A project to predict how much carbon dioxide injected into an oil field is likely to remain there forever set off the US Department of Energy (DOE) on a search for faster data analysis methods.
Speed matters because making projections going out as far as 1,000 years using a probabilistic model (Monte Carlo) will require long computing times, which are costly. DOE is trying to limit the total time by seeking quicker, lower-cost ways to generate the required data inputs by finding reasonably accurate methods that are faster than multiple processing runs of a full-field reservoir simulation model.
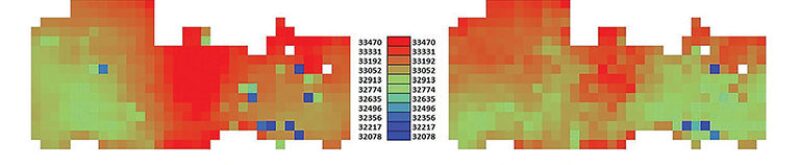
A paper (SPE 173406) presented at the SPE Digital Energy Conference and Exhibition in The Woodlands, Texas, reported on a faster method for analyzing the impact of alternating injections of carbon dioxide and water into a large west Texas oil field. The project is focused on SACROC, a west Texas oil field with a lot of available data, because it is one of the oldest enhanced oil recovery (EOR) projects using carbon dioxide.
While EOR using carbon dioxide is a far more economically attractive long-term storage possibility than alternatives that treat gas as a waste product, there is little information on whether the gas can be permanently stored that way.
The test focused on projecting pressure, gas concentration (mole fraction), and water saturation levels over the top horizon in the huge formation, known as Layer 18. The goal was to do that work significantly faster than a standard reservoir simulator.
“Using a pattern recognition-based technology addresses many time-consuming tasks performed by reservoir simulation models,” said Alireza Shahkarami, an assistant professor of petroleum and natural gas engineering at St. Francis University, who presented the paper. “It requires few simulation runs to train these artificial intelligence-based models compared to a 3D reservoir model, which requires hundreds to thousands.”
After it had been “trained” to recognize patterns in the data, the predictive model, known as a surrogate reservoir model, did calculations in seconds. The same work would have taken about 24 hours using a numerical simulator, because the predictive model can reduce its calculations to a few simulation runs while a full reservoir simulation would require hundreds of them.
Training the neural network model to do lightning fast predictions took more than a week, with most of that time required to create the database that the computer used for training—essentially seeking out patterns in the data. The training stage required about 24 hours. Setting up a numerical simulator would have required far longer, Shahkarami said.
The results of the surrogate reservoir were tested by comparing its predictions to data in sections of the reservoir which were not inputted into the surrogate reservoir model. The error rate was less than 5% in sections of the reservoir where data had been withheld, Shahkarami said. As for the reaction from the teams that manage the project, he said, “They were surprised. They had doubts about the process.”