This paper proposes a metric for quantifying drilling efficiency and drilling optimization that is computed by use of a Bayesian network. The network combines the identification of drilling dysfunctions (i.e., vibrational modes), autodriller dysfunctions, and mechanical-specific-energy (MSE) tracking into a single, normalized quantity that the driller can use to help decide which control parameters to adjust. The driller may be provided with operational cones on a weight-on-bit (WOB)/rotary speed plot to assist in this task.
Methodology
The method proposed in this paper combines real-time surface measurements available on a drilling rig, derived quantities such as MSE and bit aggressiveness, and formation data (e.g., rock strength) into a probabilistic framework capable of handling the inherent uncertainty in the data and the process. The measured and derived parameters are encoded into a set of probabilistic features indicative of either the location of a particular physical attribute or a trend/movement of the attribute.
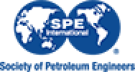