In the complete paper, the authors reduce nonuniqueness and ensure physically feasible results in multiwell deconvolution by incorporating constraints and knowledge to methodology already established in the literature. The paper demonstrates that a combination of constraints on the shapes of the deconvolved derivatives and knowledge of the reservoir results in an improved level of quality and consistency in multiwell deconvolution solutions. The new constrained least-squares multiwell deconvolution approach is illustrated by synthetic examples with known solutions involving up to nine wells.
Introduction
To apply multiwell deconvolution to real data, confidence must exist in the validity of the solution it provides and the consequent interpretation that is inferred. Therefore, for practical multiwell deconvolution of field data, reducing the nonuniqueness in a solution, ensuring the results are physically feasible, and providing the desired consistency and quality of solutions are essential.
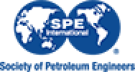