The industry worldwide has been swayed by the digitization wave, with investments targeting improvements in market performance and competitiveness. This article overviews the multi-faceted digital transformation of petroleum engineering from an intradisciplinary perspective, using specific examples from SPE’s seven technical disciplines.
Oilfield Digitization
The oil and gas industry has holistically embarked on a digital transformation which imposes a multifaceted impact on the upstream sector and petroleum engineering. Data have become a new asset for operators and contractors, as valuable as the leases, rigs, and personnel. Acting as a toolbox for visualizing, understanding, and manipulating “big data,” the digital sciences allow engineers to efficiently resolve issues, indiscernible by conventional approaches. The volume, velocity, variety, veracity, and value of data is continuously increasing, pushing for the invention of a new skillset among engineers in learning and being adept to these new digital tools.
Machine learning (ML) and artificial intelligence (AI) are quintessential examples of tools engineers can use to comprehend acquired data and its trends. Both ML and AI operate in a similar fashion. A known data set is assigned as a teaching set with a computer performing a regression analysis to recognize the mathematical functional relationship between the variables. As more data are gathered, the trends exhibited by the aggregate data become clearer and more accurate. These trends are identified and interpreted by subject matter experts (SMEs) whose call is to make intelligent decisions. In the medical field for instance, magnetic resonance imaging (MRI) scans are used to identify tumors. Vast sets of MRI scans with confirmed tumor presence are used as a training set for ML. Thereafter, computers are able to look at any MRI scan and identify even tiny tumors at an early stage of development, enhancing the scan’s accuracy and reliability. An expert physician’s opinion is always taken into account to confirm the findings and arrive at a final conclusion. This procedure has transformed medical diagnostics, helping save many lives.
Linked with big data and statistical sciences, multiple innovations such as the industrial internet of things (IIoT) and automation offer a challenge as well as opportunity to unlock big future values. The application of digitization and cloud computing—colloquially called the “Fourth Industrial Revolution” (Fig. 1)—in the oil and gas industry is often viewed as an evolution of a new discipline. Nevertheless, the impact of this “evolution” is so broad and multifaceted that it has revolutionized almost all sublets of petroleum engineering, expanding each to new fronts. While the problems petroleum engineers are facing remain fundamentally of the same nature (drilling, completions, facilities-related problems, etc.), the solutions in their arsenal have expanded. The rest of this article overviews this digital transformation petroleum engineering is undergoing from an intradisciplinary perspective, considering the seven “SPE Disciplines”: Drilling; Completions; Health, Safety, and Environment; Management and Information; Production and Operations; Projects, Facilities, and Construction; and Reservoir.
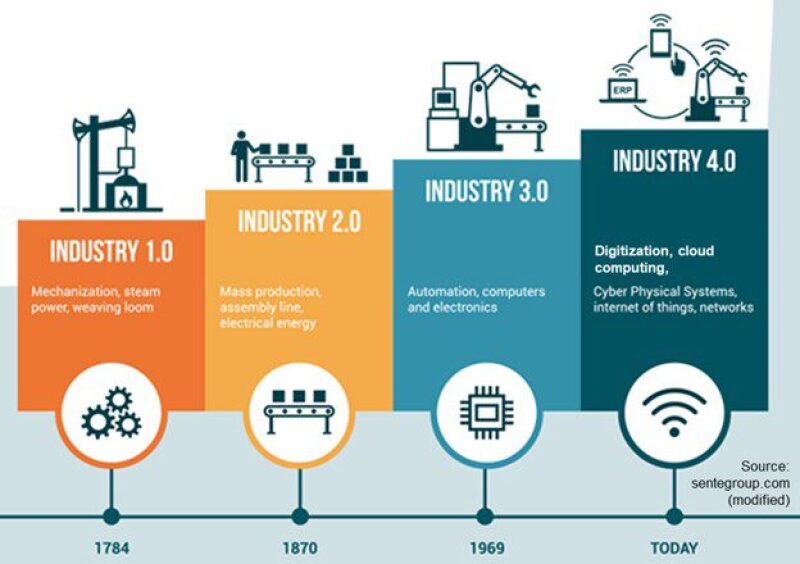
Fig. 1—The four industrial revolutions. Throughout history, certain technologies have revolutionized the industry, changing it forever. Steam power and machines changed manufacturing and business permanently in 1784, as did electricity in the next century, followed by computers in the mid-twentieth century. The fourth revolution is in progress today and involves an end-to-end digital transformation of industrial operations. Source.
Application Examples From SPE’s Seven Technical Disciplines
The oil and gas industry is rapidly evolving toward a digital transformation. Upstream optimization entails, among others, the use of sensors for truck-fleet monitoring, gathering of huge seismic data volumes for subsurface visualization, real-time analytics for rod-pump maintenance, early kick prediction, well selection for matrix acidizing, and drones for remote surveillance and digital imaging of core samples.
DRILLING |
Early Kick Detection. One key safety aspect while drilling is maintaining well control. The conventional method of identifying a kick is to recognize basic indications directly from the wellbore, such as observations of flowback, pit gain, ROP increase, lost circulation, cutting-size-and-shape changes, etc. These kick indications are often missed or misjudged by the driller, which leads to worsening of the situation, increasing the severity of the developing problem. Real-time monitoring and ML algorithms have provided a unique solution to this problem. With appropriate data set training, ML algorithms are able to predict trends in drilling parameters (e.g. circulation rates [RPM], weight-on-the-bit [WOB], etc.—see Fig. 2) and provide early warnings prior to any major conventional kick indication, which can be noted by the driller (Unrau et al., 2017; Yang et al., 2019). This routine offers the rig crew ample time to react appropriately well before the situation goes out of control.
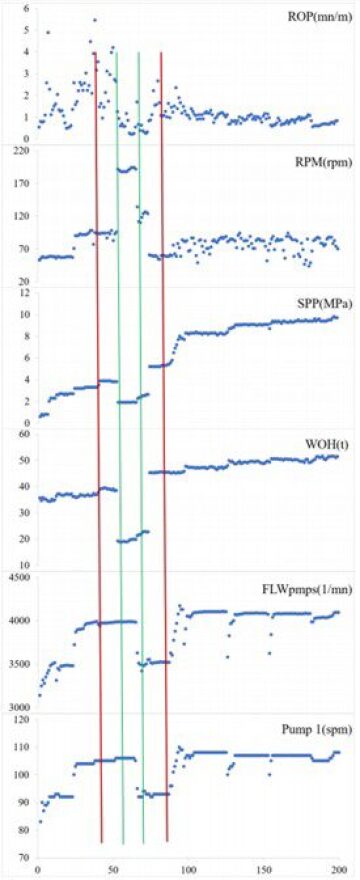
Fig. 2—The red lines bound the data range in which the ML algorithm warns for a possible kick, which is well in advance to the range of the actual kick scenario, bound by the green lines. Source. Yang et al., 2019.
COMPLETIONS |
Dyna-Card Digitizing. Sucker-rod pumps (SRP) are an integral part of well completions, (Fig. 3) assisting in production maintenance via artificial lift. Each sucker-rod pump generates a pump card (also known as dyna-card), providing information on the stroke length (“displacement” on Fig. 3) variation against load.
The dyna-card provides means for interpretation of the current condition of the downhole pump. Completion engineers (or “pumpers”) look at the dyna-cards to decipher the status of the SRPs. Image reading algorithms are developed, employing ML/AI, to provide quick analysis of these dyna-cards. These algorithms help engineers understand, predict, and thus optimize SRP operations. Using dyna-cards, showing eventual SRP failures as training sets, the algorithm is able to identify trends leading to these specific failures (Bangert, 2019; Zhou et al., 2019; Sharaf et al., 2019), ensuring repairs take place prior to failure.
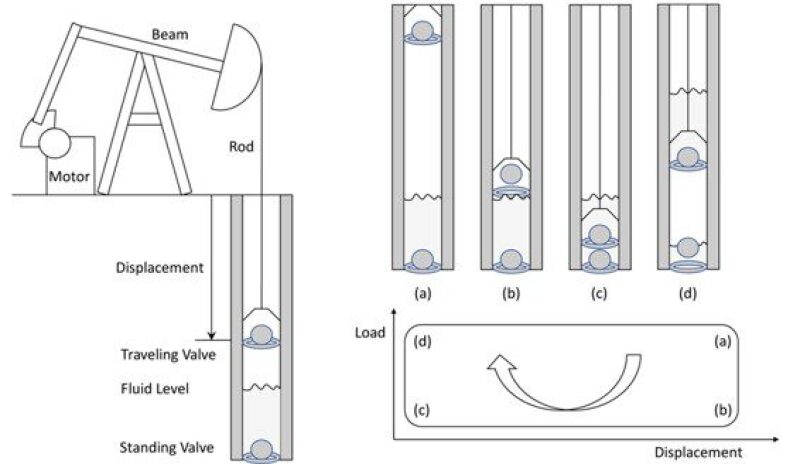
Fig. 3—Schematic of a basic SRP setup (surface and subsurface) on the left and the dyna-card on the right, with the corresponding standing valve/traveling valve configuration. Source: Bangert, 2019.
HEALTH, SAFETY, AND ENVIRONMENT |
Truck Fleet Management Systems. Optimizing the movement of mobile assets and equipment would yield higher return on investment (ROI) rates. A good example is the movement of trucks (Fig. 4) used during hydraulic fracture treatments. A typical fracture treatment requires hundreds of trucks moving to and from the well. Fleet management systems (FMS) provide driver alerts, accident prevention, and vehicle diagnostic monitoring. In addition, FMS can provide a platform where driver performance and safety can be “gamified” so that each driver is scored against his/her peers and tracked on a leaderboard. This will provide an additional motivation to “gamer” employees, and the drivers’ records can be easily monitored by his/her employer to provide a basis for bonuses and future recruitment. In one case study performed on an oilfield services company, FMS implementation led to a 300% improvement in driving safety and a 97% reduction in speeding violations. This translates to savings of $18 million per year for the company (Accenture, 2015).
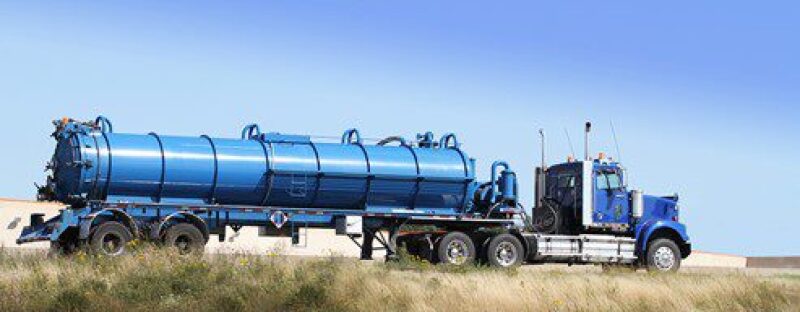
Fig. 4—Truck transferring water to the wellsite for hydraulic fracturing. Source.
MANAGEMENT AND INFORMATION |
Seismic Data Interpretation. During the exploration phase, seismic-data acquisition (DAQ) yields high volumes of structured data (SEG-D, pre-stack, post-stack), velocity (wide azimuth DAQ), veracity (seismic processing), and high variability, which is used for subsurface interpretation. Simulation techniques and advanced geophysics modeling support seismic operations. “Big data” applications enhance seismic-trace identification aiding strategic decision-making. For instance, considering the acquired data which are unstructured in various formats, appropriate data-mining techniques can be applied for SEG-D or SEG-Y format data. Fig. 5 summarizes various data-mining techniques used in oil and gas exploration. As the seismic data are often in standard “SEG-D” (developed for the field) or “SEG-Y” (user-friendly for editing) formats, proper data-mining techniques need to be applied to the unstructured data set.
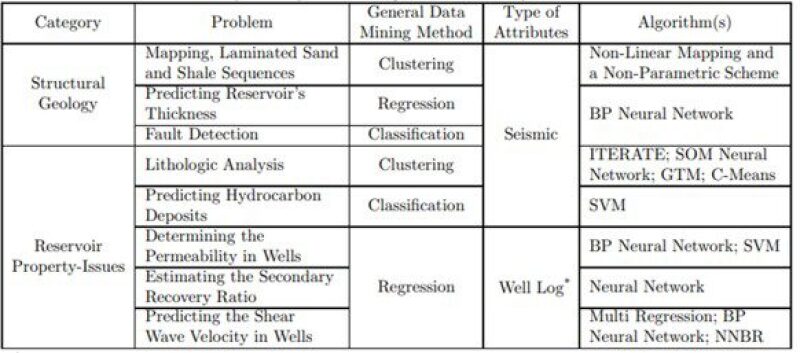
Fig. 5—Data-mining techniques. Source.
Powered by supercomputers, which utilize rapid data processing, digitization, high-speed networking, and high-resolution graphics, visualization centers (Fig. 6) enable the display and manipulation of complex volumes of 3D data, resulting in more-efficient interpretation using more data points in less time.
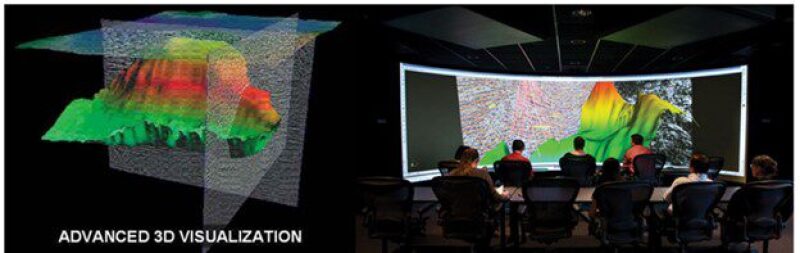
Fig. 6—Modern 3D seismic visualization. Source.
PRODUCTION AND OPERATIONS |
Optimal Subsurface Levers. There is no blanket improved-oil-recovery (IOR) strategy—every oil or gas field needs to be evaluated specifically with the value-added potential identified for the investment holistically. IOR techniques (e.g. waterflooding, EOR, matrix acidizing, etc.) aimed at achieving production optimization focus on four key areas:
- Enhance production from existing and newly drilled wells
- Maximize the economic ultimate recovery (EUR)
- Select the appropriate well intervention based on historical trends
- Maximize the return on investment (ROI)
Illustrating an example of an analytical approach toward maximizing reservoir production, following well performance history analysis, an E&P company decided to implement initiatives covering two levers: well intervention and IOR. After analyzing the subsurface levers (Fig. 7) and if “short-term production increase” methods did not fulfill the expected production levels, the next lever was pulled, which is a “step change in recovery.” A good well intervention example in regard to design and optimization using historical data is well selection criteria for matrix acidizing. Once well-performance history is analyzed, some of the wells which satisfy the criteria set for matrix acidizing are prioritized, targeting maximum improvements in well performance.
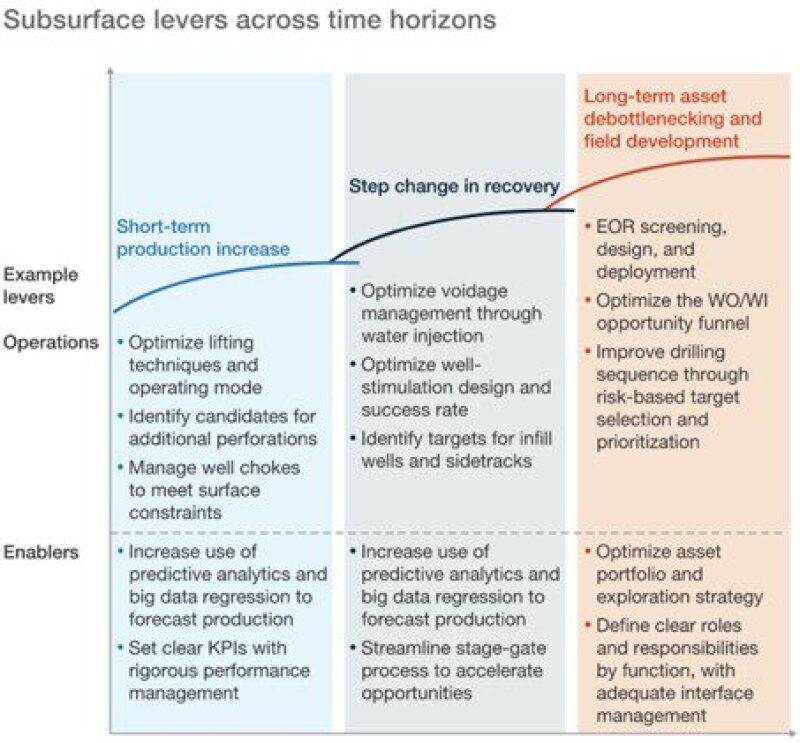
Fig. 7—An analytical approach to maximizing reservoir production. Source.
PROJECTS, FACILITIES, AND CONSTRUCTION |
Remote Monitoring and Smart Pigging. Remote monitoring of facilities uses smart video surveillance. Remote monitoring is employed via inspection applications and enhances facilities’ security and environmental protection by preventing leakages, intrusions, and deformations.
Self-navigating drones and satellites can be used to monitor storage facilities and pipelines from miles away (Accenture, 2015). This enables a facilities engineer to monitor the separators in an east Texas field from the comfort of his/her office in Houston. This reduces the number of personnel necessary for surveillance purposes and hence reduces the operator costs. Additionally, reliable remote monitoring enables reduction in the number of supervisor visits necessary in the oilfield, leading to lower costs.
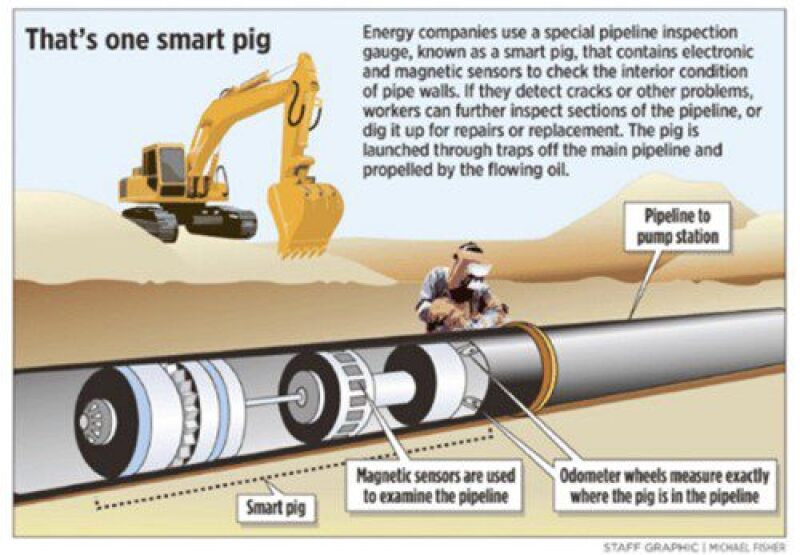
Fig. 8—Smart pig digitally transmitting data from the insides of pipelines to the operators. Source.
Furthermore, “smart pigging” (PIG: pipeline-inspection gauge) allows the pipelines to be cleaned without having to disrupt the flow through the pipeline system. Besides cleaning the pipeline, smart pigs (Fig. 8) inspect the insides of the pipeline to gather necessary data vis-à-vis metal loss, hydrocracking, etc. using non-destructive techniques such as ultrasound (Mittal, 2018).
RESERVOIR |
Digital Imaging. Digital imaging is a technique used to capture either micro-scale images or macro-scale images (outcrop photos). Computerized tomography scanning (CT scan) is used for creating clear, detailed cross-sectional images of core samples (Figs. 9–10), which give insights to the texture and various petrophysical properties of the reservoir studied. A typical example is the estimation of “fractal dimensions,” which describes the fracture distribution observed in the outcrop. This method depends on a CT scanner tracking the fracture distribution in the core sample, and then running a simulation modeling the fracture network in the entire reservoir.
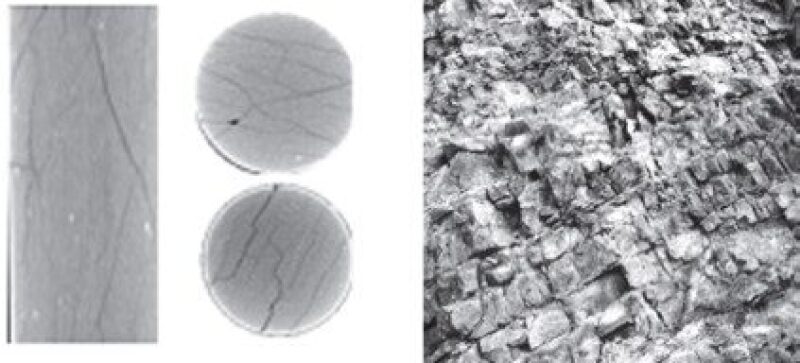
Fig. 9—Digital image output of the CT scan on the left and preprocessing the digital on the right.
The CT scans are processed (often through a MATLAB toolbox), converting the colored image of the rock mass to grayscale. Following that, the main fracture network present in the core sample is determined by digital-image preprocessing using the “histogram-equalization” method. The Canny algorithm is used to detect the edge points, and the fractal dimensions can then be calculated (Basirat et al., 2020).
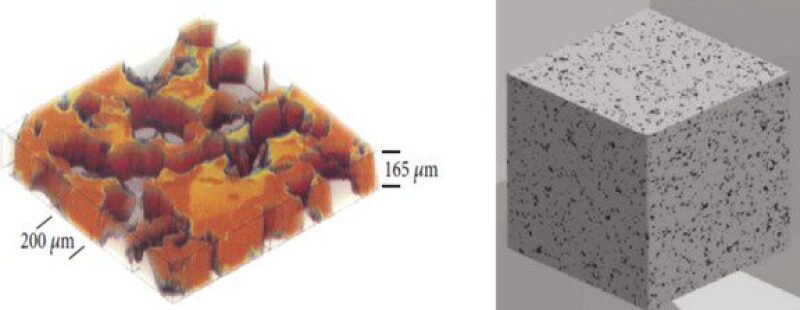
Fig. 10—3D digital core images of the organic pores.
Key Takeaways
- Many oil and gas corporations are looking into adopting digital work ways to enhance their market performance. This requires a new skillset of ability to work with big data utilizing ML and AI.
- The digital revolution impacts petroleum engineering holistically, in an intradisciplinary manner, affecting each of its sublets individually, rather than creating a new sublet. Specific examples from each of the seven SPE Disciplines are presented.
- Petroleum engineers have a new “toolbox” in their arsenal for solving traditional oilfield problems (vis-à-vis drilling, completions, reservoir, etc.). Additionally, the digital transformation offers the potential for answering questions that had remained unanswered for several years or had unfathomable engineering ability.
References
Accenture. Digital Oilfield Outlook Report: Opportunities and Challenges for Digital Oilfield Transformation. Oct. 2015.
Bangert, P. Diagnosing and Predicting Problems with Rod Pumps using Machine Learning, SPE-194993-MS. 2019.
Basirat, R. et al. Scaling Geological Fracture Network from a Micro to a Macro Scale. Frattura Ed Integrità Strutturale, vol. 14, no. 51, pp. 71–80, 2019.
Mittal, A. Remote Sensing—Oil and Gas Pipeline Monitoring. Skymap Global. 1 July 2018.
Sharaf, S. A., Bangert, P., Fardan, M. et al. Beam Pump Dynamometer Card Classification Using Machine Learning. SPE-194949-MS. 2019.
Unrau, S., Torrione, P., and Hibbard, M. Machine-Learning Algorithm Applied to Detection of Well Control Events, SPE-188104-MS. 2017.
Yang J., Sun T., Zhao Y., Borujeni T. A. et al. Advanced, Real-Time Gas Kick Detection Using Machine Learning Technology, Twenty Ninth International Ocean and Polar Engineering Conference, Honolulu, Hawaii. ISBN 978-1 880653 85-2; ISSN 1098-6189. 16–21 June 2019.
Zhou, X., Zhao, C., and Liu, X. Application of CNN Deep Learning to Well Pump Troubleshooting via Power Cards. SPE-197733-MS. 2019.
![]() |
![]() |
![]() |
![]() |
Aman Srivastava is technical adviser, well construction for Halliburton-Landmark. With almost 10 years of experience in on-field and off-field drilling activities, Srivastava holds a special interest in well-construction engineering. He is a reviewer of two peer-reviewed journals in petroleum engineering and holds a patent for his design of internal combustion engines. He earned a BS in mechanical engineering from the National Institute of Technology, India, an MS in petroleum engineering from the University of Oklahoma.
Andreas Michael is a PhD student at Louisiana State University, researching topics related to hydraulic fracturing. He received his BS and MS from the University of Texas at Austin and represented the school internationally at the PetroBowl Championship where he received second place in 2013 and 2015. His professional interests include unconventional reservoirs, petroleum geomechanics, petrophysics, economics, and geopolitics.
Lavish Garg is a formation evaluation engineer at Weatherford in India where he interprets wireline well logs focusing on well integrity issues such as pipe assessment for corrosion and cement problems. His interests encompass real-time drilling data, data analytics, and well-log evaluation. He represented his college at the 2016 PetroBowl in Dubai and is past president of the Maharashtra Institute of Technology (MIT) Pune Society of Petroleum Geophysicists, Student Chapter. Garg holds a BS in petroleum engineering from MIT Pune, India.
Rami Harkouss teaches at Beirut Arab University (BAU) and is the faculty advisor of the SPE BAU chapter. He received the 2019 Student Chapter Excellence Award and won first place in the ADIPEC University program in 2017 and on BAU’s Engineering Projects Day in 2015. Harkouss holds a BE in drilling engineering from the Petroleum University of Technology (Ahwaz-Iran), a master’s degree from École Nationale Supérieure de Chimie de Clermont-Ferrand, and a PhD in process chemical