This paper discusses the technical challenges related to implementing a rigsite, real-time drilling advisory system and current solutions to these challenges. The system uses a data-driven response-surface model based on physics-based calculations to optimize rate of penetration (ROP) while minimizing drilling-vibration dysfunction with regard to lateral (whirl) and torsional (stick/slip) vibrational modes. Minimizing these vibrations is important to mitigate bit damage that can lead to reduced ROP and increased bit trips.
Technical Overview
The system is a rigsite software application that should be deployed in view of the driller. Fig.
×
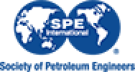
Continue Reading with SPE Membership
SPE Members: Please sign in at the top of the page for access to this member-exclusive content. If you are not a member and you find JPT content valuable, we encourage you to become a part of the SPE member community to gain full access.