In the past several decades, technology continuously pushed the technical limit of extended-reach and complex wells, bringing records for lateral length, depth, and number of laterals. In the past few years, however, the design and operations of extended-reach and complex wells started to evolve under the volatile oil market pricing conditions and the stride of digital transformation. The technology front is shifting from marching toward longer and more-complex wells to smarter wells with better return on investment (ROI).
Artificial intelligence (AI) and machine learning, which can effectively mine deep insight from a large amount of drilling operational data and synthetic forward modeling data, are starting to shape the design and operations of extended-reach and complex wells. AI and machine learning have been demonstrating their ability to provide early warning of stuck pipe, reveal root causes of drilling accidents, improve drilling design, optimize completion design, and find new ways to improve production performance.
Rather than competing on going even farther, U-shape (or “horseshoe”) wells have been drilled to tap shale gas through leveraging state-of-the-art extended-reach drilling technology and stimulation technology to improve the economics of the development of unconventional reservoirs.
Looking to the future, the march toward longer extended-reach wells and more-complex multilateral wells to access formidable reservoirs will continue. The great potential, however, lies in the application of state-of-the-art extended-reach and complex well technology to versatile oil and gas fields (both conventional and unconventional) for the purpose of further improving ROI of the project, and AI and machine learning will play a vital role in enabling a step change in design and operations.
This Month’s Technical Papers
Machine Learning Aids Early Detection of Stuck Pipe in Extended-Reach Wells
Data-Driven Approach Detects, Classifies Screenout in Horizontal Wells
Modeling, Planning Allow Successful Implementation of South Texas U-Turn Lateral
Recommended Additional Reading
SPE 202046 Automated Geosteering While Drilling Using Machine Learning—Case Studies by Ivan Denisenko Denisenko, Rogii, et al.
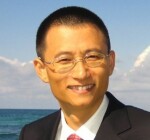
Kaibin Qiu, SPE, is a geomechanics adviser at Schlumberger. He has more than 20 years of experience in the industry and has worked on many geomechanics projects in Malaysia, Iran, Egypt, Libya, India, Indonesia, Japan, and China. Qiu holds a BS degree in hydraulic and hydropower engineering and an MS degree in geotechnical engineering, both from Tsinghua University in China. In recent years, he has been involved in the application of reservoir geomechanics for exploration and development of high-pressure/high-temperature wells, deep water, tight gas, shale gas, methane hydrate, geothermal wells, and gas injection. Beyond geomechanics, Qiu also is specialized in drilling engineering, integrated seismic to stimulation solutions for unconventionals, and carbon capture and storage. He has authored more than 40 technical papers. Qiu is an associate editor and technical review editor for the peer-reviewed SPE Journal and a technical review editor for the peer-reviewed SPE Drilling & Completion journal. He is a member of the JPT Editorial Review Board.