The growing adoption of machine learning (ML) is creating a vast opportunity to achieve major improvements in operating and financial performance throughout industry and very much so in upstream oil and gas. Yet if change can be unsettling, the perceived change stemming from the spread of ML and its relative, artificial intelligence (AI), can feed alarming predictions about massive job losses and questions of whether there will eventually even be jobs for most of the population.
However, past major transformations such as the industrial revolution and the rise of automotive transportation generated as many jobs as they replaced and ultimately more.
While AI and ML may not exactly fit that template, the replacement of humans is generally a low priority among organizations implementing these technologies, said Ramanan Krishnamoorti, chief energy officer at the University of Houston and moderator of the symposium, “Energy, Artificial Intelligence, & Robotics: The Future of People in Energy,” held there in March.
Certain tasks will undoubtedly be replaced, including some that are highly repetitive and activities where robotics can eliminate unsafe work. But jobs will be improved and new ones created, especially if efficiency gains can translate into stronger economic growth.
Enhancing Human Judgment
“If you read a lot about machine learning and AI, you see stuff about ‘robot apocalypse, they’re going to take all our jobs’ and everything,” said David Fulford, staff reservoir engineer in exploration evaluation at Apache. “However, what we’ve found is that this technology is not better because it replaces humans but because it enhances human judgment.”
“I know it’s a big industry ‘it’ word, and there’s a lot of traction behind this,” said Vincent Dumlao, senior adviser for oil and gas at Intelie. “But fundamentally, like anything else, you have to have a good foundation of data—good data in, good data out. There is no machine learning button. You have to have a good idea of what your data are and what you want to get from your data, and then pick the proper machine learning technique to draw information from that data to make those correlations.”
And to have that familiarity with specific data sets, perceive where the data might lead, and select the appropriate technique to learn from the data will require a growing number of very well-informed humans.
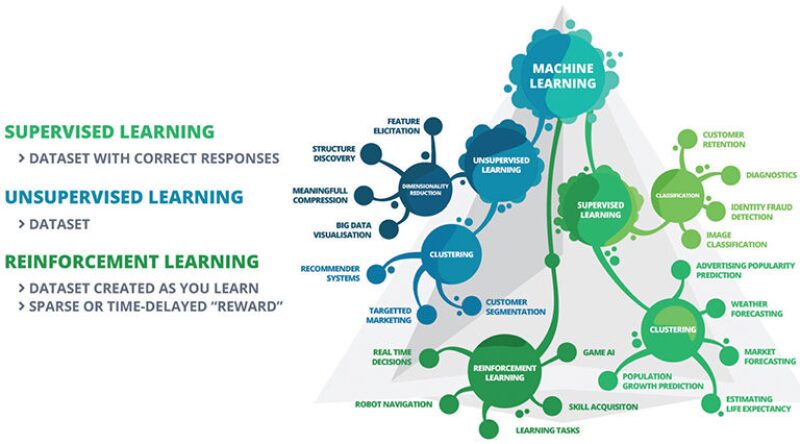
400,000 Open Jobs
Rashed Haq, global head for AI, robotics, and data at Sapient Consulting, said at the symposium that in the US alone “there are 400,000 open job positions for data science and machine learning that are not getting filled because there is not enough supply.”
At Intelie, many of the company’s client projects in upstream oil and gas involve ML applications that look at well sensor data, recognize patterns, and detect how they interact with specific operations or events in the wellbore.
“This goes into ROP [rate of penetration] prediction and optimization models, as well as geosteering models,” he said. “These are more simplified projects from a machine learning standpoint, and then we get into higher things. We had a project where we did mud-weight prediction using pure data. This was working in the Brazilian presalt.”
Drilling Below Salt
The case, in which Petrobras was the operator, was discussed in paper OTC 24275-MS. In past projects, predictions from the operator’s physics-based modeling of pore pressure, which were used to predict the needed mud weight, had proved unreliable when drilling transitioned out of the salt layer.
“Petrobras had a lot of data from all of these salt exits from 53 wells they had drilled,” Dumlao said. “So applying machine learning to this data, using the Random Forest method, the project team created a pore-pressure model for those salt exits. Petrobras now has a good model, which supplements its physics-based modeling, to predict mud weight for everything above and below the salt, and the company is using the new model for drilling these subsalt wells.”
Another area of ML that Intelie is working on is reinforcement learning for autonomous, adaptive control of equipment. “There are applications in every facet of the oil industry,” Dumlao said. “On the drilling side, geosteering is one and early kick detection is another. Geosteering is very analogous to self-driving cars. You’re taking a lot of data and are always self-referencing where you are to where you were and where you should be.”
A Digital Decision Assistant
The company is also helping an operator to develop a digital decision assistant. “If the real-time data feeds from well sensors are saying that you’ve taken a massive fluid loss or that this is likely to happen, we’re building a machine-learning platform that will notify all of the relevant people and draw all of the contextual data,” Dumlao said. “For example, if this happened in a previous well, what were the mitigation efforts and who were the people responding to it?”
At Apache, Fulford’s group has developed an ML method to generate more accurate rate-time production forecasts for unconventional oil and gas wells than humans typically can.
These ongoing forecasts are meant to guide company operations and decision making. The problem that Apache was encountering is prevalent throughout the industry—forecasts that generally have overestimated actual production numbers. In addition to the suboptimal guidance such forecasts provide for management decisions, the long-term inaccuracy could result in write-offs of booked reserves.
Bias in Forecasting
Fulford’s group identified the problem as bias on the part of production data evaluators—not bias in the colloquial sense that implies systematic error, but bias viewed simply as an expression of belief by the evaluator.
“This problem, consistently overestimating, is really a human issue; humans tend to be optimistic,” Fulford said in a presentation to the SPE Gulf Coast Section data analytics study group earlier this year. (Dumlao was also a presenter at the event.)
Estimation turns out to be a skill with little correlation to subject matter expertise. “Just because you know the most in the world about a subject doesn’t make you any better at creating estimates for forecasts about unobserved behavior,” Fulford said.
Excessive Human Confidence
He cited research published in JPT by E.C. Capen in 1976 that described how individuals were given a true/false test and asked to express confidence levels in their answers. The answers reflecting a 90% confidence level were found to have an average accuracy of only 65%.
To improve the company’s ability to forecast future production from unconventional wells, forecasts needed to be calibrated by quantifying the bias in those forecasts—with bias defined as “the difference between an estimator’s expected value and the true value of the parameter being estimated,” in Fulford’s words.
If the bias represents the estimator’s belief, that belief could encompass influences from other reputable estimators and their forecasting methods. But “none of this is based on any real rigor,” Fulford said.
Past May Not Predict Future
Forecasters have traditionally applied their modeling tools to observed—i.e., past—production data in ways that represent a “best fit” of the models to those data. However, “we’re not forecasting what we’ve seen; we’re forecasting what we haven’t seen,” Fulford said. “So the past is often not the best predictor of the future. The best predictor of the future comes from the mechanistic models we have to describe what happens next from the physics that we understand.”
Incorporating those mechanistic models, Apache developed a relatively simple, easily applied ML modeling tool for producing forecasts. It uses a Markov Chain Monte Carlo method to quantify a forecasting bias that is based on MLs from all past company forecasts.
“We are able to evaluate our own beliefs, calibrate those beliefs, and get better beliefs,” Fulford said.
More Consistency, Accuracy
Processing ongoing production data, the tool generates forecasts that represent a “most likely fit” of the modeling to unobserved data. Since adoption in 2014, the results have been much more consistent and accurate than the previous human forecasts. Use of the tool is now the standard practice, with other forecasting options allowed only after the tool has been run.
The tool is used constantly to make new forecasts. After a small amount of staff learning to set up the model, “forecasting 20 or 100 or 1,000 wells is no more work than forecasting 10,” Fulford said. “So this overload of work of forecasting these wells every quarter has become a nonissue.”
The model is updated continuously as new data are entered. “We are able to do that because it’s extremely fast,” he said. “It’s on the order of 10 to 100 milliseconds to generate 20,000 forecasts for a well and summarize those. And so to run 4,000 wells in the Permian Basin is on the order of 1 or 2 hours per month.”
Better Preventive Maintenance
Another major opportunity for AI and ML applications is predictive equipment failure for improving preventive maintenance. Addressing the University of Houston symposium, David Reid, chief marketing officer at NOV, spoke of the use of big data to understand the condition of an individual piece of equipment precisely where it is being used and calculate when it will fail.
“For a long time, we had a challenge where people thought we had too much data,” he said. “But really then came big data, just in time, where we could start having the ability to process all that and know when we would have a failure, where we would have a failure, and start getting the systems to learn what failure looks like and when it’s coming. Suddenly, the whole system gets better.”
A machine can know when it is soon to fail and “start ordering parts—start looking around the world to see if there are parts,” Reid said. While not yet possible, 3D printing technology could eventually enable the machine to build the part.
Improved Drilling Performance
Drilling performance is another area where AI, ML, and robotics are proving transformative, particularly with the growth of directional wells, which began offshore and has mushroomed with the onshore shale revolution.
The proliferation of downhole sensors, now enhanced by wired pipe, yields an unprecedented level of real-time data to an industry with an equally unprecedented ability to process these data, including the application of tools that can learn from it and respond to it accordingly.
The use of these tools removes many of the most repetitive tasks of the driller and reduce stress on the job. “You can actually elevate the role of the driller; so the driller remains connected,” Reid said.
Where wired pipe is used, the drilling system “is approaching the status of a closed loop,” he said. “What it does is it causes us to drill smarter wells, better wells. And they’re not just straight, but they’re costing less, they’re drilling faster.”
Systems now in use have pushed consistency and repeatability to new levels. “When you get repetition in a business system and you get consistency,” Reid said, “the back side of that is your whole supply chain can know when to turn up, so the waste factors go down.”
For Further Reading
OTC 24341-MS Development of Software To Predict Mud Weight for Pre-Salt Drilling Zones Using Machine Learning by G.T. Teixeiria, Petrobras, et al.
SPE 174784-MS Machine Learning as a Reliable Technology for Evaluating Time-Rate Performance of Unconventional Wells by David S. Fulford, Apache, et al.
SPE 5579-PA The Difficulty of Assessing Uncertainty by E.C. Capen, Atlantic Richfield Co.