Smart completions let engineers intervene with details of wells’ operations from a distance. Smart wells transmit nearly continuous (real-time) data streams (pressure, flow rate, etc.) to the remote office, providing immediate feedback on the consequences of recent decisions made and actions taken. Smart fields include multiple smart wells providing the possibility of managing the entire reservoir remotely and in real time. Our industry is now on the eve of making real-time reservoir management (RTRM) a reality. Artificial intelligence and data mining (AI&DM) is one of the key enabling technologies for RTRM. AI&DM enables us to process, model, and use real-time data streams, build accurate prototypes of sophisticated reservoir-simulation models that can respond to changes in model input in real time to help us make crucial reservoir management decisions and close the loop on high-frequency feedback to the reservoir model for making RTRM a reality.
In short, the contribution of AI&DM technology to smart fields can be summarized in two items: (a) It provides the technological capabilities to automatically and autonomously handle high-frequency data streams received from permanent downhole gauges (data cleansing, data summarization, pattern recognition, adaptive online modeling, etc.); (b) It provides the modeling framework and workflows that allows the existing reservoir-simulation models to be run in real time, thus making RTRM possible.
RTRM
Reservoir management is defined as the practical science of developing a hydrocarbon field in a manner that maximizes ultimate recovery. RTRM involves performing reservoir engineering analysis in real time to support frequent field-management decisions, and using near-real-time feedback on the consequences from the reservoir/well to assess management decisions and reservoir performance. Therefore, RTRM is the enabling technology for the emerging smart fields. It is the closed-loop process during which the reservoir model is continuously updated by the information/feedback received from the field (by means of high-frequency data streams) that are the consequence of the decisions made and implemented, based on the reservoir model.
The ultimate benefit of the smart field depends on the degree of our success in building and implementing RTRM. In other words, the value of high-frequency data streams is realized once we are able to use them in effectively updating the reservoir model and subsequently using the model to make field-operational decisions. Therefore, the key to moving toward successful smart-field operation is to be able to perform the following steps:
1. Acquire, process, and analyze real-time, continuous data streams from the wells.
These real-time pressure and rate data provide indications of reservoir reaction to the operational decisions made by engineers using the reservoir model.
2. Use the real-time data as feedback to the reservoir model.
By analyzing the high-frequency data in the context of the reservoir model, engineers can compare the actual reservoir/well response with the model predictions. Furthermore, the model can be updated (modified) to compensate for the discrepancies between its predictions and reservoir/well responses.
3. Make operational decisions based upon model simulations.
Make new operational decisions by comprehensively analyzing the solution space of the reservoir model and make predictions on possible response from the reservoir/well. Implement the decisions. (Among the possible decisions is to continue operations as is.)
4. Go to Step No. 1.
Perform all these analyses while taking into account and quantifying the uncertainties associated with the reservoir model.
To accomplish steps 2 and 3, the reservoir model must have the capability of analyzing multiple scenarios in real time (or near real-time) and provide real-time responses to changes to the model input or potential modifications that can be made to the well operation. The reservoir/well responses to the modifications are reflected in high-frequency (real-time) data streams. Fig. 1 is a schematic diagram of the closed-loop RTRM concept.
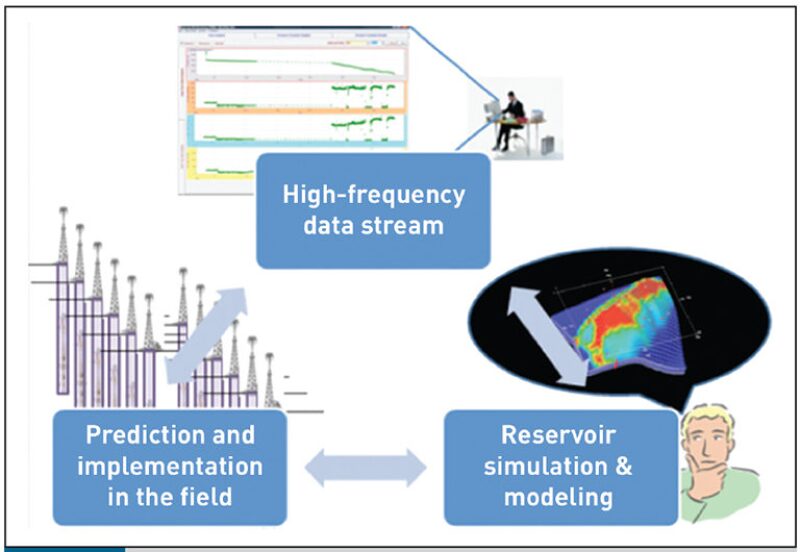
Surrogate Reservoir Model
The surrogate reservoir model (SRM) has been developed in response to the need for RTRM and to make it a reality (Mohaghegh et al. 2009; Mohaghegh et al. 2006a; Mohaghegh 2006; Mohaghegh et al. 2006b). An SRM is developed using state-of-the-art capabilities in AI&DM. AI&DM is a collection of complementary analytical tools that attempt to mimic life when solving nonlinear, complex, and dynamic problems. AI&DM consists of, but is not limited to, analytical techniques such as artificial neural networks (Mohaghegh 2000a), genetic optimization (Mohaghegh 2000b), and fuzzy logic (Mohaghegh 2000c).
An SRM is an accurate replica of a complex reservoir-simulation model that may include tens or hundreds of wells. SRM runs provide results, such as wells’ pressure and production profiles or pressure and saturation distribution throughout the reservoir, in real time
The SRM is developed using a unique series of data-generation, manipulation, compilation, and management techniques. These techniques are designed to take maximum advantage of characteristics of artificial neural networks complemented with fuzzy set theory. Upon completion of the modeling process and validation, an SRM can accurately replicate the results generated by highly sophisticated reservoir-simulation models in response to changes made to the model input, in fractions of a second. The fact that thousands of SRM runs can be performed in seconds makes uncertainty analysis a practical task. This allows the uncertainty band associated with any decisions to be identified quickly.
The SRM has been successfully field tested. In a recent study performed on a giant oil field in the Middle East, an SRM was developed to replicate the existing simulation model of the field that was developed using a commercial simulator. Consisting of approximately a million grid blocks, the computing time required for a single run of the existing simulation model is 10 hours on a cluster of 12 parallel central processing units. Upon development, calibration, and validation of the SRM that could successfully and accurately replicate the results of the simulation model, tens of millions of SRM runs were performed to comprehensively explore the reservoir model’s solution space so that a successful field-development strategy could be established. The objective was to increase oil production from the field by relaxing the rate restriction imposed on wells. The key was to identify those wells that would not suffer from high water cuts once a rate-relaxation program was initiated. The SRM had to take into account and quantify the uncertainties associated with the geological model, while accomplishing the objectives of this project.
Upon completion of tens of millions of SRM runs (equivalent to tens of millions of simulation runs) the 165 wells in the field were divided into five clusters, on the basis of rising water-cut risk. Recommendations for rate relaxation were made for wells in clusters 1 and 2. Furthermore, it was predicted that these wells would produce small amounts of water and large amounts of incremental oil over the next 25 years. On the other hand, more than 100 wells in clusters 4 and 5 were predicted to produce large amounts of water, if rate restrictions were lifted.
With completion of the study, rate restriction was lifted from 20 wells. These wells were selected from among all the clusters to provide a representative spatial distribution of the reservoir and examine the accuracy of the SRM predictions. After more than 2½ years of production, the results were analyzed. As can be seen clearly from Fig. 2 (similar results observed from all other wells in the corresponding clusters are summarized in Fig. 3), wells in clusters 1 and 2 produced large amounts of incremental oil, while water production declined. The opposite effect was observed in wells that were classified in clusters 4 and 5, as predicted by the SRM. Fig. 3 shows the maximum incremental water cut normalized for all wells in each of the clusters. It is clear from this figure that in accordance with the SRM’s predictions, water cut decreased in wells classified in clusters 1 and 2 while it increased significantly in wells classified in clusters 4 and 5.
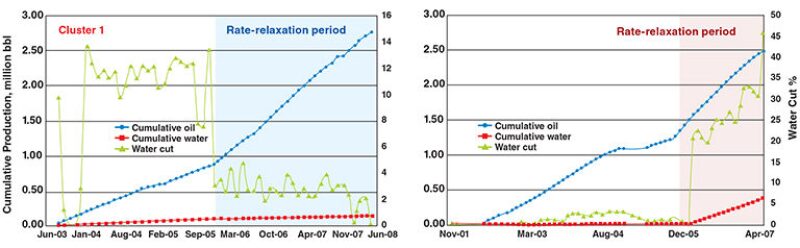
Results from this study, as well as other similar studies, demonstrate the robustness of SRM technology. An SRM can be used to develop replicas of sophisticated and large reservoir-simulation models that then can be used to drive the main engine of RTRM.
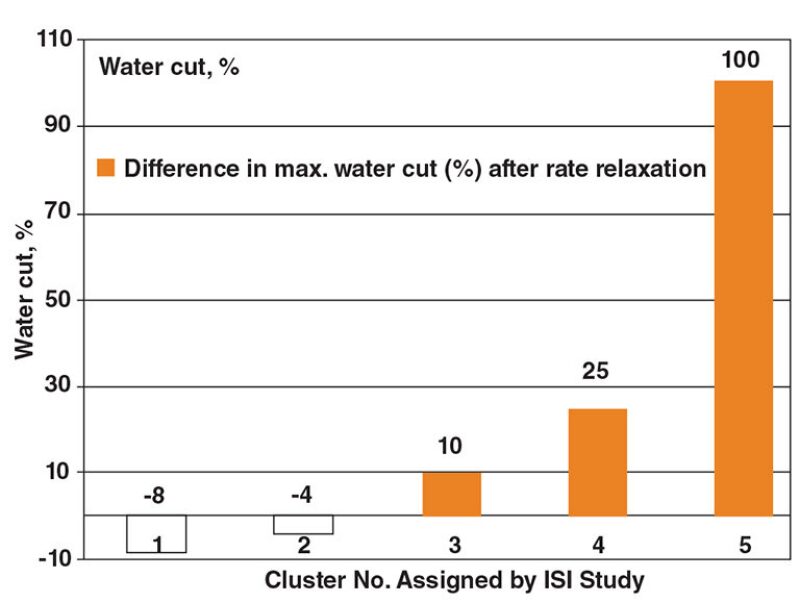
Intelligent Real-Time Data Analysis
The high-frequency (real-time) data that is collected from permanent downhole gauges and transmitted to be stored in data historians is usually unusable in its raw form. It needs to be cleansed and summarized and prepared (processed) for use in reservoir-engineering studies. The high-frequency data need to be denoised, the outliers must be identified and removed, existing trends and patterns need to be identified, and data need to be summarized so that maximum useful information can be preserved, while using the least amount of data storage. Most important, all these tasks need to be performed reliably in real time (at the same speed, or faster, than data are received) automatically and autonomously, without the supervision of an engineer.
Furthermore, the intelligent real-time data analyzer needs to be capable of taking maximum advantage of the information content of the high-frequency data. AI&DM-based intelligent data analysis of the high-frequency data streams includes two main components: high-frequency data management (HFDM) and high-frequency data analysis (HFDA). HFDM performs (autonomously and in real time) data preparations and preprocessing. This process prepares the data for reservoir-engineering analysis and includes data denoising, outlier removal, pattern recognition and summarization, and, finally, data preparation for SRM.
HFDA performs state-of-the-art reservoir-engineering analysis using the prepared and preprocessed high-frequency data streams. The analyses include
- Detection, isolation, and analysis of pressure-transient data used for continuous monitoring of well and reservoir behavior
- Empirical modeling using adaptive technology that learns data behavior and continuously modifies itself to match and model the observed data, to predict thier behavior and detect, model, and verify hypotheses about drive mechanisms by means of continuously modeling volumetric and material-balance estimates
- Diagnostic analysis to detect interwell connectivity and reservoir compartmentalization that may be present
- Communication with the SRM (sending feedback and receiving instructions)
Fig. 4 shows an example of real-time data preprocessing and summarization (left) and real-time adaptive modeling (right) applied to a well in the Middle East.
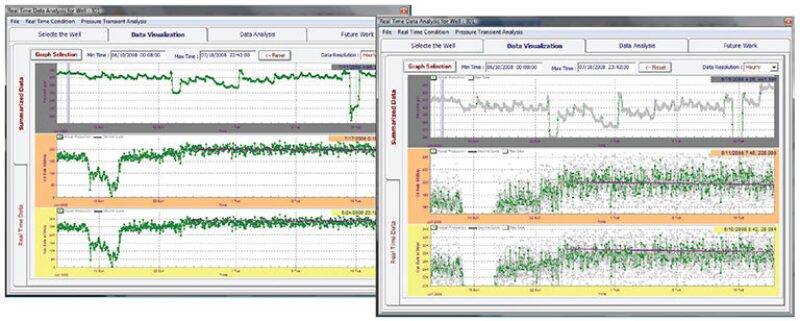
Conclusion
AI&DM contributes to all aspects of the E&P industry to help increase efficiency and productivity (Tapias et al. 2001; Saputelli et al. 2002; Zangl and Oberwinkler 2004; Finol and Buitrago 2002; deJonge and Stundner 2002). AI&DM provides a key component of the emerging smart field. At a June 2009 SPE forum on this topic in Colorado Springs, Colorado, AI&DM’s contributions to drilling, production, reservoir characterization, reservoir modeling, reservoir management, and asset-portfolio management were thoroughly discussed by numerous industry leaders. All agreed that the role of AI&DM in the E&P industry can only increase as more and more applications are implemented. It is hard to imagine that smart fields can be implemented without extensive use of AI&DM as an integral part of a complete system that also would include many other components and technologies. Therefore, including the fundamentals and applications of AI&DM in the training of our workforce (either prehiring through the universities or post-hiring through short courses) can contribute to higher efficiency and more productivity. TWA
References
deJonge, G.J. and Stundner, M. 2002. How Routine Reservoir Surveillance with Neural Networks and Simplified Reservoir Models Can Convert Data Into Information. Paper SPE 78334-MS presented at the European Petroleum Conference, Aberdeen, 29–31 October. doi: 10.2118/78334-MS.
Finol, J. and Buitrago, S. 2002. Model Identification with Fuzzy-Optimization Techniques in Geological Data Mining. Paper SPE 78340-MS presented at the European Petroleum Conference, Aberdeen, 29–31 October. doi: 10.2118/78340-MS.
Mohaghegh, S.D. 2000a. Virtual Intelligence Applications in Petroleum Engineering: Part 1—Artificial Neural Networks. J. Pet. Tech. 52(9): 64–73. SPE 101474-MS.
Mohaghegh, S.D. 2000b. Virtual Intelligence Applications in Petroleum Engineering: Part 2—Evolutionary Computing. J. Pet. Tech. 52(10): 40–46. SPE-61925-MS.
Mohaghegh, S.D. 2000c. Virtual Intelligence Applications in Petroleum Engineering: Part 3—Fuzzy Logic. J. Pet. Tech. 52(11): 82–87. SPE-62415-MS.
Mohaghegh, S.D. 2006. Quantifying Uncertainties Associated With Reservoir Simulation Studies Using Surrogate Reservoir Models. Proc., SPE Annual Technical Conference and Exhibition, San Antonio, Texas, 24–27 September. SPE 102492-MS. doi: 10.2118/102492-MS.
Mohaghegh, S.D., Hafez, H., Gaskari, R., Haajizadeh, M., and Kenawy, M. 2006b. Uncertainty Analysis of a Giant Oil Field in the Middle East Using Surrogate Reservoir Model. Proc., Abu Dhabi International Petroleum Exhibition and Conference, Abu Dhabi, 5–8 November. SPE 101474-MS. doi: 10.2118/101474-MS.
Mohaghegh, S.D., Modavi, A., Hafez, H.H., Haajizadeh, M., Kenawy, M., and Guruswamy, S. 2006a. Development of Surrogate Reservoir Models (SRM) For Fast-Track Analysis of Complex Reservoirs. Proc., SPE Intelligent Energy Conference and Exhibition, Amsterdam 11–13 April. SPE 99667-MS. doi: 10.2118/99667-MS.
Mohaghegh, S.D., Modavi, A., Hafez, H., Haajizadeh, M., and Guruswamy, S. 2009. Development of Surrogate Reservoir Model (SRM) for Fast-Track Analysis of a Complex Reservoir. Intl. J. Oil, Gas and Coal Tech. 2(1): 2–23. doi: 10.1504/IJOGCT.2009.023627.
Saputelli, L., Malki, H., Canelon, J., and Nikolaou, M. 2002. A Critical Overview of Artificial Neural Network Applications in the Context of Continuous Oil Field Optimization. Paper SPE 77703-MS presented at the SPE Annual Technical Conference and Exhibition, San Antonio, Texas, 29 September–2 October. doi: 10.2118/77703-MS.
Tapias, O., Soto, C.P., Sandoval, J., Perez, H.H., and Bejarano, A. 2001. Reservoir and Artificial Intelligence Techniques for Data Analysis. Paper SPE 68743-MS presented at the SPE Asia Pacific Oil and Gas Conference and Exhibition, Jakarta, 17-19 April. doi: 10.2118/68743-MS.
Zangl, G., and Oberwinkler, C.P. 2004. Predictive Data-Mining Techniques for Production Optimization. Paper SPE 90372-MS presented at the SPE Annual Technical Conference and Exhibition, Houston, 26–29 September. doi: 10.2118/90372-MS.