The oil and gas industry encounters severe operational and economic challenges on a daily basis due to the formation of stable water-in-oil emulsions over the course of the transportation and extraction of oils [1]. The presence of water causes various maintenance problems such as corrosion, fouling, etc., resulting in millions of dollars lost to the oil industry every year just on the maintenance of pipelines and reactors [2]. Additionally, the existence of water-in-oil emulsions reduces their oil recoveries and enhances their carbon footprints, which in turn imposes excess economic and environmental burdens on the oil industry [3].
There have been a significant number of attempts in the past to identify and comprehend the process(es) leading to the generation and stabilization of water-in-oil emulsions ([1], [4], and [5]). However, this problem persists due to the continued presence of microscopic water droplets (<10 μm), which go undetected and unremoved by conventional separation methods [4].
Here we propose a method for using data analytics to predict the overall concentration of these water droplets and help design targeted experiments to reduce or eliminate this problem.
Water-in-oil emulsions typically result from two different processes:
1. Shearing: When an aqueous-based extraction method is used to produce oil, the product undergoes tremendous amounts of mixing and transportation, which could cause the formation of water-in-oil emulsions. This is relatively well understood and could be controlled by manipulating the operating conditions.
2. Solubilization: Unlike shear-induced emulsification, the mechanism of solubilization does not require external forces. It is driven entirely by the thermodynamic tendency of naturally present surfactants in oil to associate with water (Fig. 1). As a result, the solubilization process likely produces the most stubborn emulsified water droplets in oil. This article will primarily focus on this method as a candidate for a data analytics and modelling solution to the emulsification problem.
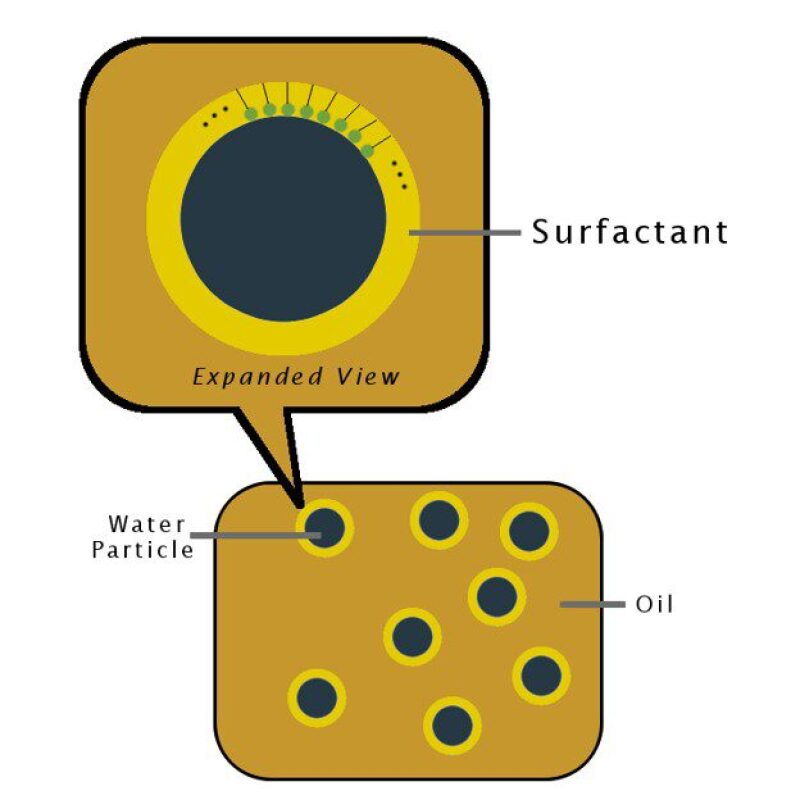
The long-established method of determining the solubility of water in oil is to first mix water and oil until achieving equilibrium, next to separate the emulsified water from oil by a settling or centrifugation process, and finally to measure the resultant water content by a suitable method (e.g., Karl Fischer titration) [5]. The challenge of implementing this method for petroleum oils is that mixing water and oil could produce extremely tight emulsions (shear-induced emulsification), and the resulting sub-micron-sized emulsified water droplets are practically impossible to remove, even with ultracentrifugation. Moreover, this method does not allow for measurement of solubilization rates of water in oil due to the opaque nature of petroleum oils. Lastly, the equipment employed in this method is delicate and expensive, making the method as a whole less attractive and less affordable for smaller companies and developing countries.
How Can Data Analytics Improve This Process?
Market Research. The first step involves gathering information on the particular properties of the oil in question. These properties could vary considerably based on factors like location, operating conditions, and extraction method. For example (Fig. 2), per the SARA analysis ([4] [6]), Canadian oil sands have higher asphaltene content compared to US crude oil, which makes Canadian oil heavier (lower diffusivity, D) and more polar (higher water solubility, c) than US crude oil. These properties are essential to predicting the solubility of water in oil as also shown below.
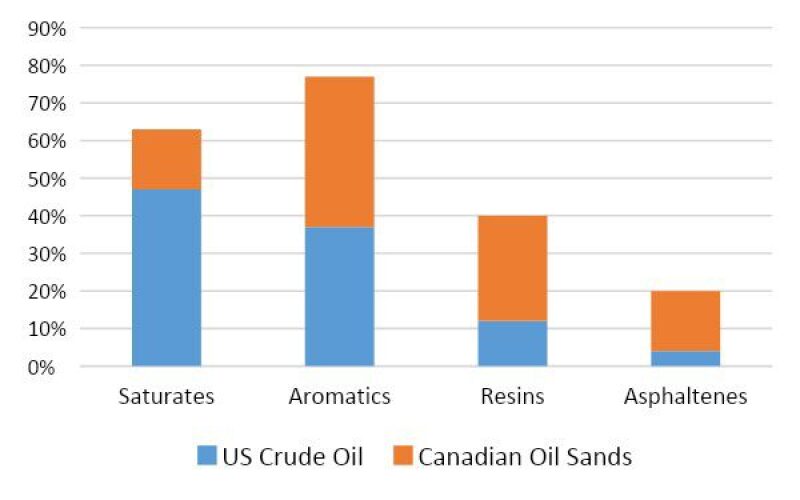
Modeling: A simple order of magnitude (scaling) analysis could help estimate the time required to solubilize the water in a given oil. Applying mass transfer at the water-oil interface yields the following equation [5]:
\(ρ_w dV/dt=-ρ_o k_m Ac\)
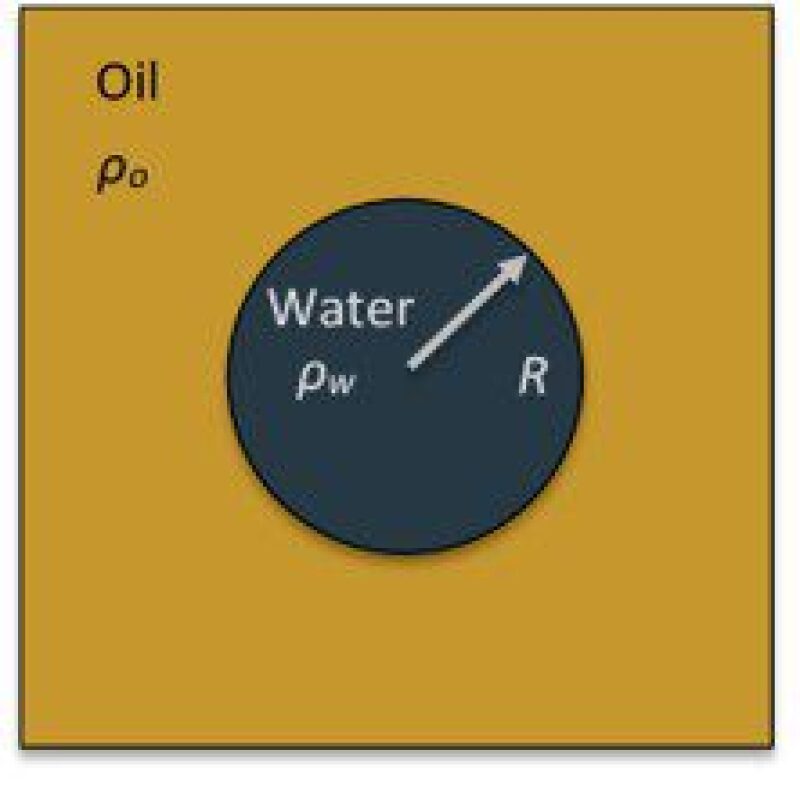
In case of a spherical drop with radius, R, this is equivalent to:
\(ρ_w dR/dt=-ρ_o k_m c\)
Here, ρw: water density; ρo: oil density; V: water volume; A: area of the water-oil interface; c: water-in-oil solubility; km: the mass transfer coefficient.
km is scaled as:
\(k_m\sim D^{0.5} G^{0.5}\)
where D is the diffusivity and G is the strain rate.
Therefore:
\(-dR/dt\sim ρ_o cD^{0.5} G^{0.5})/ρ_w \)
Upon the integration, a scaling analysis in conjunction with mass balance yields the governing equation for the solubilization time, in terms of oil and water properties (c, ρo, ρW, D) and operating conditions (R, v), as follows:
\(t_S\sim(ρ_W R)/(cρ_O D^{0.5} G^{0.5} ) \)
This information could be employed to alter operating conditions so that the solubilization problem could be suppressed if not completely stopped. As part of our approach, this information is fed into full-fledged Molecular Dynamic (MD) simulations to examine this problem in depth.
Simulation. As shown in the previous studies [7][8], Molecular Dynamic (MD) simulations could be employed to capture the progress of the whole solubilization process (Fig. 4) and estimate the water concentration in oil due to solubility. The predicted solubilization time in step 2 could be fed into MD simulations as an initial guess to kick off the simulations. Since the overall properties of the oil system change during the extraction of oil, MD simulations are important to comprehend the stages of the operation where the solubilization process could be expedited to still allow for taking necessary precautions.
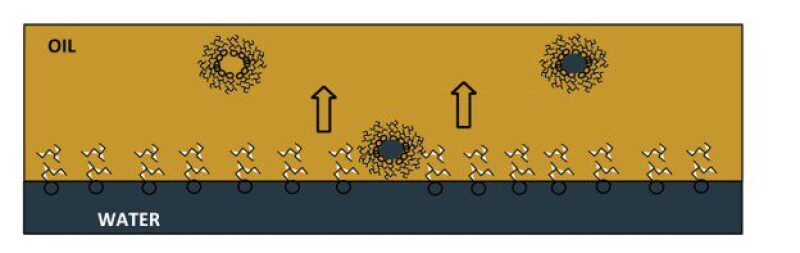
Design of Experiments. Conducting experiments with the water-in-oil system is challenging and expensive given the opaque nature of crude oil. Following the first three steps in this approach would provide a much more hospitable environment for designing more accurate experiments, which in turn would significantly reduce the number of experiments required overall.
A broad application of this approach could yield considerable savings of time and financial resources for oil industries struggling with this problem. Though not enough to solve the problem entirely, strategic application of carefully considered data analytics could enable a reduction in hours spent on designing targeted experiments, enabling the oil industry to potentially solve a long-standing problem of water-in-oil emulsions.
References
- Al-Sahhaf, T.; Elsharkawy, A.; and Fahim, M. 2008. Stability of Water-in-Crude Oil Emulsions: Effect of Oil Aromaticity, Resins-to-Asphaltene Ratio, and pH of Water. Petroleum Science and Technology, 26(17), pp.2009-2022.
- Czarnecki, J. 2001. Encyclopedic Handbook of Emulsion Technology, New York: Springer.
- Kiran, S.K.; Acosta, E.J.; and Moran, K. 2009. Study of Solvent−Bitumen−Water Rag Layers. Energy & Fuels, 23(6), pp.3139-3149.
- Sonthalia, R.; Ng, S; and Ramachandran, A. 2016. Formation of Extremely Fine Water Droplets in Sheared, Concentrated Bitumen Solutions via Surfactant-Mediated Tip Streaming, Fuel, vol. 180, pp. 538-550.
- Goel, S. 2019. The Roles of Dissolution, Coalescence, and Interfacial Tension in the Formation and Stability of Extremely Fine Water Drops in Concentrated Bitumen Solutions (doctoral dissertation, department of chemical engineering and applied chemistry, University of Toronto, Canada).
- Fan, T.; Wang, J.; and Buckley, J.S. 2002 January. Evaluating Crude Oils by SARA Analysis. In SPE/DOE Improved Oil Recovery Symposium. Society of Petroleum Engineers.
- Esselink, K; Hilbers, P.A.J.; Van Os, N.M.; Smit, B.; and Karaborni, S. 1994. Molecular Dynamics Simulations of Model Oil/Water/Surfactant Systems. Colloids and Surfaces A: Physicochemical and Engineering Aspects, 91, pp.155-167.
- Karaborni, S; Van Os, N.M.; Esselink, K.; and Hilbers, P.A.J. 1993. Molecular Dynamics Simulations of Oil Solubilization in Surfactant Solutions. Langmuir, 9(5), pp.1175-1178.
[The article was sourced from the author by TWA editors Bita Bayestehparvin, Joon Lee, and Jaspreet Singh Sachdeva.]