While machine learning (ML) is used extensively to predict black-oil properties, it is used less often for compositional reservoir properties, including those related to gas injection. Can typically extensive conventional laboratory data be used to help predict the necessary gas-injection parameters? This question is addressed in the complete paper. The authors present an ML-based solution that predicts pertinent gas-injection studies from known fluid properties such as fluid composition and black-oil properties—that is, learning from samples with gas-injection laboratory studies and predicting gas-injection fluid parameters for the remaining, much larger data set.
Methodology
The objective of the ML component is to predict the results of the swelling test using compositional and black-oil properties. Fig.
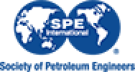