Because of inherent complexities, understanding the characteristics of perforations in downhole environments is a significant challenge. Perforation-flow laboratories have been used to provide insight into cleanup and productivity mechanisms around perforation tunnels. In contrast to previous studies, the model presented in this work uses the perforation-flow laboratory, micro-computed-tomography (CT) and conventional CT imaging, and, most importantly, an advanced simulation approach to provide an accurate assessment of cleanup techniques and productivity. This full-scale 3D flow model accounts for realistic aspects of tunnel geometry, perforation damage, and blockages that impede flow.
Introduction
In recent years, numerical tools increasingly have been used in conjunction with experiments to provide better insight into the flow characteristics of perforated cores and perforated well-scale formations.
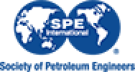