The success or failure of neural networks in discerning rock properties through a quantitative approach from seismic data and associated seismic attributes is dependent on the amount of available data. Past researchers developed a hybrid theory-guided-data-science (TGDS) technique that augmented the amount of data used for training. This paper shows the application of this method of TGDS-based synthetic seismic catalog generation followed by the application of a deep feed-forward neural network (DFNN) to a real seismic data set for a commercially oil-producing field in the North Sea.
Introduction
Machine-learning algorithms have been used for several applications in the field of reservoir characterization. Using neural network techniques for supervised learning applications to predict log properties from seismic and seismic attributes is an established technique.
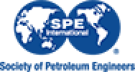