Like many shale producers these days, Texas Standard Oil is carefully considering its options for acquiring new assets in the Permian Basin. But unlike many, the independant operator is relying on the use of emerging data analytics methods to determine the right price of active oil and gas fields.
“This is the future of asset evaluation,” said Abhishek Gaurav, a completions engineer at Texas Standard who is trying to impress potential investors with an off-the-shelf analytics program he tailored to identify money-making patterns in undervalued shale plays.
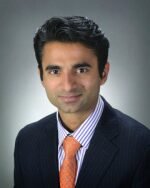
Gaurav explained that any good engineer can manually run the economics for a conventional field where there may be only 10 to 20 wells within a 5-mile radius. When scaled up to shale fields though, especially those in west Texas that have hundreds of wells within that tight radius, this chore “just becomes too massive, too soon,” he said, adding that, “Unless you have the help of data analytics, it is impossible to see or define the opportunity.”
Texas Standard’s acquisition methodology is outlined in a technical paper (SPE 185018) that was presented at the 2017 SPE Canada Unconventional Technical Conference on Thursday, Feb. 16, in Calgary.
Gaurav said the Houston-headquartered firm is trying to get investors to see beyond type curves, a production metric that has been called out for being somewhat misleading when based on short time frames. Holistically-speaking, there are dozens of factors relating to a company’s completion philosophy, local geology, and field economics that may be better predictors of future performance.
To put that puzzle together, Texas Standard uses multi-variable analytics that enables its engineers to quickly pick out what they think is the most important production driver for any study area. This approach is used in other industries, however, Gaurav said he is not aware of any other upstream companies that do this.
Pattern Identification
Texas Standard started with this multi-variable method 18 months ago and a number of interesting trends have been identified through it. One pattern flagged in late 2015 showed that a large operator was implementing a new completion design that Gaurav said today generates wells with consistent initial production rates of about 2,000 BOE—a high-water mark in the shale sector.
In Reeves County—the same county where Apache Corporation announced late last year that it discovered 3 billion bbl in a play called Alpine High—Gaurav noticed that some operators were changing the direction of their wellbores.
“Nobody was interested in this area,” until that shift in strategy came about, he said. Upon a deeper inspection of the underlying data, Gaurav saw that wells oriented a particular way were producing better than other wells despite all being in the same landing zone.
Sifting out the real performance drivers from the perceived ones may also help correct the course for operators that are following the best practices of another operator, only without understanding that they may be applying those practices in the wrong geologic conditions.
Gaurav described the above strategy as simply “copy and paste.” He explained his view: “Even the guy who drives the sand truck from Wisconsin to Texas knows that more proppant is better. And there are areas where you see that 1,000 lbs of sand per sq. foot is just great because the rock is good enough. But we also see that what really differentiates performance is the orientation—where you land the well.”
Aside from well orientation, this acquisition methodology looks at more than a dozen other variables as inputs to determine which are the ultimate influencers of oil and gas production. In some areas, that will be whether the well was drilled toe-up or toe-down. In another, the key performance driver could be reservoir pressure or the gas-to-oil ratio.
Data and Details
The good news for companies interested in using similar data science techniques to appraise other companies’ assets is that the bulk of the relevant information is in the public domain—either through regulator-managed databases or in corporate publications. The latter is especially critical in Texas where some key completion details are not required to be shared publicly, such as the number of fracture stages in a horizontal well.
Where there are gaps in the data, Gaurav said he turns to the information that exploration and production companies volunteer in their quarterly conference calls and investor slides. Often, companies will disclose descriptions of their newest completion techniques in an effort to demonstrate progress to their benefactors.
More missing pieces can be gathered up through oilfield consulting reports. However, Gaurav cautioned against using such information unless it is focused on specific areas since shale formations are notoriously heterogeneous and their defining properties can change from one drilling location to the next.
As this work continues, Gaurav is already working on submitting another technical paper to highlight how the approach has been enhanced using artificial intelligence and machine learning. His aim is to build more automation into the system and be able to complete asset evaluations in just minutes.