This paper presents a new geostatistics modeling methodology that connects geostatistics and machine-learning methodologies, uses nonlinear topological mapping to reduce the original high-dimensional data space, and uses unsupervised-learning algorithms to bypass problems with supervised-learning algorithms. The algorithm presented is a neural topology-preserving pattern-based geostatistical simulation algorithm that integrates the self-organizing map (SOM) concept and its updated version—growing self-organizing map (GSOM)—with an unsupervised competitive learning structure.
Introduction
In oil and gas reservoir modeling, any model construction faces challenges of limited data to some extent. The heuristic behind all geostatistical techniques is the implicit existence of statistical relationships among available data. “Data” here is a broad term; it could be discrete points, such as porosity or permeability at certain locations, but it also could be training images (TIs), which are used in this work.
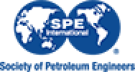