Analytics and artificial intelligence (AI) will disrupt our industry as we know it. The question is not “if” but “when and by whom.” As current sources of competitive advantage erode and new ones emerge, perhaps the only constant in a highly uncertain world is the criticality of good talent for success. Upskilling the workforce for analytics, therefore, needs to be a one of the top priorities as we sail unchartered waters.
One of the key bottlenecks in the oil and gas industry, like in some other mature industries, is a talent shortage in the analytics domain. In our view, the long-term sustainable talent model is a two-pronged approach of not just hiring newly minted data scientists but also developing citizen data scientists—that is, arming functional experts such as engineers with working analytics skills. Because a large percentage of the oil and gas workforce comes from quantitively oriented STEM (science, technology, engineering, and mathematics) fields, upskilling them on analytics likely is a more pragmatic option with a quicker time to value.
What is needed to achieve this ambition, however, is a clear strategy, plan, and sponsorship from leadership coupled with enthusiasm and willingness to learn from employees.
Why Analytics Is Key to the Future of the Oil and Gas Industry?
There is no better industry to leverage AI and machine learning (ML) than oil and gas, and this likely is the best kept secret within the industry. The potential to unlock trapped value is significant across the exploration and production (E&P) value chain for three key reasons.
- An incredible amount of subsurface and surface data is collected every minute, but only a small fraction is used to drive (integrated) E&P decisions.
- Subsurface problems have always been known to be extremely complex, and the oil and gas industry was one of the first to leverage supercomputers. Today, tremendous value is being discovered in connecting surface and commercial objective functions to the reservoir (subsurface) variables.
- A lot of disparate and disjointed data sources and associated databases capture data for rock properties, well costs, supply chain, operations, maintenance, finance, and human resources, for example. When connected prudently and adequately, these granular data enable decision-making that is prompt and reliable.
Since the last downturn in 2014, the oil and gas industry has struggled with lowering the cost of supply and reducing the long cycle times (latency) that have characterized E&P projects. AI/ML has the potential to upend both of these problems for the industry. The cost of supply can be reduced by 30–50%, and cycle times can be reduced by multiple years when advanced analytics is successfully leveraged in E&P decision-making. Fig. 1 shows the key capabilities that can be enabled through analytics.
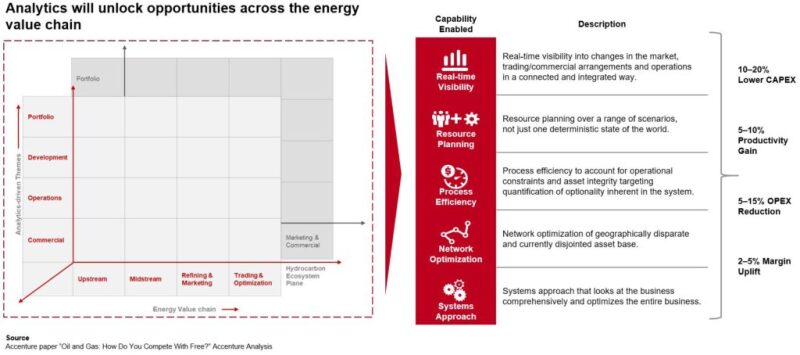
In the new analytics-driven operating model, not only can a significant fraction of the work be automated or digitally enhanced on the back of analytics and AI/ML, but also these enhancements can make the value-adding activities more targeted and effective. Fig. 2 illustrates how the high-level activities of a production engineer can be automated or augmented by analytics and AI/ML in the new operating model. Research shows that 50% of a worker’s time is augmentable using current intelligent technologies and 20% of core processes can be automated; operators can redistribute work to improve productivity, reduce nonworking money, and create jobs that would be more appealing for today’s graduates.
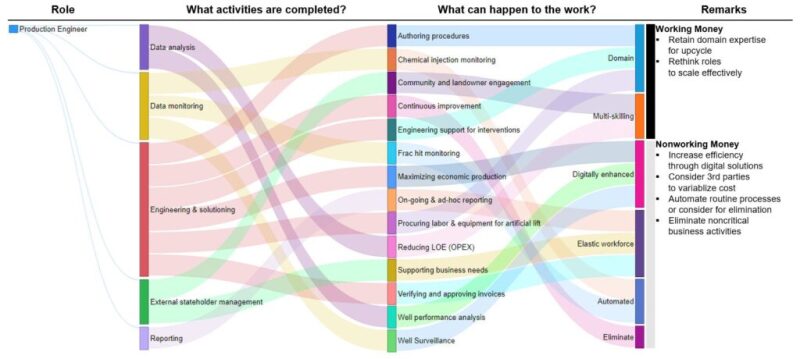
For example, production engineers are faced with vast amounts of data collected for each well (related to both cost and productivity), which they must process, analyze, and synthesize to derive meaningful insights regarding well performance to allow them to focus on the most-economic barrels. These data range from fixed and variable operational-expenditure (OPEX) items to hydrocarbon and water production. Advanced analytics can be leveraged not only to minimize OPEX (e.g., network optimization for supply-chain opportunities such as water hauling) but also to maximize well productivity through proactive well intervention (e.g., through the use of production chemicals or artificial-lift optimization).
These tasks could be daunting and are often performed poorly when AI/ML is not adequately leveraged, leading to suboptimal half-cycle margins for the production systems or fields. We have demonstrated that, by leveraging advanced analytics, production engineers not only can automate much of the data processing and analyses but also can systematically eliminate negative-margin wells/barrels and focus on the highest-value barrels. This approach can lead to a significant half-cycle margin boost of approximately 15–20% based on OPEX savings alone.
What Does Analytics Entail, and What Factors Make for Successful Execution?
Data science, engineering, and analytics professionals wanting to make dramatic impact with their skills and knowledge should look no further. What better industry than the energy industry, which is so fundamental to the growth and prosperity of humankind, to unleash the full potential of advanced analytics. The secret is getting out, and the oil and gas industry is increasingly attracting very good data-science and analytics talent that will shape the future of the industry for years to come.
Solving these complex problems and scaling the solutions throughout the company, however, are two very different things. Scaling analytics is inherently a multidimensional problem, requiring not only the right skill mix but also changes to leadership, culture, and governance. Organizations that drive transformational value from analytics do so primarily because all levels constantly ask the questions, “Can data help my team make better decisions and improve profitability?” and “What is holding us back from leveraging the data and associated analytics?” Weaving an analytics mindset into the organizational DNA involves fundamental behavioral change by addressing the factors illustrated in Fig 3.
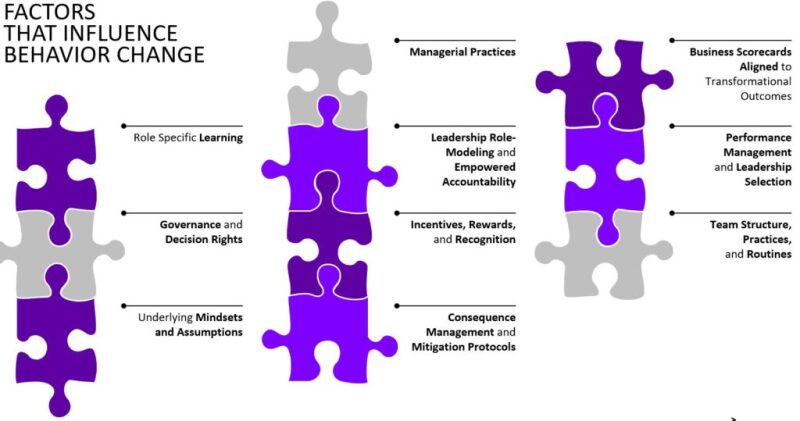
required to adopt an analytics mindset.
How Should Leadership and the Workforce Approach Upskilling for Analytics?
Imperatives for leadership to upskill talent in the analytics space, effectively creating the citizen data scientist, include the following:
Understand how the work will change. Analytics programs typically start with two questions: “How can we transform the business through analytics?” and “As a result, how will the work change for my teams?” Aligning on these inquiries identifies not only the required skills but also the changes to leadership and culture required to adopt the eventual analytics solutions.
Define the analytics competencies that are needed to make this vision a reality. The field of data analytics covers a broad range of competencies. Upskilling talent across all these domains can be time-consuming and expensive. During this step, leadership should align quickly on which competencies are needed to achieve the vision and which roles should be upskilled.
Leverage the broader ecosystem to train staff; don’t recreate the wheel. Oil and gas operators have a range of internal capabilities that already provide training on some of the competencies. Rather than building custom in-house training programs, operators should leverage the wider ecosystem of learning providers—such as universities, startups, or companies dedicated to data-science training—to deliver training cost-effectively while keeping pace with the speed at which technologies and tools change in the domain.
Create an environment that allows analytics to flourish. Many analytics initiatives (including upskilling) lose momentum after initial sponsorship from leadership. For analytics to flourish, leadership needs to be a continuous champion of the priority that analytics has within the organization. Examples of this behavior include traditional communications such as town halls and webinars; sponsoring and participating in companywide events such as hackathons, reverse hackathons, and communicating success stories; and, finally, the most challenging, simple day-to-day actions such as consistently inquiring about how analytics is being applied to improve daily or critical business issues.
Understand if the analytics and upskilling strategy is working; if not, pivot effectively. Oil and gas leaders often approach decisions related to analytics and the underlying technology with the same mindset as traditional infrastructure decisions; however, this rigid approach often does not allow for discovery of unknowns and quick pivots. Digital and analytics have an inherent uncertainty and a rapid pace of evolution that makes them unique. Examples of pivots include acknowledging that select analytics use cases are not providing the underlying value, changing the emphasis on analytics skills in the training programs, or adjusting the underlying technology used to drive the analysis. Acknowledging that these pivots may take place when beginning the program often makes it easier to adjust when under way.
Conclusion
Talent management is a key ingredient for adapting to and thriving in the new energy landscape. This also is the component that takes time and a true transformational mindset to build. There couldn’t be a better time to get momentum behind this. As with any transformational change, upskilling the workforce for advanced analytics will require deliberate planning and effort from all ranks of organizations, including leadership support and sponsorship.
Manas Satapathy is a managing director in Accenture’s Strategy Energy practice. With more than 20 years of experience, he has worked with many of the largest oil and gas, mining, and oilfield-services companies to formulate and implement strategies and investment initiatives. Satapathy has published several articles on the evolution of global crude oil and natural gas landscapes.
Jessica Van Singel is a managing director in Accenture’s Talent and Organization practice. She has more than 20 years of experience leading complex, large-scale transformation efforts for clients across the energy portfolio, driving step-change improvements in leadership and workforce capability and bringing innovative thought leadership and data-driven insights to how we activate and enable sustaining behavioral and transformational change.
Aleek Datta is a managing director in Accenture Strategy and specializes in helping oil and gas companies improve performance and reduce costs through strategy, organization, and business-transformation enhancements. He collaborates with clients on global transformation and reorganization projects to help drive integrity, effectiveness, efficiency, and productivity advances.
Vikram Mukhi is a manager in Accenture’s Strategy Energy practice. He has more than 8 years of experience working for exploration and production and oilfield-equipment and -services companies. At Accenture, Mukhi focuses on advanced analytics, digital transformation, and operating model themes.