The role of process operations upstream, midstream, and downstream is critical to fundamental business resilience and production optimization in a dynamic environment. In the complete paper, the authors propose a fast, hybrid, self-learning dynamic process-modeling method derived from routine plant measurements that can be used for short-term forecasts, scenario modeling, and process optimization and control.
Introduction
The methodology, reduced-order modeling (ROM), can use large quantities of data from plant sensors to create a dynamic process model that can be used to optimize process performance. The authors propose a model structure and a hybrid method that is physically intuitive and interpretable and can learn incrementally in real time and thus can augment human intelligence with fast machine-derived insights.
Problem Description
In the offshore facility system considered in this work, the oilwell flowlines are connected to the platform through risers that terminate at the facility inlet. Each topside flowline includes a subsea production flowline connected to a pressure-controlled choke.
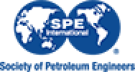