Digitization of the Oil Industry
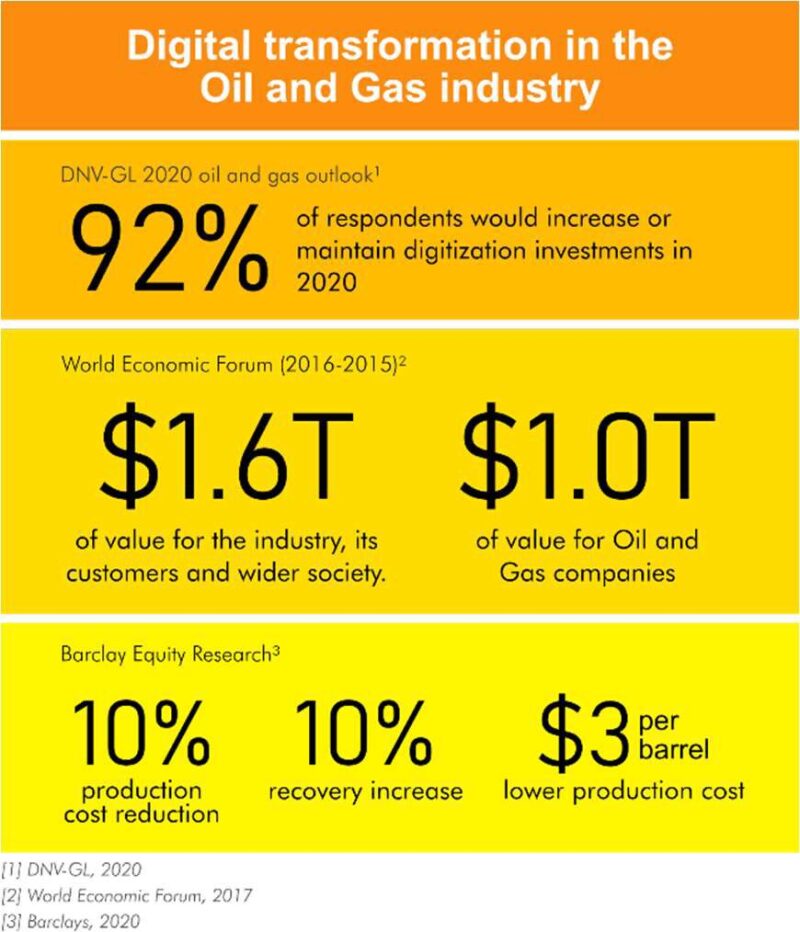
Oil and gas companies are awash with data from many different disciplines. With the digitization of the oil and gas industry and the Internet of things, where digital sensors are placed everywhere throughout the value chain, the amount of data grows exponentially. Bill Braun, the chief information officer for Chevron, has estimated that the amount of data collected will double every 12 to 18 months (Crooks 2018).
As an example, the oil and gas industry only uses about 5% of the seismic data it has available for exploration activities. Furthermore, it has been reported that engineers and geoscientists spend more than half of their time searching for and assembling data (Brulé 2015).
Various reports, therefore, predicted substantial cost savings and increases in hydrocarbon production as a result of successful digital transformation of the oil and gas industry where data is used much better and faster.
The large volume of data in the oil and gas industry, both structured and unstructured, that inundates the business daily is referred to as “big data.”
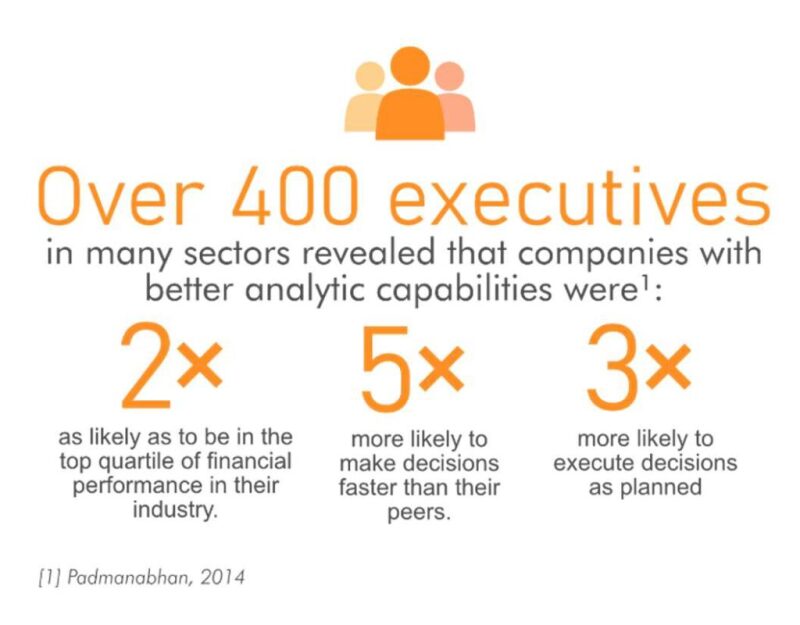
The key attributes of the structured data are that it is well organized, fully digital, labeled, and voluminous. Numerous initiatives have been taken to deploy artificial-intelligence and machine-learning solutions to add value and extract knowledge. Many of these efforts have been successful because the supervised-learning technologies used require exact described attributes of the structure data.
Less focus, however, has been placed on unstructured data represented by text and images in reports, presentations, and spreadsheets; more than 100 file formats exist. Engineers and geoscientists struggle to work with these massive amounts of unstructured, yet valuable, data because they are not organized, are difficult to manage, and may not be complete. New data added to an existing unstructured database is lost collectively to the organization almost immediately because it relies on individual experience, thereby compounding the problem.
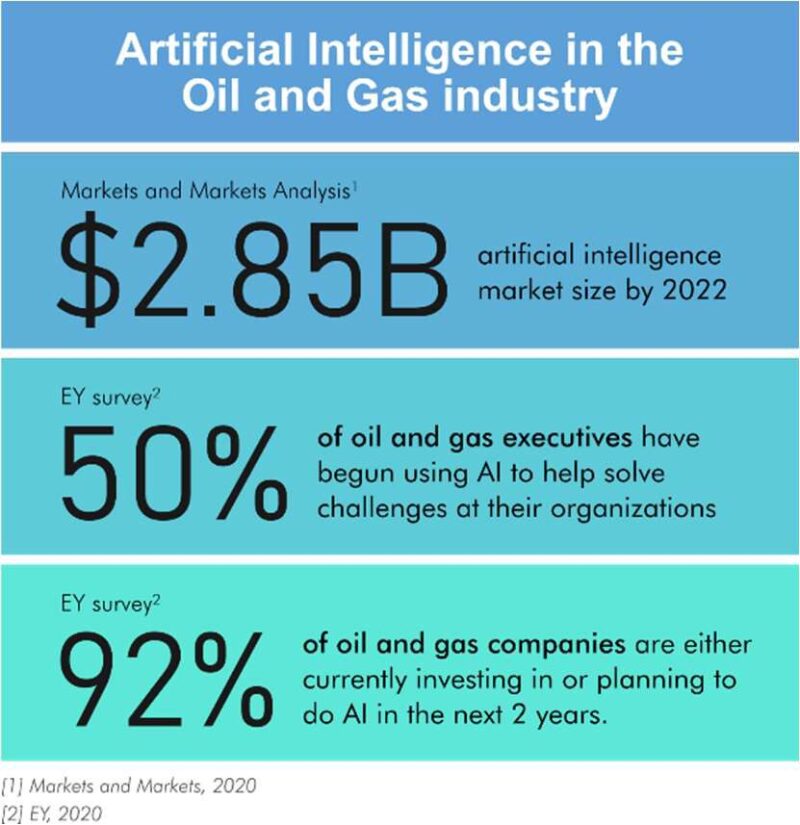
Similar supervised technologies are more difficult to apply directly to unstructured data because of a lack of the attributes of the structured data. With the development of new work flows and alternative applications, unstructured data now also can be organized successfully. This innovation can support oil and gas companies in analyzing the large quantities of unstructured data from disparate sources and generating real-time insights (World Economic Forum 2017).
Processing of Unstructured Data
Initial processing of unstructured data has been focused on digitization, data storage, and simple search functions to make use of this “dark” data, which is not part of the oil and gas in-depth work flow. By using advances in supervised/unsupervised machine learning and cloud computing, it is now possible to organize the data further, extract knowledge, and integrate it into existing work flows.
Digitized unstructured data are ingested through a pipeline with work flows using machine-learning techniques such as natural-language processing (NLP) or deep convolutional neural network to provide a structured data set by tagging text and images.
A work flow for automatically extracting information from the documents consists of a set of algorithms to identify blocks/segments within a document, after which supervised machine learning is used to classify the document segments as either text or nontext.
Optical character recognition can be applied to the text segments to convert them into editable text, which then is analyzed further using NLP and sentence analysis. In a separate data pipeline, the nontext components, such as images and tables, can be tagged using convolutional neural networks (Maver et al. 2020).

The aim is to free the unstructured data, to make the information accessible, understandable, and actionable across business units, departments, and geographies, which increasingly is a requirement as data expands in both volume and complexity (Buchholz et al. 2018).
Improving the Decision-Making Process
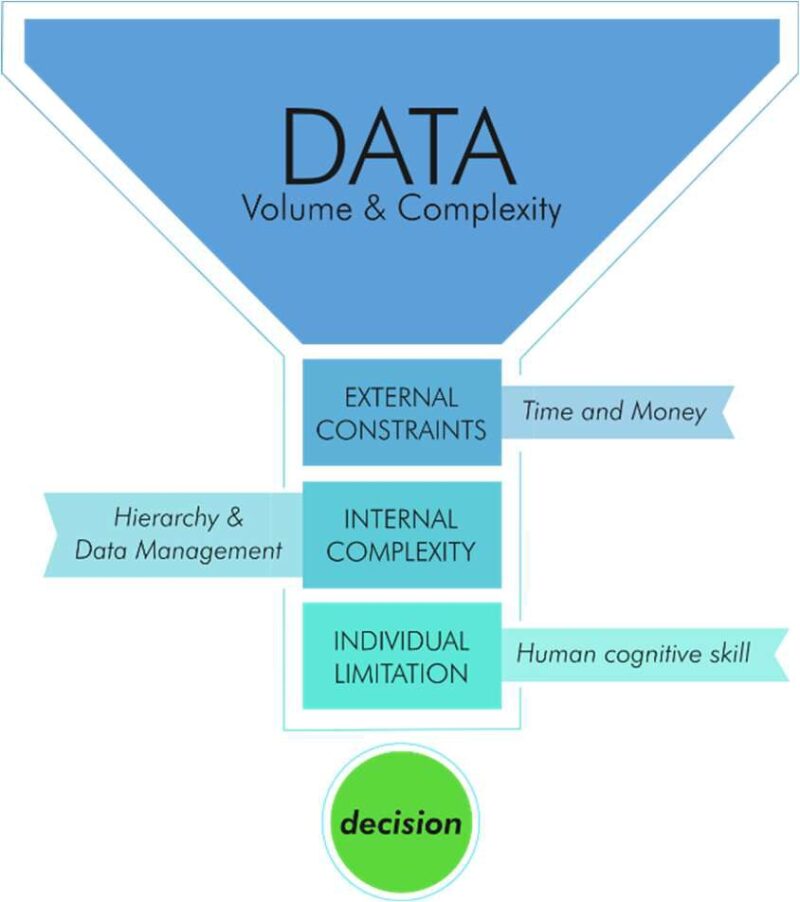
Data is only important if it leads to decisions within an organization that have a business impact.
Within a data-to-impact process are three limiting factors for adequate data decisions to be taken (Baillard 2020):
- External constraints such as time or money
- Internal complexity within an organization
- Individual limitations related to human cognitive skills
The outcome of processing the unstructured data is to overcome these limiting factors. As a result, the following value can be realized.
Data Management. By ingesting both historic and future unstructured and structured data and making the data accessible through a single web-enabled interface, any data can instantly be identified, located, and retrieved through a text and image search.
This makes it possible to continuously organize new data being produced. Consequently, all data can be used in decision making. The access to data is seamless across teams and subject-matter areas supporting cross-company collaboration and a better overview.
Today, organization often are tempted to buy, acquire, or record new data to improve the decision-making process. This has been particularly true in recent years with the emergence of big data and data science, which can overwhelm the decision makers but which now can be reduced by organizing the unstructured data (Baillard 2020).
Decision Making. The following tools have been developed to ensure that relevant information can be retrieved when required, which can improve decision making:
- Intelligent full text search capabilities of the text corpus and images with a link to the original data point (e.g., report)
- Structuring of all images for better overview and comparison
- Tables converted to CSV files for easy data retrieval
- Non-English translation functionality for easier information digestion and full search capabilities in English
Advances in NLP enable the translation to work anywhere and in any language. An explorationist can go to South America or Africa in search of hydrocarbons, mine through troves of engineering and geoscience documents written in Spanish or French, and get real-time machine-aided translations in English. This is breaking down language barriers and opening doors for new opportunities in foreign countries.
Improved decisions are made because all data can be located, and the decisions can be made faster, estimated to be up to 40 times faster than manually reading and reviewing the data (Baillard et al. 2019).
New Knowledge
Unstructured big data is difficult or even impossible to access to manually extract new complex geoscience, engineering relationships, and knowledge.
When data analytics is applied to the organized data, higher-order analysis can be performed. For example, a knowledge graph can make a 50-year drilling history of more than 150 wells instantly available, the geodensity of information promptly accessible, and microscopic analysis immediately available from all wells in an area (Maver et al. 2020).
Future Benefits
In comparison to other sectors, the oil and gas industry’s approach to the digital transformation is expected to be evolutionary rather than revolutionary. However, developments in technologies such as the cloud, big data, and data analytics are driving trends that have immense potential for the oil and gas industry (World Economic Forum 2017).
The solutions now available for organizing unstructured data have the ability to manage the four V’s in big data: volume, variety, velocity, and veracity. A substantial volume of unstructured data can be organized from a large variety of document types, all available instantaneously (with a high velocity) and with a high degree of accuracy (high veracity).
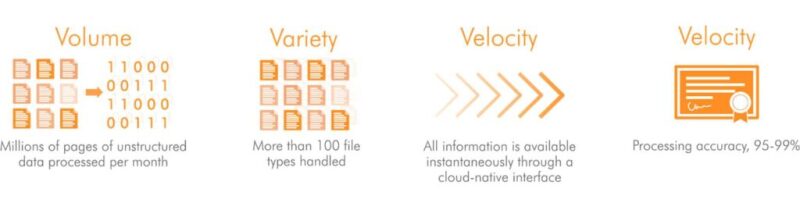
Data siloes, in particularly, are an issue in the oil and gas industry, where local storage of data and long, complex value chains mean individual businesses or even units within a business may lack a holistic view of the data they need to improve their operations (Williams and Strier 2020). With the consolidated unstructured and structured data instantly accessible across the organization and value chain, the data siloes can be demolished.
New data will only be required to enhance the existing old data (now structured) by filling the voids. Management, therefore, will benefit from better decision making when acquiring new data because it will have a more-complete overview of the current old data present within the organization (Baillard 2020).
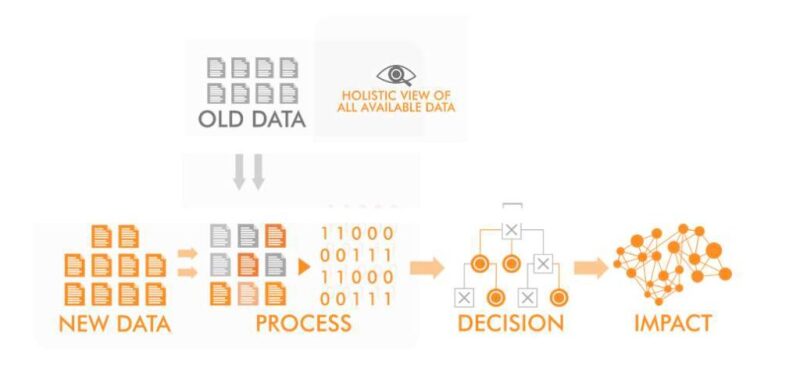
Exploration and production decisions become easier and faster with the availability of information and the ability to rank opportunities and portfolios on the basis of technical and economic metrics. In a well-organized pipeline with accurate quality-control and quality-assurance processes, millions of documents can be processed per month.
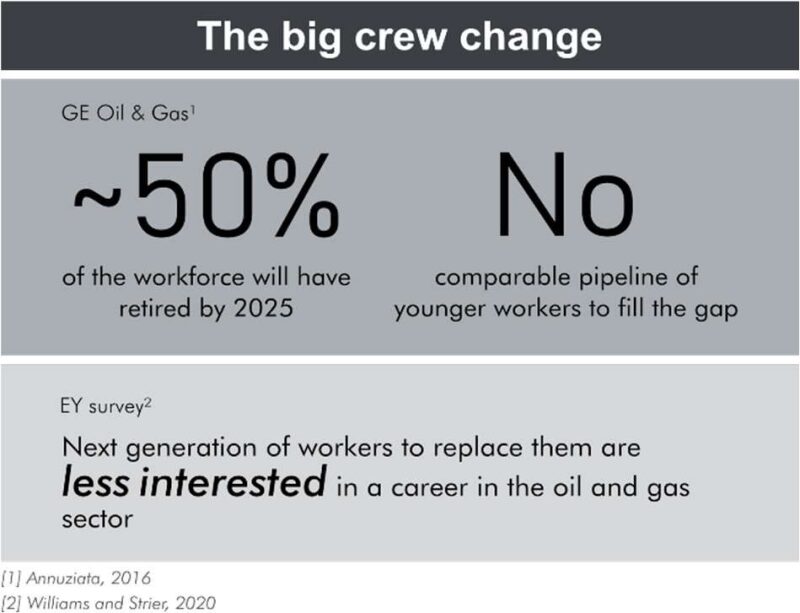
The main bottleneck in the process becomes verifying and making the documents available in a timely manner for the process.
With the continued aggregation and ingestion of more unstructured data, machine-learning processes will improve, resulting in better data analytics providing a continuous exponential knowledge explosion across geography, organization, and disciplines.
The big crew change is sweeping over the oil and gas industry, mergers and acquisition are changing the industry landscape, and staff changes because of fluctuations in the business environment are making capturing and maintaining corporate knowledge a significant issue. This challenge can be managed much better by having a process to make unstructured data easily captured, organized, and accessible in a central cloud-native repository. In the competition for exploration and production opportunities, those oil and gas companies embracing digitization will gain enormous commercial advantages. Those that don’t face big challenges ahead.
References
Anderson, J.D., Lenschow, R., Rainforth, L., et al. 2020. North America Oilfield Services and Equipment. Frac to the Future; Oil’s Digital Rebirth. Barclays, London, England (15 January).
Annunziata, M., 2016. Digital Future of Oil and Gas and Energy. GE Oil and Gas, London, England.
Baillard, F., 2020. Do You Always Need New Data? Medium, 15 May, https://medium.com/@fb_88498/do-you-always-need-new-data-96d4c0364a (accessed 15 June 2020).
Baillard, F., Maver, K.G. and Hernandez, N.M., 2019. A New Way of Handling Unstructured Data in the Age of Digitalization. EAGE Subsurface Intelligence Workshop, Manama, Bahrain, 8–9 December. https://doi.org/10.3997/2214-4609.2019X610103.
Brulé, M.R., 2015. The Data Reservoir: How Big Data Technologies Advance Data Management and Analytics in E&P. SPE Digital Energy Conference and Exhibition, The Woodlands, Texas, USA, 3–5 March. SPE-173445-MS. https://doi.org/10.2118/173445-MS.
Buchholz, S., Briggs, B., et al. 2018. Insights, Tech Trends for the Oil and Gas Industry. Deloitte, www.deloitte.com/insights/tech-trends (accessed 15 June2020).
Crooks, E., 2018. Drillers Turn to Big Data in the Hunt for More, Cheaper Oil. Financial Times, 12 February, https://www.ft.com/content/19234982-0cbb-11e8-8eb7-42f857ea9f09.
DNV GL, 2020. New Directions, Complex Choices: The Outlook for the Oil and Gas Industry in 2020. DNV GL, Høvik, Norway.
Economist, 2017. Data Drilling: Oil Struggles To Enter the Digital Age. The Economist, 6 April, https://www.economist.com/business/2017/04/06/oil-struggles-to-enter-the-digital-age (accessed 15 June 2020).
EY, 2020. Applying AI in Oil and Gas. EY, https://www.ey.com/en_jo/applying-ai-in-oil-and-gas (accessed 15 June 2020).
Markets and Markets, 2020: AI in the Oil and Gas Market. Markets and Markets, https://www.marketsandmarkets.com/Market-Reports/artificial-intelligence-oil-gas-market-87246288.html.
Maver, K.G., Hernandez, N.M, Baillard, F., and Cooper, R., 2020. Processing of Unstructured Geoscience and Engineering Information for Instant Access and Extraction of New Knowledge. First Break 38 (6): 59–64. https://doi.org/10.3997/1365-2397.fb2020044.
Padmanabhan, V., 2014. Big Data Analytics in Oil and Gas: Converting the Promise Into Value. Bain and Company, Dallas, Texas (26 March).
Williams, J., and Strier, K., 2019. Is AI the Fuel Oil and Gas Needs? EY, 8 January, https://www.ey.com/en_jo/oil-gas/is-ai-the-fuel-oil-and-gas-needs (accessed 15 June 2020).
World Economic Forum, 2017. Digital Transformation Initiative: Oil and Gas Industry. World Economic Forum, Geneva, Switzerland (January 2017).
Kim Gunn Maver, SPE, is the executive vice president for Iraya Energies. He has 30 years of experience in the oil and gas service industry, holding senior business development and management positions with Ødegaard, WesternGeco, Schlumberger, RXT, and Spectrum. Maver holds an MBA degree from Copenhagen Business School and a PhD degree in geology from Copenhagen University.
Charmyne Mamador is the head of product design for Iraya Energies. She has a passion for developing beautiful and functional interfaces, particularly for statistical and spatial data displays. Mamador holds an MS degree in energy engineering.
Nina Marie Hernandez, SPE, is the founder and chief executive officer of Iraya Energies. She has international experience working in various technical, sales, and business-development roles, including working as Asia business development manager for Schlumberger in the petrotechnical consulting group. Hernandez holds an MS degree in petroleum engineering from the Technical University of Denmark.