The authors describe a machine-learning (ML) approach for processing distributed-fiber-optic-sensing (DFOS) data that enables dynamic flow-profile monitoring using a fiber-optic electric-line cable deployed in a gas condensate well and compare the method with a conventional approach. DFOS technology has the potential to provide more-efficient and dynamic flow profiles compared with traditional methods, particularly in high-rate gas wells where production logs (PL) are recorded at reduced rates to avoid tool lifting.
Technology and Field Introduction
DFOS surface acquisition systems launch short pulses of light into an optical fiber, then receive its backscatter and convert it into physical measurements: temperature in the case of distributed temperature sensing (DTS) and acoustic intensity in the case of distributed acoustic sensing (DAS). These systems essentially turn a fiber-optic cable into a long array of thermometers (DTS) or multifrequency microphones (DAS). The DFOS systems record the backscatter signal continuously and measure at multiple points along the fiber. The major advantage of DFOS acquisition is the ability to record data throughout time at a very high sampling frequency.
Unlike traditional PL tools, insights provided by DFOS offer a real-time view of changes in production without intervention.
A successful trial was performed in a high-rate gas-producing well to test the quantitative inflow profiling algorithm on DFOS data. As part of the work, conventional PL data were acquired in high-rate gas production Well A01. An electric-line cable with built-in multimode and single-mode fiber-optic line was used to acquire conventional PL data.
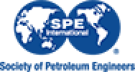