This paper presents a data-driven technology and associated work flow for fast identification of field-development opportunities in mature oil fields that accelerates subsurface field-development planning and reduces the time requirement from months to weeks. This technology is ideally suited for large, complex oil fields with large data sets and has been used for brownfield rejuvenation, for asset evaluation during acquisition, and as an independent validation system within internal review programs for large oil companies.
Introduction
This approach does not require full-field static or dynamic modeling but retains a high degree of confidence by coupling a reliance on data and analytics with judgment of experienced subject-matter experts who quickly validate results. This methodology relies on automated or machine-assisted geological and engineering work flows. Because the forecasting algorithms use regression or neural-network techniques that train on historical data, this approach is not applicable to undeveloped or early-stage greenfields.
Methodology
This technology relies on rapid integration and quality control of key geological and well data, including wireline logs, production/injection by well, completions data, and well trajectories.
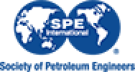