Time savings and bit longevity are major challenges in coal-seam gas (CSG) unconventional fields onshore Queensland. Maximizing rate of penetration (ROP) on the basis of optimal drilling parameters was the key to tackling these issues. A formal process for optimizing performance was developed, with a focus on optimizing polycrystalline diamond compact (PDC) bit design and drilling hydraulics and developing a drillers’ road map. As a result, ROP increased from 50 to 150 m/h.
×
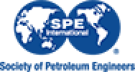
Continue Reading with SPE Membership
SPE Members: Please sign in at the top of the page for access to this member-exclusive content. If you are not a member and you find JPT content valuable, we encourage you to become a part of the SPE member community to gain full access.