The Society of Petroleum Engineers is planning a series on petroleum data analytics (PDA) at the SPE Houston Training Center. The series will kick off with Week One: Subsurface Analytics on 24–28 February and will be led by University of West Virginia Professor of Petroleum and Natural Gas Engineering Shahab Mohaghegh.
For 5 days, attendees will be presented with a myriad of topics centered on the analytics necessary to demystify the subsurface. The topics are
- Day 1—Artificial Intelligence and Machine Learning: Theoretical Background
- Day 2—Python for Petroleum Data Analytics
- Day 3—Shale Analytics: Completion and Production Optimization Using Artificial Intelligence and Machine Learning
- Day 4—Reservoir Analytics: Data-Driven Reservoir Simulation and Modeling
- Day 5—Reservoir Analytics: Enhancing Numerical Reservoir Simulation using Artificial Intelligence and Machine Learning
Course Description
Petroleum data analytics (PDA) is the application of artificial intelligence (AI) and machine learning (ML) in the oil and gas industry. The future of our industry will be highly influenced by PDA. Engineering-domain experts who become highly skilled AI and ML practitioners are the ones who will control the future of engineering disciplines, including petroleum engineering. Becoming an engineering-related AI and ML expert practitioner requires fundamental understanding and extensive experience using AI and ML to solve engineering-related problems.
The objective of this weeklong PDA series is to provide the required and realistic foundations to the new generation of petroleum professionals that has recognized the potential of AI and ML in the industry. Clearly, short courses will not cover or provide all that is necessary for petroleum professionals to become true PDA experts. However, a week such as this will play a crucial role for the enthusiast of this technology in identifying the scientific realities associated with the foundation of AI and ML and its true application in PDA.
Petroleum Data Analytics
PDA experts are expected to be able to use AI and ML to solve petroleum-engineering-related problems in ways that are different from how such problems have been addressed in the past 70 years in our industry. The difference has to do with avoiding assumptions and simplifications that have played a significant role in addressing most of the traditional petroleum-engineering solutions. Traditional engineering approaches to problem solving in our industry incorporate simplified mathematical equations that are overwhelmed by assumptions in order to model highly complex physical phenomenon that is not directly observable. Continuing to use such traditional approaches and combining them with AI and ML algorithms (hybrid models) minimizes the contribution of this highly capable technology to engineering problem solving.
While some approaches of such combinations may help the conventional methods to work better; faster; and, in some cases, even easier, this is not the way AI and ML can contribute to the industry. The application of such hybrid approaches to completion and production optimization of shale wells is a good example. Using the traditional methods as the foundation of achieving such objectives removes the science and the reality from modeling and analyses of hydrocarbon production from shale plays. Then, using AI and ML to contribute to such hybrid approaches only makes the separation of science and reality from modeling and analysis easier and faster to accomplish. This is not only a comment and an assumption; it is based on actual work that we did and proved to be achievable. Another example of such combination is the enhancement of traditional decline curve analysis through the inclusion of physics. This would be a better example, but it is still a combinatorial (hybrid) approach and should not be referred to as AI and ML.
PDA incorporates AI and ML to solve highly complex petroleum-engineering-related problems by avoiding traditional methods, not by combining them. Realistic, scientific, and purely data-driven analysis and modeling to represent the complex physics of the phenomena is the way AI and ML must be used in the context of PDA. This requires that PDA experts have three characteristics:
- Domain expertise
- Expertise in practicing AI and ML
- Realistic understanding of AI and ML
Domain Expertise
PDA experts must have domain expertise in the specific areas of petroleum engineering that they are addressed using AI and ML. Examples of such domain expertise are being drilling expert, a completion expert, a reservoir-engineering expert, a reservoir-modeling expert, a reservoir-management expert, a surveillance expert, a production expert, or a surface facility expert. Lack of domain expertise takes us back to the initial application of this technology in our industry by some operating and service companies. They brought in experts of AI and ML from Silicon Valley and spent large amounts of resources. A waste of time and resources was the main outcome.
Expertise in Practicing AI and ML
PDA experts must develop expertise in solving and addressing petroleum-engineering-related problems through purely data-driven AI and ML approaches. Understanding the mathematics behind the ML algorithms or having the capabilities to code such algorithms does not turn petroleum-engineering professionals into PDA experts. While understanding the mathematics behind the ML algorithms is an absolute requirement, and being able to use Python to code such algorithms is a useful capability, at least within the next few years, they are not enough to turn petroleum engineers into expert practitioners of the application of AI and ML in engineering-related problems.
This must be made very easy to digest. All one needs to do is to ask oneself the following question: Is a fresh petroleum engineering graduate with a bachelor’s degree a reservoir-engineering expert? Such individuals have taken about 6 to 18 credit hours of courses that are somehow related to reservoir engineering in the last 2 years of their education. Nevertheless, once they start to work as a reservoir engineer for an operating or service company, they will go through a series of training to be exposed to the realities associated with the nature of and fluid flow through porous media. The reservoir-engineering experts usually are those with many years of field experience, not just a few courses in academia. The same is true for PDA. PDA experts require actual practices of application of AI and ML in the areas in which they have domain expertise. This takes a lot of time and may require many vicissitudes. It will never happen in a couple of months or a couple of years. We need to be realistic about the application of AI and ML for engineering-related problem solving.
A lack of expertise in the application of AI and ML by domain experts currently is being experienced by some operating and service companies. When some petroleum professionals who have been exposed to AI and ML technology for a couple of years have applied this technology to a few problems, some of these applications end up being unsuccessful. They then move toward “hybrid models,” which means back to traditional engineering approaches in order to get the AI and ML to provide reasonable results. This is just as bad as the lack of domain expertise when AI and ML experts from Silicon Valley were used solve petroleum-engineering-related problems.
Realistic Understanding of AI and ML
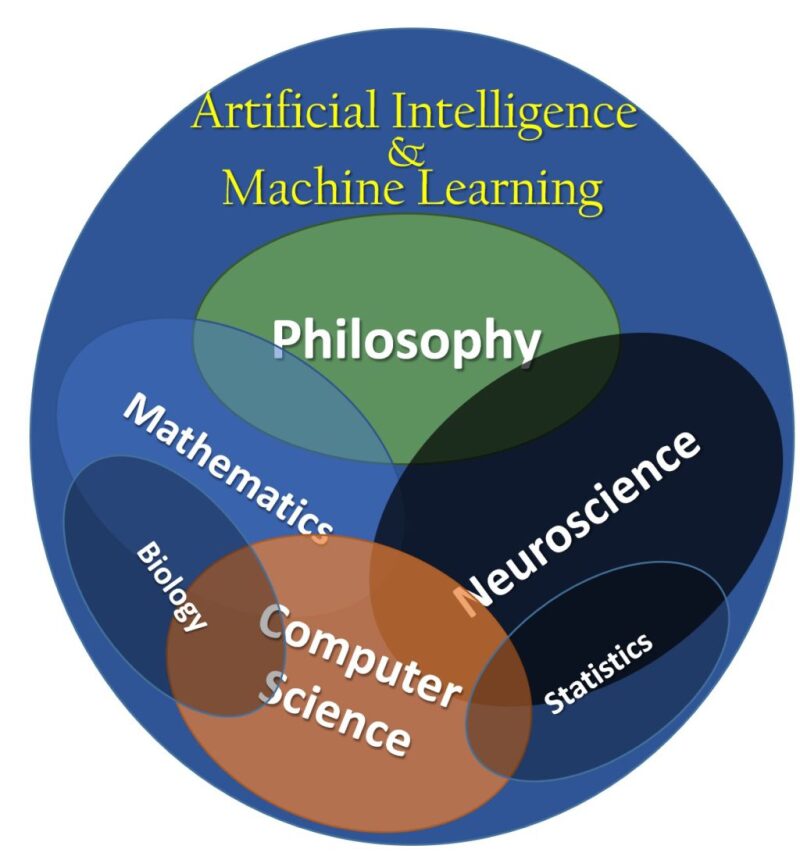
sciences to AI and ML.
Having some fundamental knowledge of AI and ML is incredibly attractive and can end up being highly addictive (once one becomes involved in it in a realistic manner, moving away from it can be very difficult). AI and ML is a complete paradigm shift in science and technology. As one becomes interested in learning more about this technology, one may become very interested in biology, neuroscience, evolution, philosophy, statistics, mathematics, and computer science (Fig. 1). Developing such a deep understanding of this paradigm shift is not necessary to become an expert engineering practitioner of this technology, but a certain amount of such understanding is required so that one does not mix this technology with traditional approaches to engineering problem solving. The more of this understanding that one develops, the more one becomes interested in researching this topic and developing new approaches to engineering problem solving.
Among the three major characteristics listed, domain expertise is the most important, followed by expertise in a large number of realistic practices of AI and ML algorithms. Major knowledge and expertise in the foundation of the AI and ML contributes significantly to becoming a research scientist in PDA. However, it is not necessarily a requirement for being a PDA expert. Nonetheless, the fact remains that knowledge of the foundation of AI and ML will be a characteristic of PDA experts, once they increase their interest in the topic.
Mohaghegh has authored three books (Shale Analytics, Data Driven Reservoir Modeling, and Application of Data-Driven Analytics for the Geological Storage of CO2) and more than 170 technical papers and has carried out more than 60 projects for independents, national oil companies, and international oil companies. He is an SPE Distinguished Lecturer (2007–08) and has been featured four times as the Distinguished Author in SPE’s Journal of Petroleum Technology (2000–04). Mohaghegh is the founder of Petroleum Data-Driven Analytics, SPE’s Technical Section dedicated to artificial intelligence and machine learning. He has been honored by the US Secretary of Energy for his AI-related technical contribution in the aftermath of the Deepwater Horizon accident in the Gulf of Mexico and was a member of US Secretary of Energy’s Technical Advisory Committee on Unconventional Resources in two administrations (2008–14). Mohaghegh represented the United States in the International Standard Organization in Carbon Capture and Storage (2014–16).