Methods such as interference tests and tracers have been developed to obtain knowledge of reservoir heterogeneity and connectivity from dynamic data. However, detecting well connectivity using interference tests requires long periods with stable reservoir pressure and constant flow-rate conditions. Conversely, the long duration and high frequency of well-production data have high value for detecting connectivity if noise, abrupt changes in flow rate, and missing data are considered. In this work, a methodology to detect interference from long-term pressure and flow-rate data was developed using multiresolution analysis in combination with machine-learning algorithms.
Introduction
Multiple methodologies have been proposed to detect well connectivity using production data.
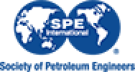