To unlock the potential of large relative permeability (Kr) databases, the work flow proposed in the complete paper integrates data analysis, machine learning (ML), and artificial intelligence (AI). The work flow allows for the automated generation of a clean database and a digital twin of Kr data, using AI to identify analog data from nearby fields by extending the rock-typing scheme across multiple fields for the same formation.
Introduction
Accurate Kr curves are critical because they can improve reservoir characterization, reduce uncertainty in history matching and production forecasting, and provide robust and reliable field development plans. However, preparing special core analysis (SCAL) data, particularly Kr curves, as an input for reservoir simulation traditionally has been a highly manual, time-consuming, labor-intensive process. Furthermore, no automated tools are available currently that perform a full quality review of Kr data using analytical or numerical methods. As a result, the quality and representativeness of Kr data used in reservoir simulation models is frequently inadequately checked.
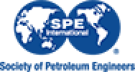