The aim of this work is to present the effectiveness of a fully integrated approach for ensemble-based history matching on a complex real-field application. The predictive ability of the ensemble of models is greatly enhanced through an integrated work flow promoting collaboration between all subsurface disciplines. One key feature of the ensemble-based method that is especially important for complex reservoirs is that it overcomes the typical limitation of the traditional approaches where the number of uncertainty parameters resulting from practical or algorithm constraints often has to be reduced.
Methodology
The distinguishing feature of ensemble-based methodologies is their capability of integrating multiple sources of data while quantifying and propagating the uncertainty in reservoir-model parameters. In order to achieve a proper uncertainty assessment, one must couple the ensemble-based data-assimilation technique with an integrated work flow covering the entire reservoir-modeling process.
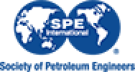