The study described in the complete paper develops an explainable data-driven method using five methods—recurrent neural networks, XGBoost, the group method of data handling (GMDH), CatBoost, and genetic programming (GP)—to create a model using a multidimensional data set with more than 700 rows of data for predicting minimum miscibility pressure (MMP). Moreover, two robust correlations are developed that can be used for a wide range of parameters. The vast range of features in the data set makes it suitable to study the effects of different parameters on MMP in conditions representative of CO2 enhanced oil recovery and carbon capture, use, and storage.
Introduction
Numerous studies shed light on the development of empirical models used for MMP forecasting. Nevertheless, the accuracy levels of most correlations are inadequate because of the methods used to construct the equations used in the model’s development. Data-driven techniques, however, make use of diverse data to track and determine connections between factors.
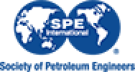