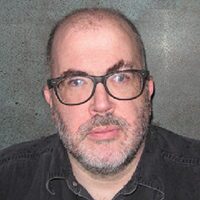
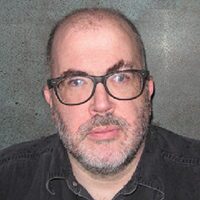
-
After 9 years of contributing to JPT, it’s time to hang up my spurs. I wanted to use this opportunity to raise a couple of issues, namely the effect and utility of machine learning and the usage of point-and-click engineering software.
-
The notion of reducing our environmental footprint, minimizing leaks and spillages, and identifying operational efficiencies is nothing new. We have been addressing these issues for years. Sustainability, however, has gained a higher profile recently.
-
In my view, we still do not possess a full understanding of oil production in unconventional fractured reservoirs. Our ability to forecast such assets remains elusive, even with copious amounts of analytics, mountains of data, and an arsenal of machine-learning tools.
-
A radical digital revolution is happening all around us (or so we are told). Applying this to reservoir simulation, we apparently need to understand better when and, more importantly, when not to use such technology—to appreciate its bounds, its limitations, its range of validity, and so on.
-
Nearly a decade after an SPE meeting in Bruges set industry-inspiring benchmarks for reservoir modeling, the time has come to overcome a new set of challenges.
-
Reservoir-simulation-model inputs are numerous, and uncertainty is pervasive—before, during, and after development. With the pressure to deliver results quickly, how do we find the right balance?
-
Coupled, full-field, integrated, holistic, or asset: Call it what you will, this class of modeling is becoming increasingly commonplace.
-
As the development of shale oil and gas becomes increasingly significant, so does the need for modeling tools for their accurate and timely forecasting.