Cost estimation for facilities depends on early concept selection and critical inputs, often complicated by uncertainty in one or more of the critical inputs. Empirical cost models and cost modeling methods using these inputs vary in degrees of scope, comprehensiveness, and robustness.
Cost modeling is critical in developing project economics to determine if a project is a sound investment. Its importance extends from concept comparison and selection to use in planning and optimization of field development, and to benchmarking of projected and actual costs.
Although idiosyncrasies in projects and their required facilities drive cost estimations, differences in organizational capabilities further affect the validity and reliability of these estimations.
This article will examine the industry’s approach to cost estimation, highlighting challenges and sources of error, and then discuss models specific to the Arctic and offshore Gulf of Mexico (GOM) regions.
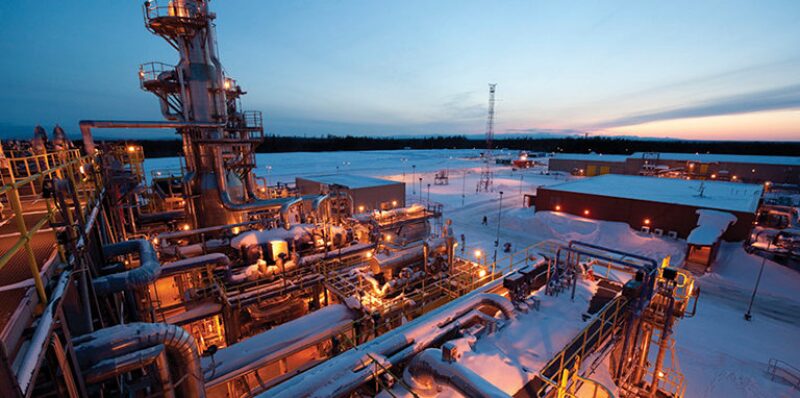
Definition of Project Scope
A thorough understanding of the asset development, inclusive of the facilities, reservoir and well construction scopes, is often lacking in the cost estimation process, said Neeraj Nandurdikar, manager of exploration and production practice at Independent Project Analysis (IPA). Each component, if not assessed and incorporated correctly, can lead to less robust cost estimates.
For example, if the reservoir appraisal is not fully complete (or not complete at the right time vis-à-vis facilities definitional work) in a gas project, leaving uncertain the amount of impurities such as hydrogen sulfide or carbon dioxide, the understanding of the scope of facilities is incomplete and will adversely affect its cost estimate. Without adequate subsurface knowledge, the need and planning for injection becomes uncertain, or the capacity for injection becomes incorrectly modeled.
Consequently, the unavailable information will affect the required space, weight, and pumping capacity, and the related topside equipment and facilities, and these again have a direct impact on the accuracy of the cost estimate.
Shortfalls in timely functional cooperation may also contribute to error in estimations, Nandurdikar said. Although each function is doing its job, the time line for each is not optimally aligned for inclusion in the estimation process. Especially today, equipment must be ordered with significant lead time, thus driving early, and often premature, decision points. For example, one function may be running ahead and making its decisions, however, it is not reflecting the latest understanding of the asset. As a result, the cost estimate may be locked in before a comprehensive assessment of the project could be completed, thus missing critical elements of scope.
Although functional cooperation is a significant source of error, Nandurdikar said, “IPA has been talking to its clients about it for the last five to seven years, and rarely sees much progress to alter this. Even when you look across the industry, you have facilities, reservoir, and well disciplines, but there is no asset discipline.”
Value of Lessons Learned in Estimation
The quality of companies’ databases of lessons learned that are used in cost estimations also varies greatly. Although the databases generally contain volumes of technical data, they are often unmanageable because of the sheer quantity of data. “When there is too much detail, the significant nugget, or ‘ah-ha’ moment is unidentifiable,” Nandurdikar said.
Another caution for using costs from previous construction of facilities is to be certain the conditions of and context for the historical number are fully understood. A poor definition of scope or the prevailing market conditions may have resulted in higher quotes. In such situations, blindly carrying forward project costs may introduce significant errors and perpetuate the higher estimates.
Bill Barger, president of Advanced Project Solutions, commented on the difficulty in predicting costs of components and services three to five years in advance of the time of need: “The offshore industry does not function as a completely free market system. There are a limited number of suppliers and vendors for some services, for example, heavy lift vessels. Therefore, the mobilization fees and rates for these vessels fluctuate greatly.”
Another aspect of cost estimation, Barger said, is the understanding of the types of risks that could affect a facilities project and including them in contingency planning and related costs. “The industry at large has limited organizational abilities, a limited pool of comparables to assess the reasonableness of our cost estimates, and overly narrow perspectives of what risks could actually affect our projects, and therefore contribute to a large cost overrun,” he said.
Shortfalls in Available Talent
“Some companies do these things better than others. What separates those companies that succeed in cost estimations with good results have good cost engineering capabilities, as compared to cost estimating capabilities,” said Nandurdikar. Cost engineering goes beyond the basic factoring in of variables, such as tons of steel, labor rate, and productivity, to evaluate the facilities within the broader context of scope and execution strategy.
On the industry’s dearth of appreciation for cost engineering, Barger said, “there is very little estimation talent in the industry, making it difficult to come up with a valid cost estimate when you do not have experts in that area. There has been a gradual progression of downsizing in this discipline over the last three decades.”
He pointed to another limitation in expertise: “Time is money, and there is a linkage of scheduling and cost. There is limited scheduling talent in the industry. The only functions less appreciated than cost engineers are planners and schedulers.”
Much of the new engineering talent entering the industry is focused on the development of technology, instead of cost engineering, scheduling or planning, he added.
Bill Lamport, senior vice president at GL Noble Denton, noted another significant factor in cost estimation. “Labor and talent are in short supply as major projects pick up around the world. The combination of an aging workforce and a downtick in hiring over the last 15 years has led to a shortage. Labor costs have grown and continue to do so,” he said.
IHS reported that costs for upstream construction labor and engineering, and project management posted strong gains, 9% and 6%, respectively, from the third quarter of 2010 to the first quarter of 2011, largely as a result of a tightening pool of talent and the competition to hire and retain personnel (“Costs of Building and Operating Upstream Oil and Gas Facilities Trending Upwards,” IHS, June 2011).
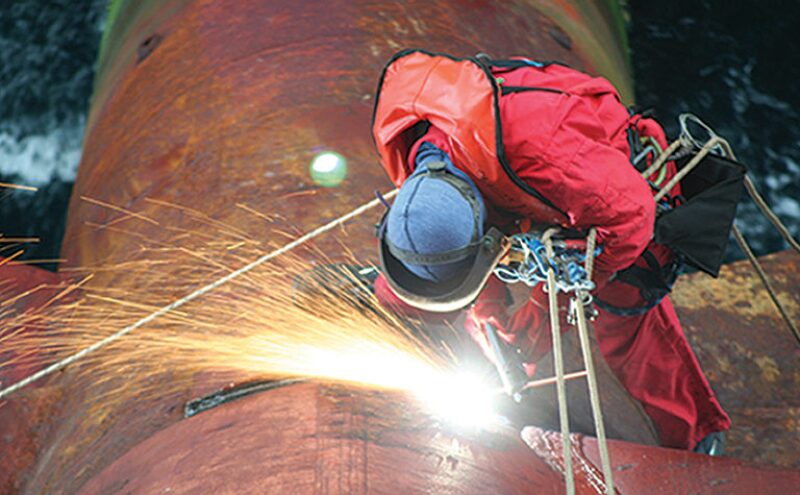
Development of Arctic Cost Models
Generally, the offshore GOM and Arctic regions do not present dramatically different issues in cost estimations, said Barger. One exception that he noted is the comparatively smaller databases of historical project information in the Arctic that project teams and facilities groups can actually use in terms of cost estimations. The GOM has significant repeatability in terms of large existing databases that can be drawn upon for comparisons.
Lamport added that facilities costs in the Arctic are generally higher because of equipment and process requirements related to environmental issues, material and transportation issues, and short work seasons as a result of weather conditions.
Kuznetsov et al. (2011) addressed methods for cost estimation in Arctic offshore oil projects developed by Rosneft Oil, noting that for most Russian offshore fields, existing historical databases are unavailable, and there are no analogous projects that can be used for estimating Arctic costs. Although there are several current projects on Sakhalin Island, they are located in the sub-Arctic region. Unlike the Russian Arctic, the developed North Sea projects have no multiyear ice and icebergs, therefore, they could not be used for Arctic cost estimations.
Most of the software programs for capital cost estimations are designed for evaluation of facilities projects in the “typical” regions of the world and include classifications of equipment and materials from western producers, according to Kuznetsov. These programs’ calculations are derived using databases of existing offshore projects, located predominantly in the offshore GOM and the North Sea.
He and his co-authors wanted to find simple aggregative dependencies to use in the development of proxy models that are applicable to preliminary conceptual studies in facilities in the Russian Arctic region.
They began with the premise that the cost of building gravity base structures (GBS) is calculated as the sum of unit costs multiplied by the weight of constituent materials. In the simplest case, the total cost of the reinforced concrete structure is determined by the average cost per unit volume of concrete laid in the foundation. The value varies with the complexity of the design.
Beginning with a simple cost model for estimation of a GBS concrete volume, the cost/weight of the jacket in sub-Arctic regions is measured by the correlation of the water depth and the topside mass. The authors performed a cluster analysis of concrete gravity base volumes for selected fields to identify “typical” and nontypical groups. An average model was then built to evaluate the fields’ deviation from the average volume of GBS concrete. A linear regression was done on the modeled GBS volume of concrete vs. actual, and coefficients were calibrated by the “typical” data points within the 15% range.
The resultant model was applicable to the Sakhalin Island region and a number of shallow fields (less than 100 m in depth) in the North Sea.
Another regression GBS model was built for the Russian Arctic region. The model was based on several engineering solutions for Arctic GBS developed by design engineering bureaus. It is shown that in the Arctic regions where ice loads are the main challenge, the mass of a topside facility is not a statistically significant factor.
Models for cost estimation of topside facilities were also developed. A group of Norwegian sector topside projects were selected, for which the following parameters were known: type of production platform, topside blocks, basic design data (peak oil and gas production rates), and capital costs. A model of mass regression was built, using the plateau-level oil and gas ratios as independent factors. Correlations for different regions—United Kingdom, Denmark, and Norway—were built, and a cluster analysis was performed.
Differences were clearly shown in the unit costs of topside facilities. Denmark and the UK were similar at the same magnitude of peak oil ratio and peak gas ratio, while Norway’s capital costs were higher. The Sakhalin Island fields clustered together with Norwegian projects, thereby indicating that the model used for Norway can be used in cost estimations for Sakhalin Island projects.
Empirical Cost Models for the Gulf of Mexico
Compared with the limited availability of comparable data for the development of Arctic cost models, the offshore GOM region has a more extensive history of facility construction and installation projects. However, as noted by C.J. Jablonowski and A. Strachan (2008), the offshore facility literature offers little detail on empirical cost models or cost modeling methods. They believe the primary causes for this gap are the proprietary nature of the cost and technical data, and the potential complexity of statistical analysis and specification of regression models.
Jablonowski and Strachan estimated cost functions for tension leg platforms (TLP) and spars using data from 24 completed offshore GOM projects. All spars and TLP in the data set were installed between 1986 and 2006. Costs were adjusted to constant 2006 US dollars and were assumed to occur in equal amounts between the start and end of each project development. Total project cost of the production facility, subsea systems, and initial development wells was examined.
Models of production facility costs generate estimates for total project costs and for the marginal effects of specific project attributes. For example, the marginal cost of 1,000 BOPD of oil capacity on a spar is estimated to cost about $6.5 million; on a TLP, the same capacity costs about $1.3 million. The marginal cost of gas capacity is similar for each facility. Each additional well slot on a spar costs about $26 million; on a TLP about $10 million. An integrated drilling rig on a TLP adds $164 million. Tables 1 and 2 show summaries of spar and TLP data used in the study. Fig. 1 shows the distribution of spar and TLP projects by water depth and reserves.
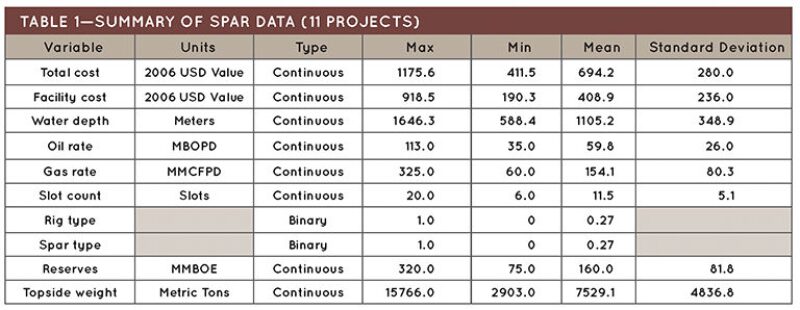
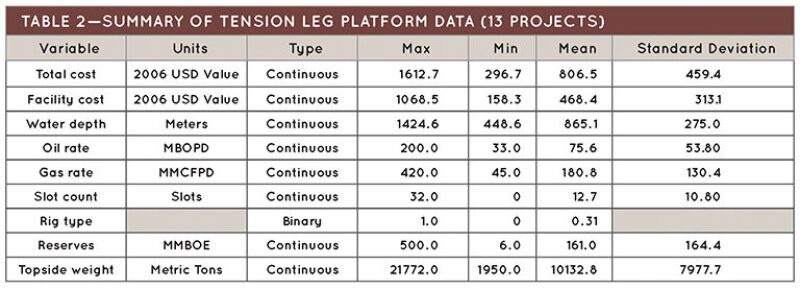
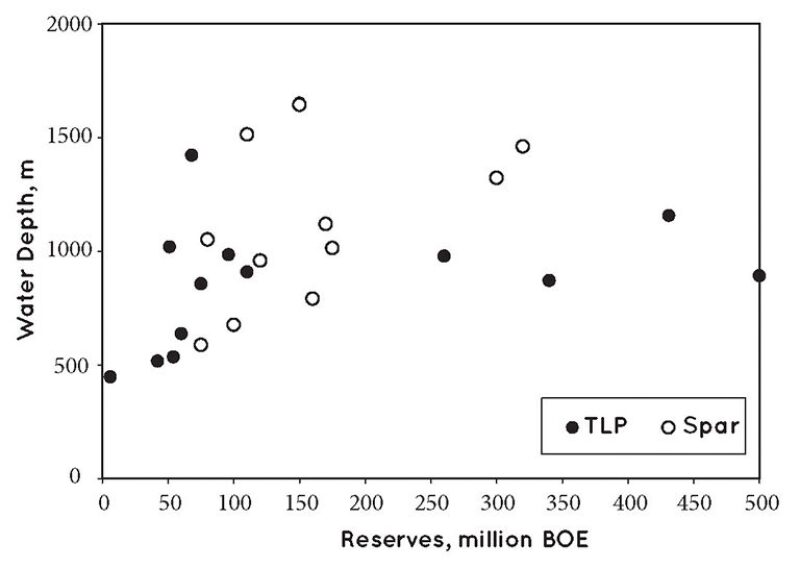
The authors cautioned that the costs are provided as 2006 US dollars. From 2006 to mid-2008, there was an estimated 16% increase in the index used for cost adjustment. Additional adjustment would be required to account for more recent increases in costs.
For Further Reading
SPE 134678 “Modeling Facility Expansion Options Under Uncertainty” by C. Jablonowski, et al.
SPE 115483 “Empirical Cost Models for TLPs and Spars” by C.J. Jablonowsi, et al.
SPE 149916 “Capital Costs Estimation Method for Arctic Offshore Oil Projects” by Mikhail Kuznetsov, et al.