Reliable reservoir models require that all available data is captured in a consistent manner. Furthermore, reliable models need to incorporate the cross-discipline knowledge of the subsurface team and accurately describe the reservoir physics. All the while, the models must account for uncertainty.
With a traditional approach to reservoir modeling, a subsurface team can spend months or years to generate a single model—a base-case model that will often violate all the criteria outlined above and have disappointing predictive capabilities (Thomas 1998). Consequently, reservoir management decisions are often suboptimal, with the asset potential not fully realized.
Reservoir Modeling Challenges
One of the largest challenges in reservoir modeling is the consistent integration of static and dynamic data. This is especially true when modeling is done in a step-wise manner, treating static and dynamic data conditioning (also known as history matching) as separate tasks.
Understandably, this can limit the interaction between the different subsurface disciplines during model generation and history matching. Thus, the resulting models might perfectly match the current dynamic data measurements but completely fail to honor the input (static) data and the geological concept.
A typical example could be a porosity field that has been multiplied by a factor of five in a 500 × 500 meter box around a single well, an artificial modification introduced to match the water cut during dynamic data conditioning. This increase in porosity might be justifiable under a different geological concept. However, a lack of efficient tools and workflows makes it extremely time-consuming to update these models. As a result, the desired feedback loop in the modeling process is not achieved as model predictions typically must be delivered within a deadline.
Uncertainty quantification is another great reservoir modeling challenge. Uncertainty studies are often conducted in the static data domain, ignoring the information found in available dynamic data. Alternatively, rejection sampling techniques are used as ad hoc solutions to incorporate the dynamic data, where models that do not adequately match the observed data are removed from the uncertainty study.
Hence—despite hundreds or thousands of simulations having been run—a probabilistic forecast of reserves or water breakthrough time is often severely biased with a narrow range, as key uncertainties or data are overlooked. For these reasons, many subsurface teams revert to the rule of thumb, using a base-case prediction plus or minus 20% to 30% to represent the reservoir uncertainty. Failure to capture and propagate uncertainty in the modeling process often results in an increased risk of suboptimal decision-making.
Integrated Models Honor All Data
Resoptima has developed ResX, a reservoir modeling and data conditioning software tool that efficiently meets the challenges describe above. Rather than generating a single, base-case reservoir model, it uses repeatable workflows to generate an ensemble of models that are equally plausible and consistently honor all available data.
During the dynamic data conditioning process, the software tool updates the very same parameters that were used to describe the model; 2-D surfaces used as input to the structural framework; 3-D properties describing the facies distribution and the petrophysical properties; scalar variables describing the relative permeability curves; and other uncertain parameters defining the model.
Hence, the reservoir modeling and data conditioning efforts are carried out in a single step, making multidisciplinary collaboration a natural part of the process and avoiding the introduction of model inconsistencies.
Furthermore, by capturing and propagating uncertainty in all parts of the modeling process (including the static and dynamic input data), ResX can bring an improved understanding of the reservoir to the asset team that can help it to manage decision-making risks more efficiently.
In the dynamic data conditioning phase, the tool combines big-data analytics with the subsurface team’s knowledge to update the full ensemble of reservoir models. Because every model is equally probable given the current static data measurement, none of the models are thrown away during history matching.
Instead, the parameters used in the model-building process are directly updated—with the data honored and the changes to the input models minimized. This ensures that the model uncertainty is not reduced unless the data measurements strongly support doing so, which reduces the risk of overfitting the models to the current data (e.g., Silver 2012).
Case Study: Goliat Field
The Goliat field, operated by Eni in the Barents Sea offshore Norway, consists of eight subsea templates with 32 well slots that are tied back to a cylindrical floating production, storage, and offloading vessel (Fig. 1, at the top of the article).
The field produces from two reservoirs, the Realgrunnen group and the Kobbe formation at depths of 1100 m and 1800 m, respectively. The reservoirs both contain oil with an overlying gas cap and have hydrostatic pressures of 120 bar and 190 bar, respectively.
Reservoir production employs water injection for pressure support and gas is reinjected into both formations. Field production began in March 2016, and 12 oil producers, three gas injectors and seven water injectors are now on stream.
Using the ResX tool, integrated reservoir modeling workflows were established for the Kobbe and Realgrunnen reservoirs as illustrated in Fig. 2. The variabilities in the static data interpretation, geological concepts, facies modeling description, petrophysical modeling, and other factors were incorporated, capturing and propagating uncertainty in a modeling process that is repeatable and updatable when new data arrives.
Despite the geological differences between the two reservoirs, the building blocks for the integrated modeling workflows were identical, according to the software tool’s design.
Throughout the project, the model received multiple updates to account for new production data, e.g., by adjusting the geological concepts and evaluating the approach used for modeling the vertical permeability.
Importantly, the modeling framework remains unchanged, despite fundamental changes made to some of the modeling process building blocks (when new data contradict previous model assumptions). This makes model updating highly efficient.
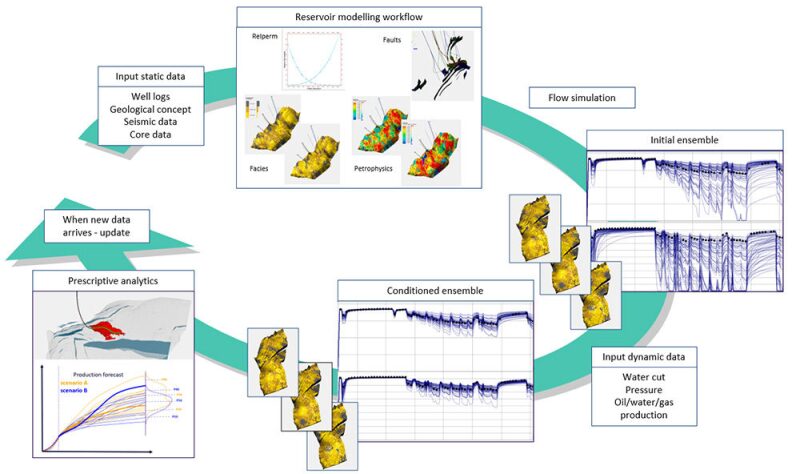
The business objective of the study was to identify potential infill well targets in the two reservoirs. To accomplish this, the ensemble of generated models was used as input to a prescriptive analytical tool to automatically identify bypassed areas and evaluate potential infill targets.
The ResX software confirmed the location of previously identified targets and, more importantly, allowed for the identification of several new locations that are suitable candidates for infill drilling. Having the ability to quickly identify opportunities and manage the risks in reservoir management decisions is crucial to the goal of increasing productivity and efficiency through the life of the field.
The Way Forward
The amount of data a subsurface team must manage in modeling a reservoir modeling is steadily increasing. Having the ability to quickly convert the data into usable, valuable information and generate reliable models enables vital input for field reservoir management.
The ensemble-based modeling approach at the Goliat field demonstrates how computer power, data, and subsurface team expertise can be brought together to improve reservoir understanding in a fraction of the time needed with traditional tools and approaches.
How fast reservoir models can be updated is an open question. The ambition should always be to update models when new data arrive rather than waiting for months or years, as they sit in a database, before they are used in the model. With the continuous increase in computational power, the ability to generate, simulate, and update models in real time when the data arrive should no longer seem a blue-sky dream. It should soon be reality.
Acknowledgements
The authors would like to thank the Goliat licensees, Eni and Statoil, for permission to use the Goliat field as a case study. The authors additionally express thanks for their helping role in this article to Guro Solberg, Lars-Erik Gustafsson, Knut Ingvar Nilsen, and Audun Fykse, of Eni; and Eirik Morell and TC Sandø of Resoptima.
References
Silver, N. 2012. The Signal and the Noise: Why So Many Predictions Fail—but Some Don’t, First edition, Chapter 1, pp. 19–46. New York, New York: Penguin Press.
Thomas, J. M. 1998. Estimation of Ultimate Recovery for UK Oil Fields; The Results of the DTI Questionnaire and a Historical Analysis. Petroleum Geoscience, 4 (2): 157–163. doi: 10.1144/petgeo.4.2.157