Data mining for production optimization in unconventional reservoirs brings together data from multiple sources with varying levels of aggregation, detail, and quality. The objective of this study was to compare and review the relative utility of several univariate and multivariate statistical and machine-learning methods in predicting the production quality of Permian Basin Wolfcamp shale wells. Methods considered were standard univariate and multivariate linear regression and the advanced machine-learning techniques support vector machine, random forests, and boosted regression trees.
Introduction
In the last few decades, because of the diminishing availability of conventional oil reserves, unconventional reservoirs are fast becoming the mainstream source of energy resources. In the meantime, with technology advances in data collection, storage, and processing, the oil and gas industry, along with every other technical industry, is experiencing an era of data explosion.
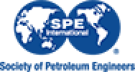