In this column, I provide a book report on Nancy Leveson’s Engineering a Safer World (2012).
The central premise of the book is: The process hazard analysis methods we use today were designed for the relatively simple projects of yesterday and are inadequate for the complex projects we build today.
I agree with her.
Would It Have Prevented Bhopal?
My litmus test of a new process hazard analysis technique is: “Would this approach have prevented the Bhopal accident?” A typical HAZOP (hazard and operability study) would not have prevented Bhopal in my opinion. I believe that Leveson’s systems-theoretic process analysis (STPA), a process and safety-guided design approach, would have.
Why Do We Need a New Approach to Safety?
The traditional approaches worked well for the simple systems of yesterday. But the systems we are building today are fundamentally different.
Reduced ability to learn from experience because of
The increased speed of technology change
Increasing automation removes operators from direct and intimate contact with the process
Changing nature of accidents from component failures to system failures due to increasing complexity and coupling
More complex relationships between humans and technology
Changing public and regulator views on safety. Decreasing tolerance for accidents.
Difficulty in making decisions because at the same time as safety culture is improving, the business environment is getting more competitive and aggressive
Accident models explain why accidents occur and they determine the approaches we take to prevent them from recurring. Any such model is an abstraction that focuses on those items assumed to be important while ignoring issues considered less important.
The accident model in common use today makes these assumptions:
Safety is increased by increasing system and component reliability.
Accidents are caused by chains of related events beginning with one or more root causes and progressing because of the chance simultaneous occurrence of random events.
Probability risk analysis based on event chains is the best way to communicate and assess safety and risk information.
Most accidents are caused by operator error.
This accident model is questionable on several fronts.
Safety and reliability are different properties. A system can be reliable and unsafe.
Component failure is not the only cause of accidents; in complex systems accidents often result from the unanticipated interactions of components that have not failed.
The selection of the root cause or initiating event is arbitrary. Previous events and conditions can always be added. Root causes are selected because
The type of event is familiar and thus an acceptable explanation for the accident.
It is the first event in the backward chain for which something can be done.
The causal path disappears for lack of information. (A reason human error is frequently selected as the root cause is that it is difficult to continue backtracking the chain through a human.)
It is politically acceptable. Some events or explanations will be omitted if they are embarrassing to the organization.
Causal chains oversimplify the accident. Viewing accidents as chains of events and conditions may limit understanding and omit causal factors that cannot be included in the event chain.
It is frequently possible to show that operators did not follow the operating procedures. Procedures are often not followed exactly because operators try to become more efficient and productive to deal with time pressures and other goals. There is a basic conflict between an error viewed as a deviation from normative procedures and an error viewed as a deviation from the rational and normally used procedure. It is usually easy to find someone who has violated a formal rule by following established practice rather than specified practice.
We need to change our assessment of the role of humans in accidents from what they did wrong, to why it made sense to them at the time to act the way they did.
Complexity Primer
Project management theory is based generally on the idea of analytic reduction. It assumes that a complex system can be divided into subsystems, and that those subsystems can then be studied and managed independently.
Of course, this can be true only if the subsystems operate independently with no feedback loops or other nonlinear interactions. That condition is not true for today’s complex projects.
Complex systems exist in a hierarchical arrangement. Even simple rules sets at lower levels of the hierarchy can result in surprising behavior at higher levels. An ant colony is a good example (Mitchell 2009):
A single ant has few skills—a very simple rule set. Alone in the wild it will wander aimlessly and die. But put a few thousand together and they form a culture. They build and defend nests, find food, divide the work.
Culture? Where did that come from? No scientist could predict ant culture by studying individual ants.
This is the most interesting feature of complex systems. Culture is not contained within individual ants; it is only a property of the collective. This feature is called emergence—the culture emerges.
An emergent property is a property of the network that is not a property of the individual nodes. The sum is more than the parts.
Safety is Emergent
There is a fundamental problem with equating safety with component reliability. Reliability is a component property. Safety is emergent. It is a system property.
The system is hierarchical (Fig. 1).Safety depends on constraints on the behavior of the components in the system including constraints on their potential interactions and constraints imposed by each level of the hierarchy on the lower levels.
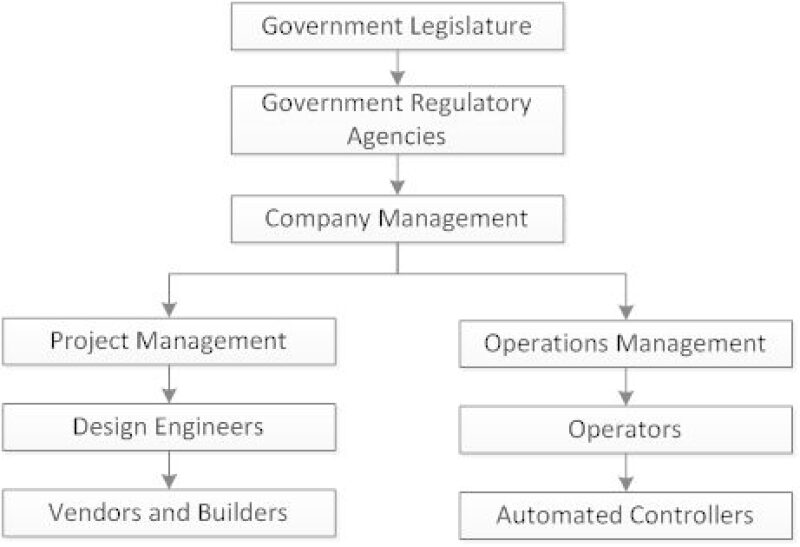
Safety as a Control Problem
Safety depends on system constraints; it is a control problem.
Fig. 2 is a simple control loop. We are all familiar with control loops for controlling process variables. This is no different.
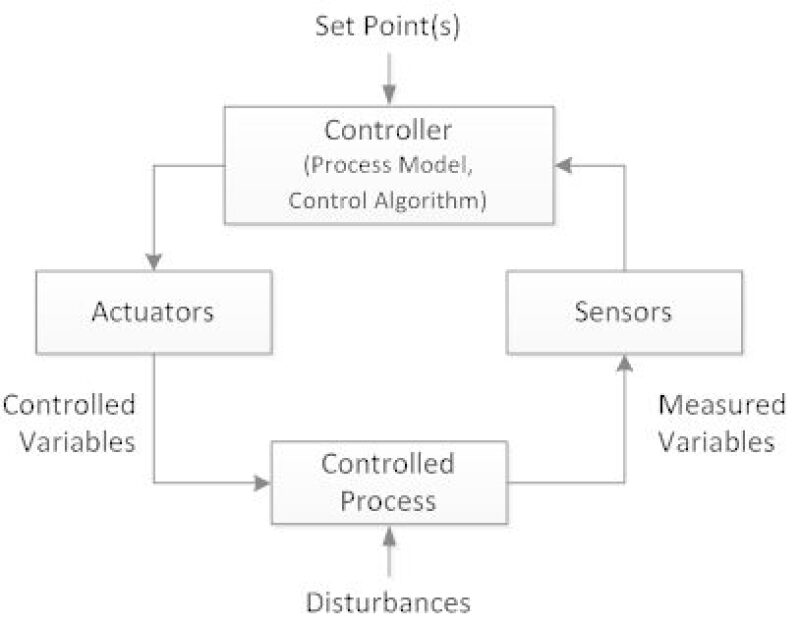
The four required conditions for control are:
Goal condition. The controller must have a goal. For a simple process control loop, the goal is to maintain the set point.
Observability condition. Sensors must exist that measure important variables. These measurements must provide enough data for the controller to observe the condition of the system.
Model condition. The controller must have a model of the system (process model). Data measured by the sensors are used both to update the model and for direct comparison to the goal or set point.
Action condition. The actuator must be able to take the action(s) required to achieve the controller goals.
Role of Mental Models
The controller discussed above may be a human or an automated system. It must contain a model of the system (process model).If the control is a human, he/she must possess a mental model of the system.
The designer’s mental model is different from the operator’s mental model. The operator’s model will be based partly on training and partly on experience. Operators use feedback to update their mental models. Operators with direct control of the process will quickly learn how it behaves and update their mental models.In highly automated systems, operators cannot experiment and learn the system.
Further, in highly automated systems the operator will not always have an accurate assessment of the current situation because his/her situation assessment is not continuously updated.
I have a fishing example of this. I occasionally (but rarely) go fishing in the marshes near Lafitte, Louisiana (south of New Orleans).I don’t know the area well, but I have a map.If I keep track of my movement, I always know where I am and can easily recognize features on the map.If/when I get lazy and just motor around, then I find the map almost useless.I can no longer match geographical features to the map.Every point of land in the marsh looks much like every other point.
Control Algorithm
Whether the controller is human or automated, it contains an algorithm that determines/guides its actions. It is useful to consider the properties of a typical automated loop. Most industrial control loops are PID (proportional-integral-derivative) loops. A PID controller has three functions:
Proportional action. Takes action proportional to the error (difference between the measured variable and the set point); small errors yields minor valve movements; large errors yield large valve movements.
Integral action. Takes action proportional to the integral of the error. Here a small error that has existed for a long time will generate a large valve movement.
Derivative. Takes action proportional to the derivative of the error. A rapidly changing error generates a large valve movement.
Tuning coefficients are provided for each action type. The appropriate tuning coefficients depend on the dynamics of the process being controlled. The process dynamics can be explained pretty well with three properties: process gain, dead time, and lag.
Process gain is the ratio of measured variable change to control valve position change. Lag is a measure of the time it takes the process to get to a new steady state. Dead time is the time between when the valve moves and the process variable begins to change.
Unsafe Control Causes
Control loops are complex and can result in unsafe operation in numerous ways, including: unsafe controller inputs; unsafe control algorithms including inadequately tuned controllers; incorrect process models; inadequate actuators; and inadequate communication and coordination among controllers and decision makers.
STPA—A New Hazard Analysis Technique
The most widely used process hazard analysis technique is the HAZOP. The HAZOP uses guide words related to process conditions (flow, pressure, temperature, and level).
STPA guide words are based on a loss of control rather than physical parameter deviations .(Note that all causes of flow, pressure, temperature, and level deviation can be traced back to control failure.)
The STPA process is as follows:
Identify the potential for inadequate control of the system that could lead to a hazardous state.
A control action required for safety is not provided.
An unsafe control action is provided.
A control action is provided at the wrong time (too early, too late, out of sequence).
A control action is stopped too early or applied too long.
Determine how each potentially hazardous control action could occur.
For each potentially hazardous control action examine the parts of the control loop to see if they could cause it.
Design controls and mitigation measurements if they do not already exist
For multiple controllers of the same component or safety constraint, identify conflicts and potential coordination problems.
Consider how the controls could degrade over time and build in protection such as
Management of change procedures
Performance audits where the assumptions underlying the hazard analysis are such that unplanned changes that violate the safety constraint can be detected
Accident and incident analysis to trace anomalies to the hazard and to the system design.
Safety-Guided Design
Hazard analysis is often done after the major design decisions have been made. STPA can be used in a proactive way to guide design and system development.
The Safety-Guided Process
Try to eliminate the hazard from the conceptual design.
For hazards that cannot be eliminated, identify potential for their control at the system level.
Create a system control structure and assign responsibilities for enforcing safety constraints.
Refine the constraints and design in parallel
Identify potentially hazardous control actions of each system component and restate the hazard control actions as component design constraints.
Determine factors that could lead to a violation of the safety constraints.
Augment the basic design to eliminate potentially unsafe control actions or behaviors.
Iterate over the process (perform STPA steps 1 and 2) on the new augmented design until all hazardous scenarios have been eliminated, mitigated, or controlled.
An example of a safety-guided process is the thermal tile processing system for the Space Shuttle. Heat resistant tiles of various types covered the shuttle. The lower surfaces were covered with silica tiles. They were 95% air, capable of absorbing water, and had to be waterproofed. The task was accomplished by injecting a hazardous chemical DMES (dimethylethoxysilane) into each tile. Workers wore heavy suits and respirators. The tiles also had to be inspected for scratches, cracks, gouges, discoloring, and erosion.
This section is a partial/truncated application of Safety Guided Design to the design of a robot for tile inspection and waterproofing.
Safety-guided design starts with identifying the high-level goals:
Inspect the tiles for damage caused by launch, reentry. and transport.
Apply waterproofing chemical to the tiles.
Next, identify the environmental constraints:
Work areas can be very crowded.
With the exception of jack stands holding up the shuttle, the floor space is clear.
Entry door is 42-in. wide.
Structural beams are as low as 1.75 m.
Tiles are at 2.9- to 4-m elevation.
Robot must negotiate the crowded space.
Other constraints:
Must not negatively impact the launch schedule.
Maintenance cost must be less than x.
To get started, a general system architecture must be selected. Let’s assume that a mobile base with a manipulator arm is selected. Since many hazards will be associated with robot movement, a human operator is selected to control robot movement and an automated control system will control nonmovement activities.
The design has two controllers, so coordination problems will have to be considered.
Step 1: Identify potentially hazardous control actions.
Hazard 1—Robot becomes unstable. Potential solution 1 is to make the base heavy enough to prevent instability. This is rejected because the heavy base will increase the damage if/when the robot runs into something. Potential solution 2 is to make the base wide. This is rejected because it violates the environmental constraints on space. Potential solution 3 is to use lateral stabilizer legs.
However, the stabilizer legs generate additional hazards that must be translated into design constraints such as the leg controller must ensure that the legs are fully extended before the arm movements are enabled; the leg controller must not command a retraction unless the stabilizer arm is in the fully stowed position; and the leg controller must not stop leg extension until they are fully extended.
These constraints may be enforced by physical interlocks or human procedures.
Summary and Conclusion
Leveson argues that our standard accident model does not adequately capture the complexity of our projects. Her proposed solution sensibly addresses the flaws that she has noted.
Viewing safety as a control problem resonates with me. All or almost all of the hazard causes that we discover in HAZOPs are control system-related, yet the HAZOP method does not focus explicitly on control systems. And control between levels of the hierarchy is generally not considered at all in process hazard analyses.
I am particularly attracted to the ability to apply STPA during project design, as opposed to other process hazard analysis techniques that can only be applied to a ‘completed’ design.
References
Leveson, N. 2012. Engineering a Safer World, Systems Thinking Applied to Safety. MIT Press.
Mitchell, M. 2009. Complexity, A Guided Tour. Oxford University Press.
Howard Duhon is the systems engineering manager at GATE and the former SPE technical director of Projects, Facilities, and Construction. He is a member of the Editorial Board of Oil and Gas Facilities. He may be reached at hduhon@gateinc.com.