In the complete paper, a novel hybrid approach is presented in which a physics-based nonlocal modeling framework is coupled with data-driven clustering techniques to provide a fast and accurate multiscale modeling of compartmentalized reservoirs. The research adds to the literature by presenting a comprehensive work on spatiotemporal clustering for reservoir-studies applications that considers the clustering complexities, the intrinsic sparse and noisy nature of the data, and the interpretability of the outcome.
Introduction
History matching is the most time-consuming phase in any reservoir-simulation study. As a means of accelerating reservoir simulations, a 2018 study proposed an approach in which a reservoir is treated as a combination of multiple interconnected compartments that, under a range of uncertainty, can capture the reservoir’s response during a recovery process. In this work, the authors extend that approach to represent a reservoir in a multiscale form consisting of multiple interconnected segments.
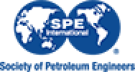