Kick and lost-circulation events are major contributors to nonproductive time. In the absence of good flow-in and flow-out sensors, pit-volume trends offer the best possibility for influx and loss detection, but errors occur because external mud addition and removal to the pits is not monitored or sensed. In the complete paper, the authors introduce a method that uses a Bayesian network to aggregate trends detected in time-series data with events identified by natural language processing of driller memos, thereby improving significantly the accuracy and robustness of kick and lost-circulation detection.
Methodology
The objective of this project was to develop a model to detect and quantify kick and lost-circulation events using readily available real-time surface signals. This model used drilling memos to supplement real-time surface signals.
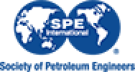