This column is part of a continuing series by Alec Walker, cofounder and CEO of DelfinSia.
Sustained lower hydrocarbon pricing demands a step change in oil and gas operational efficiency in order to free up cashflow and keep stock prices high. Digital transformation, the industry’s aspirational antidote, is necessarily driven by data practices. The rate of data proliferation in the oil and gas industry is large and accelerating, and the pressure on the industry to catch up in data science is strong and growing. Operators are talking about taking steps previously thought of as bold to bring in data science expertise, to rebrand themselves as technology companies, to work more closely and quickly with third-party software providers, and to share their data externally with the community for the sake of capturing more value. Some of this is actually happening, and at paces considered quick for an industry criticized for being slow moving. This is serious. The laggards are already failing.
Proliferating, also, is a slew of data science jargon, and one impediment to the speed of digital transformation is confusion over associated terminology. A good deal of this jargon is actually standard in the data science communities, but most of it is fresh in the general oil and gas community. This allows a new brand of slick salespeople on the scene, talking a big game using all the buzzwords that are associated with success but that mean something different to everyone saying them. The Society of Petroleum Engineers is beginning to make a concerted effort to help demystify the buzzwords, and this article kick starts that effort. Consider the following true anecdote provided in the second person from a friend running a department at a leading oil operator:
You’re sitting in your second-favorite conference room listening to an especially confident software vendor give a sales pitch. Apparently, this solution can do everything easily, and, yet, the “how” remains nebulous. “Artificial intelligence … big data … cloud computing … .” You suppress a deep sigh. Do these mean to him what they mean to you? You’ve had meeting after meeting like this ever since a strongly worded memo from the C-Suite about how your bonus will now be a function of your department’s success in achieving aggressive digital transformation benchmarks. Attempting to connect dots between various salesmen reveals some telling contradictions in the definitions of this “how” terminology. This salesman is talking fast and using your whiteboard as well as your projector screen, making egregious assumptions about your work flow and risks as he expounds the benefits of the software he represents. He’s comparing your workflow to an ATM, explaining that, because the options are finite and outcomes predictable, it will be a cinch to set everything up for immediate value-add to your team. The list of things he is overlooking about your situation and obscuring about his offering is growing faster than you can mentally store until the next time he takes a breath. “We can do everything I said by tomorrow, but, if you share this data and this data, too, we can do all that AND cure cancer.” He drops the marker like a microphone onto the white board sill, and, as he moves to sit down, you cannot help but stand up. You’re a bit surprised, yourself, but your legs are already walking, and you leave the room without a word.
Developing terminology is natural for practitioners in a field of study. A certain concept comes up enough in discussion to warrant a name. The name sticks because it is much easier to use than continuing to speak in definitions. With the concept fully understood by the practitioners, they can debate its merits relative to other concepts more quickly and precisely when they refer to it by name. A great example of the benefits and drawbacks of terminology is almost any legal document. Terms are defined up front in the first pages, and then the complexities that deserve independent scrutiny can be discussed around those terms. If you cut the first pages out of a legal document, however, the capitalized words cited again and again throughout the rest of it start to mean different things to different people, and responsible parties do not want to sign the dotted line. From how operators explain to us their interactions with digital transformation salespeople, our industry’s first page is missing. The goal, beginning with this article, is to corral the major buzzwords being thrown around in the oil and gas digital transformation circuit, put together appropriate definitions from data science and computer science, and then tailor each to the oil and gas industry. Each buzzword has been assigned a Nebulous Factor, a rating of how uselessly broad its general definition is. The lower the Nebulous Factor, the less excuse there is not to know and apply the general definition. The higher the Nebulous Factor, the more justified the expectation to have a case-specific definition provided. With a common baseline understanding of the terms, industry practitioners can have solid ground to stand on when evaluating digital transformation solutions. It is hoped, then, that vendors cannot hide their offerings behind nebulous buzzwords and best practices and solutions will rise to the top more quickly.
Terms
Data Science (Nebulous Factor: 4 out of 5)
Big Data (Nebulous Factor: 5 out of 5)
Artificial Intelligence (Nebulous Factor: 5 out of 5)
Machine Learning (Nebulous Factor: 2 out of 5)
Natural Language Processing (Nebulous Factor: 2 out of 5)
Neural Network (Nebulous Factor: 2 out of 5)
Deep Learning (Nebulous Factor: 3 out of 5)
Knowledge Graph (Nebulous Factor: 5 out of 5)
Cloud Computing (Nebulous Factor: 1 out of 5)
Parallel Processing (Nebulous Factor: 1 out of 5)
As a final note, it is important to remember that terminology is generated in all disciplines, including across the oil and gas industry. While it is wise to beware the software organization spouting buzzword-laden value propositions without a strong grounding in your industry, at the same time, do inform the ones that will listen about your own terminology. Terminology can be precise and particular within a certain group and very nebulous outside that group. Asking for clarification on buzzwords to align expectations and generate complete mutual understanding is the recommended best practice. For example, does your firm use “HSE,” “SHE,” “EHS,” or something else? A software vendor might be capable of adding enormous value to your company even though they stop you in your explanation of your use case to confirm that to you, “HSE” means “health, safety, and environment.” OK, just checking.
Data Science (Nebulous Factor: 4 out of 5)
Data science is a term for organizing and interpreting data, usually involving methods and practices from the fields of computer science and statistics.
On a scale of 1–5, data science gets a Nebulous Factor of 4. It is an umbrella term associated with a broad range of efforts, so people can have different things in mind when they use it. Simplistically and cynically, one could argue that data science applies to any time data is used to learn something. Ignoring newly captured digital data and continuing to drill and complete wells according to a long-standing tradition that has been profitable in the past is arguably still data science; the experiential data showed that the method worked. This is less common in the new economy, but there you have it. If you study data science in an academic program, you use statistics and computer science to learn how to organize complex data to derive elusive value. The industry is recognizing that such data can be captured, and it is beginning to recognize the existence of this more-elusive value, so data scientists are rightfully in higher demand. Given how nebulous “data science” is, you should not immediately assume associated value. When someone simply states that they use data science to accomplish something, this does not imply any specific methods or any degree of sophistication. Hence, in any circle, you have the socially approved right to ask for more information: “What methods will you use?” “What precedents in my application are there for these methods, and what results were obtained?” “How long does this take to implement?” “In what format must the data be, and how will you get it into that format?” A longstanding industry example of data science is deriving a predictive well decline curve from offset-well production, completion, geology, and other data. Another industry example of data science is deriving a more-optimal wastewater trucking schedule from data, including transportation costs, wastewater production by well, and well locations.
Big Data (Nebulous Factor: 5 out of 5)
Big data describes data sets large, complex, or dynamic enough that they must be analyzed using methods unnecessary when dealing with small, simple, or static data sets.
Big data gets a Nebulous Factor of 5 out of 5. Its definition suffers from moving goal posts and from enormous inconsistencies in affiliation. The term came from an attempt to characterize the data sets handled by major consumer-facing software firms, which had been collecting an increasing amount and range of data about an increasing number of target customers. Certain common practices in data science simply would not work on these data sets to derive value (forget your common spreadsheet application!), so more experimental and initially less effective methods had to be applied. Data scientists cannot be assumed to agree with one another about whether any particular data set qualifies as big data, but the term was meant to be used essentially to express that the data set will be challenging. The term still provides some utility when used to qualify a data set by the methods required to process it, but less as time goes on. Increased computational power, increased access to data, and improvements in data science over the years have led to a growth in effective methods to derive value from many types of data sets, big or otherwise. The big data qualifier, as a result, is less and less meaningful. In fact, some practitioners use the term to imply that the data set in question is inherently more exciting, rather than less. This is because of a popular assumption that there must be more value in any large volume of data than any small volume. This is also because there tend to be less stones unturned in such data sets. (Many data sets traditionally defined as “big” have still not been tapped with newer data science methods by their owners, presumably because the owners are ignorant of such methods.) Unfortunately, for its utility, the term has captured the public imagination and has adopted many creative associations. Big data is sometimes used to connote a method in and of itself, as in, “We use big data to give you instant insights from your drilling reports.” If the drilling reports are the data set, what does big data refer to here? If someone tells you they use big data to do something for you, you might start by asking whose big data; how they will access it; what method they are talking about; and whether they cannot instead use smaller, more-organized, and more-bounded data to do the same thing for you more quickly and effectively.
Artificial Intelligence (Nebulous Factor: 5 out of 5)
Artificial intelligence (AI) describes computer methods that do what humans otherwise do.
AI gets a Nebulous Factor of 5 out of 5. At some point, a computer did some calculating that impressed a human, and this buzzword was coined. It is important to remember that, before the age of computers, humans did all the calculating themselves. Computers continue to expand the range of calculations that can be performed, and their tendency to process calculations more quickly, accurately, and cost-effectively than humans means this expanding range continues to create value. What is impressive or inherently human-like about these machine capabilities is open for interpretation, as is the distinction between “intelligence” and “artificial intelligence.” Focusing on that is a semantic distraction. Like “big data,” AI has captured the public imagination and has now adopted many creative associations. It can have an almost magical allure, implying somehow that humans are no longer needed anywhere, as if the ability to store all chess moves in an array and organize them by probabilities must mean that machines could supplant the human beaten at chess in any other human activity. At this point, pretty much any software practice can cite another similar software practice (or television show reference) that uses the term AI as justification for affiliating itself with the buzzword. Such affiliation has tended to lend an air of mystical credibility and sophistication, but this is thankfully beginning to change. You should not be wowed by any and all mention of AI, but you also should not instantly shun anyone using the term. It can be a credibility facade behind which may lurk nothing but vaporware, and, yet, well-meaning people use it to refer to real practices that add real value. Some are tempted to reject outright any entity using the buzzword on suspicion of what we might call “all sizzle and no steak,” or, if you prefer, “all hat and no cattle.” While this skeptical faction grows, software vendors still show an increased likelihood of speaking opportunities at conferences and paper publishing acceptances when they incorporate this buzzword into their vernacular. During this confusing transition time, be wary of pride that starts and ends with “AI,” but otherwise indulge entities making use of the buzzword to see what they are actually talking about. Brush the term aside and look for the meat.
Machine Learning (Nebulous Factor: 2 out of 5)
Machine learning (ML) describes the iterative adjustment of models to fit data.
ML gets a Nebulous Factor of 2 out of 5. ML has a strict definition in the world of computer science. Unlike most of the buzzwords in this column, scientists and engineers use this term in their work to communicate precise information. ML implies that a machine with access to parameters, a model, and a data set undergoes the following loop: (1) uses the model to guess what the data is, (2) measures the difference between the model and the actual data, and (3) adjusts the model. Once the model fits the data well enough to satisfy a condition in the parameters, the loop is broken, and the ML process ends. Machine learning is so named because the machine improves the model. If you have ever used the curve-fit or Solver functionalities in Microsoft Excel, you are a practitioner of machine learning. Machine learning can get very complex when applied to more-complicated data sets including those with more than two dimensions. Machine learning is typically used either to sort data into categories (Which of these satellite photos contain a wellhead?) or to predict data (What will be next month’s production from this well?). Depending on complexity of the project, at some point, machine-learning experts are needed to ensure that the appropriate parameters, models, and data are used to drive value. Two common problems with machine learning in the industry are the extreme lengths practitioners must undergo to collect and organize data as well as the tendency to overfit the model to the data. Overfitted models sacrifice general applicability for specific applicability, meaning they cannot be reused reliably. Despite its rather neat definition, ML is still a buzzword and is still associated with obfuscation. Some vendors assume that the prospective client will not be familiar with the definition of machine learning, so the term can be used to convey credibility in place of more thorough means. Prove these vendors wrong.
Natural Language Processing (Nebulous Factor: 2 out of 5)
Natural language processing (NLP) is the use of computational methods to organize and extract value from human language.
NLP gets a Nebulous Factor of 2 out of 5. Scientists and engineers use the term “natural language” to mean the language that humans use to communicate with other humans, as opposed to the language the humans use for any other purpose, including traditional communication with machines. NLP is simply the processing of that human-to-human language. This could involve using algorithms on text files to extract knowledge or data, it could involve using algorithms on audio files to convert speech into text, or it could arguably involve using algorithms on video files to interpret words from the movement of lips (though some might call that one step before the actual NLP). If you ever used enterprise search engines or hit control+F in a document, you are an NLP practitioner. Like machine learning, NLP requires experts for advanced applications. For example, how far will a keyword search get you when you are looking for all the possible causes for a certain effect (Why is my downhole pump failing?), especially when there are many documents each with many instances of the keywords “downhole,” “pump,” and, “fail?” While on-demand extraction of data and knowledge from industry textual files is an exciting prospect, many NLP vendors fail to mention up front that they require their clients to train the provided algorithms for months or even years before value can be added. While NLP is not currently too nebulous of a term, it is under threat of becoming more nebulous for two reasons. First, as the science and art of NLP progress, the distinction between how humans interact with humans and how humans interact with machines becomes blurrier. As a result, what constitutes natural language becomes more nebulous. Second, as more and more organizations begin to compete in the area of NLP, the buzzword risks increasingly creative affiliations that confuse precise mutual understanding. When vendors claim to be able to use natural language processing on your data, ask how your team-/firm-/industry-specific phrasing and terminology will be taken into consideration and how many of your experts’ hours will be tied up before the tool works. Focus on a specific use case that you know how to measure, and make sure you are aligned with the service provider.
Neural Network (Nebulous Factor: 2 out of 5)
A neural network is a model that relies on machine learning to adjust weights assigned to variables in a chain of functions.
Neural network gets a Nebulous Factor of 2 out of 5. Like many of the now popular concepts in data science, neural networks date back several decades. They were first proposed as models of how biological brains work, with the individual groupings of variables (called “nodes”) meant to resemble neurons. Nodes are strung together in layers, some receiving as input the outputs from other nodes, hence the term “network.” Each node runs a function on its inputs to yield an output, and the final node yields the final output of the network. Neural networks rely on machine learning to adjust weights assigned to the variables in each node to alter the output of the functions. At some point in the machine-learning loop, the weights in each of the nodes are optimized to yield a final output surpassing an accuracy threshold. While this definition may sound complex, it conveys a standard bounded meaning in the data science world. Neural networks are sometimes referred to as “artificial neural networks” to distinguish them from the neural networks in biological brains. It is important to remember that neural networks cannot add value before being trained toward a specific goal on collected, organized, and labeled data. If someone says they can use neural networks to accomplish something, make sure the data acquisition and labeling plan is clear, including whose job it is to do that part.
As an industry example, say we want to train a neural network so that it can trigger a computer to alert humans automatically when a pipe is leaking. We would feed the network numerous labeled pictures, some containing leaking pipes. Attributes that can be measured from a picture by a computer, such as how many black pixels it contains, would become the input variables for the nodes in the first layer of the network. The calculated outputs from these nodes are passed along to the next nodes until the final node makes a guess as to whether the given picture contains a leaking pipe. Because the picture is labeled, the model can compare the guess to the label to determine correctness. Whenever the guess is wrong, the model can adjust weights internally before guessing again. Eventually, the model emerges from the machine learning process capable of analyzing pictures to determine leaks. Cameras taking regular pictures of pipes then can feed those pictures to the trained neural network, which can determine with some accuracy whether the pipe has sprung a leak since the last picture it analyzed. These kinds of applications are common on assembly lines in manufacturing.
Deep Learning (Nebulous Factor: 3 out of 5)
Deep learning is a term for the machine learning that is applied to a neural network.
Deep learning gets a Nebulous Factor of 3 out of 5. Deep learning is one type of machine learning, implying that the model being trained by the machine learning is a neural network. Data science practitioners refer to deep learning as a method of applying machine learning. “Did you try deep learning for that problem?” would imply, “Did you try training a neural net to address the problem?” While deep learning has a precise definition among data science practitioners, it has a far less precise definition among some others. A common problem seems to be the use of deep learning to qualify a solution that potentially has nothing to do with neural networks, as if, rather than having merely learned, the software solution deeply learned and is now much more capable. Neural networks are not universally superior to other models. If someone is using deep learning to justify the merit of a solution, you should explore whether they mean that they used a neural network to model something and, if so, why the neural network was the right choice.
Knowledge Graph (Nebulous Factor: 5 out of 5)
A knowledge graph is a collection of ideas connected by defined links.
Knowledge graph gets a Nebulous Factor of 5 out of 5. The general concept of ideas connected with links is something easily understood, but the term communicates no clear meaning without elaboration, and it is still subject to misuse. Knowledge graphs are also called “knowledge bases,” which technically implies that the links between ideas are not necessarily arranged in a graphical format. The terms are used interchangeably, nonetheless. Connecting the reader to this article by the fact that the reader read this part of this article constitutes a knowledge graph. The graph contains two ideas—the reader and the article—and it contains one defined link: the fact that the reader read at least part of the article. This knowledge graph could potentially be useful, because it could be used to determine whether the reader has read part of this article. Take the user as an input and return something about this article as an output. Typically, knowledge graphs have many different types of ideas and links. Any one of the ideas can become an input yielding information about the relationship between that idea and at least one other idea in the knowledge graph based on the type of link. Knowledge graphs potentially are quite useful to find and interpret data. Google uses a knowledge graph to assist its core search engine by connecting ideas that users enter in their searches to other ideas related through links in the knowledge graph. There seem to be many interpretations of knowledge graphs touted by software vendors, some of which are connected to the very general definition provided here. Knowledge graphs are not inherently helpful or impressive. If someone communicates that they have a knowledge graph and that it should be respected, you can ask what kinds of ideas it connects, what kinds of connections it makes between those ideas, and how comprehensive it is. Note that a knowledge graph does not need to be objectively large to be comprehensive, and it does not need to be comprehensive to be useful. For reference, Google said its knowledge graph contained approximately 70 billion connected ideas in October of 2016. Google is still working hard to be able to answer every question it wants to answer.
Cloud Computing (Nebulous Factor: 1 out of 5)
Cloud computing is the practice of using computers other than the user’s device for storage and computation.
Cloud computing gets a Nebulous Factor of 1 out of 5. It has a simple definition clear to anyone who has used the Internet. Using a web browser is cloud computing, as servers (other computers) need to hunt for and send back information to your device. Those companies that have graduated from troglodytic physical file cabinets traditionally stored their data on their own local machines, but leaders migrated decades ago to central servers so that information could be more readily accessible. If something is stored on a shared drive, everyone can find it in the same place. If someone makes an edit, they edit the file for everyone (historical versions of the file remain accessible), so there are not multiple live conflicting files floating around in email attachments. Centralized storage and processing also created economies of scale. The oil industry is now following other industries and outsourcing their cloud computing to third-party vendors, typically major software companies. This creates further economies of scale, and it pushes the liability of protecting the data to those with the deepest pockets and the most expertise. Most software vendors also outsource to third party cloud providers for the same reasons. Oil companies traditionally concerned about sharing data with software providers can rest assured from a security standpoint when those providers are outsourcing cloud computing. This has given rise to the peripheral buzzword “cloud native.” Cloud native software is simply software built to run on cloud networks rather than on a local machine. It equates to less risk and more utility. Pretty much all software is cloud native these days, so be wary of vendors using this characteristic as a major selling point.
Parallel Processing (Nebulous Factor: 1 out of 5)
Parallel processing describes the computation of multiple parts of a single process simultaneously on separate processors.
Parallel processing gets a Nebulous Factor of 1 out of 5. It has a common and static definition. It is important to note that many modern computers contain multiple processors allowing parallel processing to happen on the same machine. Parallel processing is very useful in implementing deep learning on complex neural networks with large data sets because the task of calculating node functions can be split across multiple processors simultaneously. This means that neural networks can learn faster and their human designers can more quickly assess and tweak design imperfections. As parallel processing is tied to ML and AI, it runs the risk of following these other buzzwords and expanding its definition beyond the current clear and useful version. Be wary when someone tells you they use parallel processing to get something done that must be accomplished in series, and, likewise, be wary when you hear that parallel processing is to be used when the associated hardware is not available.
Read Alec Walker's previous columns here:
Oil and Gas Has a Problem With Unstructured Data
Leave thoughts and comments for Alec Walker here.
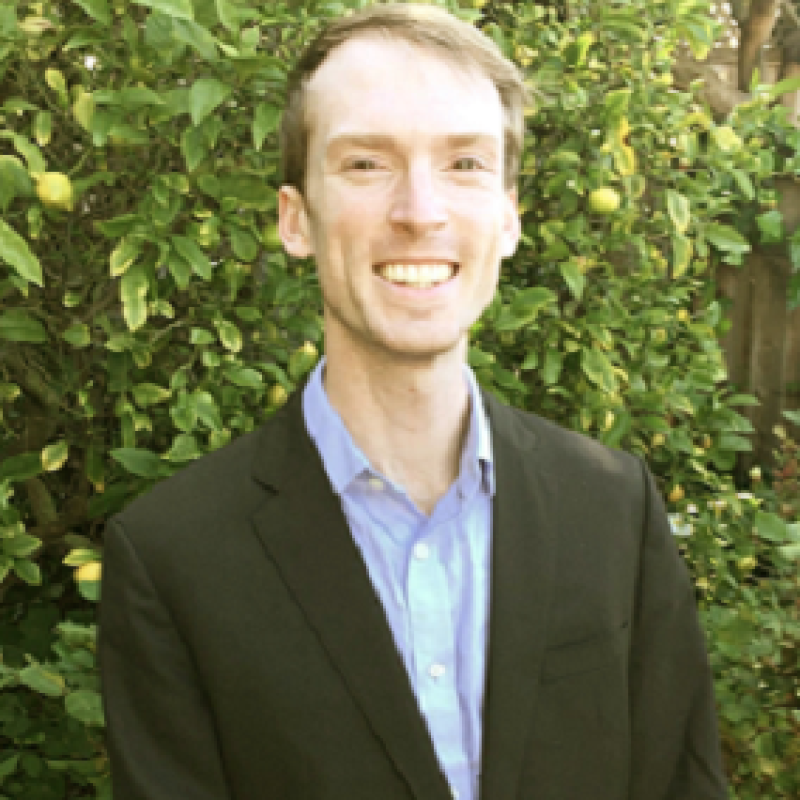
Alec Walker is the cofounder and chief executive officer of the natural-language-processing and data-analytics firm DelfinSia in Houston (LinkedIn). Delfin helps the oil and gas industry extract value from unstructured data. Walker has led digital-transformation and internal entrepreneurship projects for a variety of leading organizations including Intel, Inditex, AECOM, and General Motors. He has worked for Shell as a technical service engineer in refining, a tech tools software product manager, and as a reservoir engineer in unconventional oil and gas. Walker holds a BS degree in chemical engineering from Rice University and an MBA degree from the Stanford Graduate School of Business.