In the complete paper, the authors present a workflow that combines probabilistic modeling and deep-learning models trained on an ensemble of physics models to improve scalability and reliability for shale and tight reservoir forecasting. Their approach is applied to synthetic cases and many wells in the Permian Basin. Through hindsight studies, these models have been demonstrated to generate realistic and diverse production curves, capture the physics of unconventional flow, quantify well-production-outlook uncertainty, and help interpretation of subsurface uncertainty.
Introduction
In the realm of unconventional asset development, scalable forecasting is a key component in forecast reliability. In recent years, data-driven machine-learning models and workflows have emerged as potent tools for predicting well performance, particularly in scenarios where wells share similar reservoir properties, completion designs, and operational conditions.
A novel approach known as physics-informed machine learning (PIML) has gained prominence.
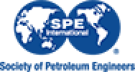