interpretation results.
It can be safely predicted that the importance of well test analysis in reservoir characterization will continue to increase as new tools such as permanent downhole pressure gauges and downhole flowmeters become more widely used and as the scale relationship with the interpretation of other data from geophysics, geology, and petrophysics becomes better understood.
This article contains highlights of paper SPE 102079 “From Straight Lines to Deconvolution: The Evolution of the State of the Art in Well Test Analysis,” SPEREE (Feb. 2008), 11-1, pp 41–62.