The revolutionary growth of shale oil and gas development over the past 2 decades has pushed operators increasingly toward a manufacturing approach to resource development. Thousands of wells are drilled in the leading shale plays to offset decline rates as high as 50% in the first year (Oil+Gas Monitor 2014). However, the advancements in drilling and completions methods that have driven the growth of shale plays reflect two different underlying trends in regard to costs.
As an example, EOG Resources reported to investors that its average drilling days per well in the Eagle Ford Shale in Texas declined from 14.2 in 2012 to 4.3 in 2015 (EOG Resources 2015), a 70% decrease. However, during the same span, its overall costs per well only declined from USD 7.2 million to USD 5.7 million (The Value Investor 2015), a 20% decrease. So what explains this divergence? The increasing intensity and cost of the completion program is most likely a major factor.
The methods that have driven down completion costs have focused on using less expensive and more efficient materials and equipment. However, there is also an incipient, growing industry movement toward evaluating the potential gains by engineering the locations of stages and perforation clusters in relation to the varying geological properties along the wellbore.
Historical evidence indicates that between 30% and 50% of perforation clusters do not produce. With each stage costing USD 100,000 to USD 200,000, this area is a substantial source of potential capital expense savings and increased production.
Whether the goal is to engineer completions or improve well placement, companies need better information on the geological complexity of the reservoir to advance along the learning curve in shale and improve results. However, the shift from traditional vertical wells to the horizontal wells that have driven the shale boom has dramatically increased the costs of formation evaluation.
High Cost of Logging
Including rig time, the average cost to log a horizontal well with shuttle-deployed or pump-down tools is approximately 10 times the cost of deploying wireline tools in a vertical well. Even so, the horizontal formation evaluation logging market has been growing at a 40% annual rate, which underscores the importance of the logging data.
However, there clearly is a problem as factory-style field development increases. An operator possibly can afford a quarter- to a half-million dollars per well to log a handful of appraisal wells with these high-end services. But what about the hundreds or thousands of wells that follow? There has not been an economic means of logging wells on this scale with this business model. And the recent downturn in global oil prices has intensified the problem of high logging costs as the pressure to reduce completion budgets builds.
Fortunately, the manufacturing approach to field development that accentuates the problem of logging costs also points to a solution. With their thousands of wells being drilled, completed, and put into production, the shale plays provide an ideal environment for collecting data and transforming that data into insights, a practice that at least loosely reflects the larger movement toward big data. Virtually every well gathers a trove of real-time drilling data, and when multiplied by the thousands of wells, the accumulated data hold tremendous leveraging potential in improving the drilling, completion, and production process.
Big Data to the Rescue
Witnessing the growing use of big data to increase drilling efficiency, Quantico Energy Solutions began 2 years of research to determine whether the data could also be an information source for formation evaluation characteristics.
As a result of the research, the company recently commercialized a logging system called QLog, which leverages proprietary machine-learning software and a vast library of horizontal logging and drilling data from a number of wells that exceed those of virtually any oil and gas company. Developed with support from several major shale operators, the system is economic for obtaining simulated logs on every well drilled without having to place a tool in the well.
The system was developed by industry specialists in neural networks and openhole logging tool design. By training neural network models with horizontal wells that possess drilling and logging data, the QLog system is able to simulate compressional, shear, and density logs on horizontal wells in which conventional logging tools are not run. From these three primary logs, elastic properties such as Young’s modulus, Poisson’s ratio, horizontal stress, and brittleness can be derived.
The data-driven nature of the company enables it to pursue a faster pace of innovation than if it were to design physical logging tools. For example, the company aims to launch within months a real-time log simulation service to assist drillers with well placement. The network effect of various operators aggregating data through the company also leads to several advantages. Simulation models specific to a local field can be generated as quickly as in a few days. In addition, operators that lack horizontal logs can use the existing models that have been constructed for active plays such as the Permian, Bakken/Three Forks, and Eagle Ford basins.
The simulated logs have been shown in several blind tests conducted by operators to show repeatable accuracy that is consistent with conventional logging tools. “The key aspect of the system is it eliminates lost-in-hole risk and onerous costs without sacrificing measurement quality,” said Chuck Matula, president of Quantico.
Built on drilling data, the company’s simulation models have proved significantly more accurate in the prediction of horizontal logs than the benchmark method of extrapolating gamma ray data.
An analysis was conducted by Quantico and a major operator in the Permian Basin comparing more than 70 log simulations that relied on gamma ray vs. the addition of drilling data alongside the gamma ray. The QLog outperformed the gamma ray model in the large majority of instances (Fig. 1 above).
In the remaining instances, the better accuracy from using gamma ray alone proved to be statistically insignificant. In other words, the drilling data not only increased the accuracy of the simulated logs, but it also meaningfully increased the accuracy compared with a traditional approach of relying on gamma ray data alone.
Case Study: West Texas
An operator needed an economic means of generating geomechanical logging measurements on wells in the Delaware Basin of west Texas. Historically, the operator had run conventional openhole logs on its wells in the basin. However, with the steep drop in oil prices, the cost of conventionally logging a hole deviation of 90° and a lateral section of more than 5,800 ft was prohibitive.
Using the QLog system, with local area data contributed by the operator to supplement Quantico’s library of well data, log simulation models were produced within a week without running a tool into the hole. The models’ inputs were soon customized to incorporate specific measurements collected by the operator during drilling.
The model was used to produce simulated compression, shear, and density logs. Subsequently, Quantico used its proprietary QFrac software, which uses the simulated logs to automatically generate elastic properties information and recommend engineered stage locations, to provide the operator with Young’s modulus, Poisson’s ratio, horizontal stress, and brittleness data (Fig. 2). The operator was thereby able to examine optimal stage length and perforation cluster positioning.
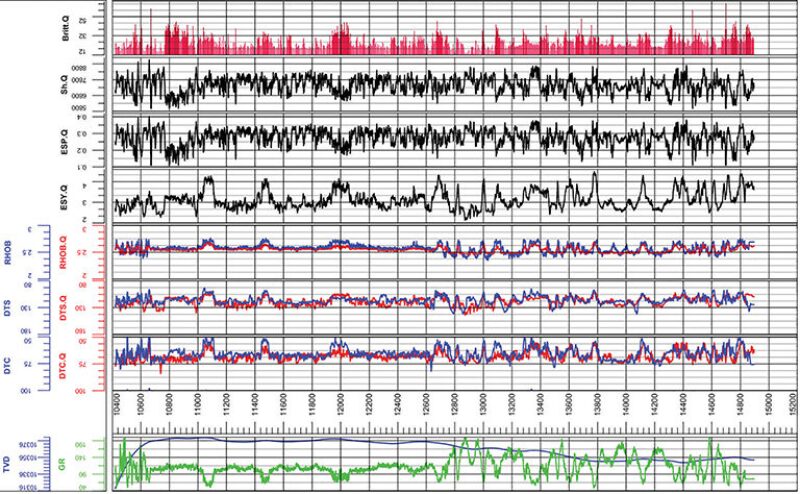
With the use of the simulated logs, the operator was able to obtain critical formation data of a quality consistent with conventional logs and reduce logging-related costs by 90%.
Looking Ahead
“We believe that we are only in the ‘first innings’ of identifying data-mining methods that solve salient problems for oil and gas companies,” Matula said. With such methods, similar solutions may be leveraged for areas such as drilling optimization, geophysics, and production logging.
“The success or failure of future data-mining solutions lies largely in identifying where data exists but may be underutilized, rather than requiring new measurements to be taken, and then providing industry professionals with data they can use in existing workflows and software platforms,” Matula said. “If so, then the rewards from even marginal Capex (capital expenditure) savings or higher production on each well can be replicated on thousands of wells.”
Such innovative methods and relentless attention to driving efficiencies are critical to the economic future of shale resource development, especially in a low-price commodity environment, he said.
References
EOG Resources. 2015. What’s New in 2015? 4 May 2015. (accessed 17 June 2015).
Oil+Gas Monitor. 2014. Oil Demand and Well Decline Rates Ensure Strong Outlook for Oil Industry. 5 November 2014. (accessed 17 June 2015).
The Value Investor. 2015. EOG Resources—Disappointing Production Guidance, as “Normal” Earnings Cannot Support the Valuation. Seeking Alpha, 19 February 2015. (accessed 17 June 2015).