The authors used a high-quality digital-log data set to characterize reservoir quality accurately in the Niobrara and Codell Formations in the Denver-Julesberg (DJ) Basin. A petrophysical work flow was developed, and detailed mapping of the reservoir attributes was completed. The log-derived parameters, along with an aeromagnetic and vitrinite-reflectance data set, provided excellent insight into which geologic parameters could be tied best to well-production response.
Introduction
In 2013, the authors began to evaluate production response in an area where nearly 50 Niobrara wells were completed by a single operator with a similar completion design for all wells. There was a wide variation in production results after 180 days of production, ranging from 4 to 16 BOE/lateral ft.
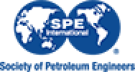