The authors present a geology-driven deep-learning estimated-ultimate-recovery (EUR)-prediction model for multistage hydraulically fractured horizontal wells in tight gas and oil reservoirs. The novel approach was made possible by recent developments in the field of deep learning and by the use of big data (more than 200,000 geological data points and more than 800 wells). A deep neural network (DNN) was trained to learn the relationship between geology and the average EUR (estimated by decline analysis).
Introduction
Currently, the consensus in the literature and among geologists is that it is impossible to achieve a good hydrocarbon-in-place estimate for tight or unconventional resources by entering geological parameters into an equation. Volumetric analysis, for instance, gives only a very crude estimate and often does not work at all. This consensus is not wrong, and yet highly skilled teams of geologists and exploration engineers manage to make good guesses as to where the sweet spots are, even in regions where they have barely adequate geological data.
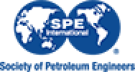