Over the last couple of decades, we have seen a steady stream of “intelligent” innovations go from ideas to infancy to catalog solutions. Advances in metering, measurement, control, automation, and modeling have changed our daily routine such that our decisions are better informed, and, as a result, we produce in more-challenging environments, at lower cost, and with better recoveries.
Whether deployed in the office or in the field, the majority of these advances have pertained to improvements in our organizations, in our production facilities, and in our wells. While still not routine, tools based on artificial intelligence, large-scale model integration, and automated data/model integration are finding their way into the typical practitioner’s toolbox. What is clearly emerging now are practical solutions that take the next step into the reservoir and across solution types and discipline boundaries. In the coming years, artificial-intelligence applications will steer our exploration and appraisal processes. While we push model integration (e.g., between static and dynamic and for entire production systems), artificial intelligence will be working behind the scenes to find model inconsistencies, to narrow uncertainties, and to improve our forecasts and option assessments.
These advances are coming at an opportune time in that they can support better decision making in some of our most-costly decisions (e.g., helping us to improve our productive drilling rates and demonstrating the real costs of deviating from reservoir-management plans). The papers highlighted in this section provide clear illustrations of how some of this will be achieved.
This Month's Technical Papers
Geology-Driven Estimated-Ultimate-Recovery Prediction With Deep Learning
Machine Learning Applied to Multiwell-Test Analysis and Flow-Rate Reconstruction
Flow-Control Optimization Maximizes Accuracy of Multiphase-Flow Rate Allocation
Recommended Additional Reading
OTC 26209 Proactive and Reactive Strategies for Optimal Operational Design: An Application in Smart Wells by A.C.A. Abreu, PUC-Rio, et al.
SPE 176760 Leveraging Intelligent-Field Data for Full-Field Pressure-Transient Analysis by B. Al-Wehaibi, Saudi Aramco, et al.
SPE 177829 Smart Proxy: An Innovative Reservoir-Management Tool; Case Study of a Giant Mature Oil Field in the UAE by S.D. Mohaghegh, Intelligent Solutions, et al.
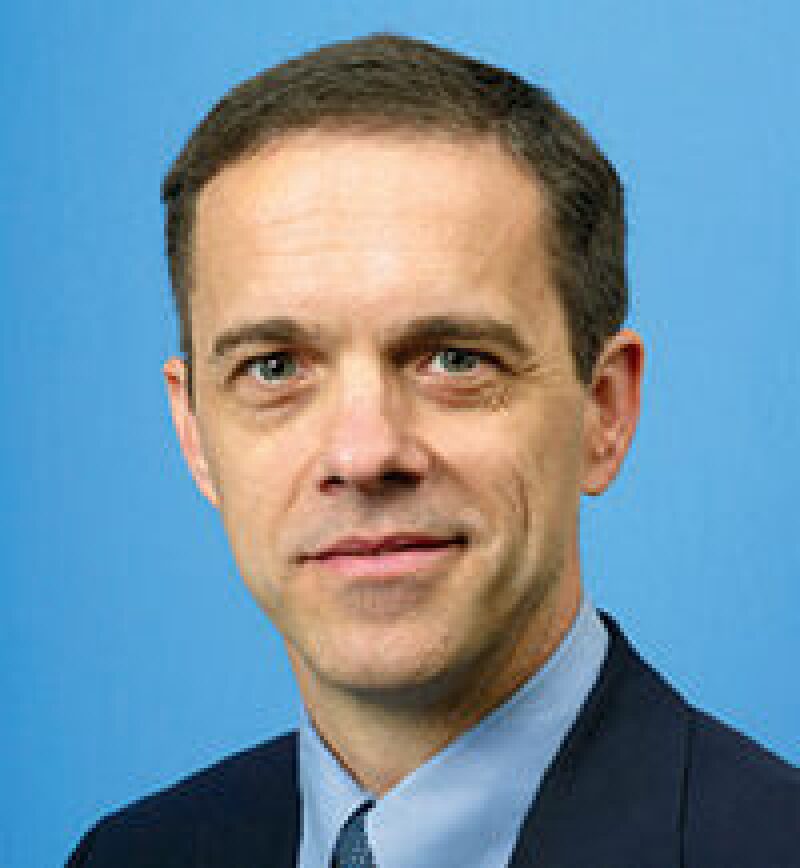
John Hudson, SPE, is a principal production engineer with Shell. He has more than 25 years of experience in multiphase-flow research, flow-assurance design of deepwater production systems, and development of model-based real-time operations decision systems. Since joining Shell, Hudson has held technical and managerial positions in Europe and North America, including leading a team that developed a model-based cloud computing solution that was deployed globally to gas plants with a total production capacity in excess of 10 Bcf/D. He currently provides production-engineering support for the development of a next-generation simulator. Hudson holds a PhD degree in chemical engineering from the University of Illinois. He serves on the JPT Editorial Committee and can be reached at www.linkedin.com/in/hudsonjohnd.