It is critically important for decision making and an extremely challenging task to quantify the uncertainty of model parameters and production forecasts properly after conditioning to production data. This paper presents a novel approach to generate approximate conditional realizations using the distributed Gauss-Newton (DGN) method together with a multiple local Gaussian approximation technique. Results are compared with those obtained from other approaches, such as randomized maximum likelihood (RML), ensemble Kalman filter (EnKF), and Markov-chain Monte Carlo (MCMC).
Introduction
It is well-known that oil- and gasfield development is a high-risk venture. Uncertainties originating from geological models (e.g., structure, stratigraphy, channels, and geobodies) are coupled with uncertainties of reservoir models (e.g., distribution of permeability and porosity in the reservoir) and uncertainties of economic parameters (e.g., oil and gas prices and costs associated with drilling and other operations). It is critically important to properly quantify the uncertainty of such parameters and their effect on production forecasts and economic evaluations.
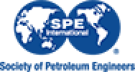