In the late 1990s, a friend in the office showed me a cobbled-together neural network in Excel that was driving (then Hyprotech, now Aspen) Hysys such that the calculation speeds were significantly faster than those in the more-rigorous but wandering solution that Hysys alone could yield. While interesting and a good solution for that problem, it seemed to me at the time something that a hobbyist would do in the evenings.
During the past few years, it has become painfully obvious that such a dismissive conclusion is outdated. Artificial-intelligence (AI) -based methods have become mainstream engineering, and we as practitioners need to have a firm understanding of the principles and be ready to apply them when the opportunities arise.
AI-related papers now make up a significant fraction of the overall literature in intelligent fields. For example, during the past year, Ikezue and Onukwuli have shown how neural networks can be used to control gas production in multilaterals. Baarimah et al. used a variety of techniques, including neural networks and fuzzy logic, to predict reservoir-fluid properties and demonstrated why this could be a better approach to a single correlation choice. Alzate et al. used neural networks to generate and test artificial well logs to assist in geomechanical evaluations. Note that these papers and others presented in the last year come not only from academic institutions but increasingly from operators and service providers reporting how these techniques are being used to make business decisions.
Why have these technologies gained traction? The answer, of course, is partly that the technologies have matured, though the basics have clearly been in place for some time. The more important trends are a vast increase in the availability of data and the increasing need to make business decisions before the physics of the problem is understood well enough to form a reasonable physical model. AI has emerged as a go-to solution type to help us build physical insight into problems where the complexity or heterogeneity of the problem makes a comprehensive physical model still too difficult to pose.
Among the articles featured in the section, you will find some enlightening examples that illustrate the utility of these approaches.
Recommended Additional Reading
OTC 25391 A Review of Intelligent-Completions Installations: Lessons Learned From Electric/Hydraulic, Hydraulic, and All-Electric Systems by Maciel Potiani, Baker Hughes, et al.
SPE 169388 Generating Synthetic Well Logs by Artificial Neural Networks Using MISO-ARMAX Model in Cupiagua Field by G.A. Alzate, Universidad Nacional de Colombia, et al.
SPE 170113 An Integrated Application of Cluster Analysis and Artificial Neural Networks for SAGD-Recovery-Performance Prediction in Heterogeneous Reservoirs by Amirian Ehsan, University of Alberta, et al.
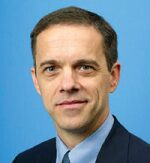
John Hudson, SPE, is a senior production engineer with Shell. He has more than 25 years of experience in multiphase-flow research, flow-assurance design of deepwater production systems, and development of model-based real-time operations decision systems. Since joining Shell, Hudson has held technical and managerial positions in Europe and North America, including leading a team that developed a model-based cloud computing solution that was deployed globally to gas plants with a total production capacity in excess of 10 Bcf/D. He currently provides production-engineering support for the development of a next-generation simulator. Hudson holds a PhD degree in chemical engineering from the University of Illinois. He serves on the JPT Editorial Committee.