Can a camera do a better job than humans at creating a minute-by-minute record of drilling activity? Or evaluate damaged drill bits better?
Based on two papers at the SPE/IADC International Drilling Conference and Exhibition, the answer might soon be yes.
Schlumberger researchers showed that video from a camera on the drill floor and on the drilling cuttings shaker kept an accurate record of what the rig was doing at the time, also known as the rig state (SPE 204086).
A paper from The University of Texas-Austin (UT-Austin) showed a camera phone could create images for use in their bit-evaluation program to assess the damage and offer a reasonable explanation for what caused it (SPE 204124).
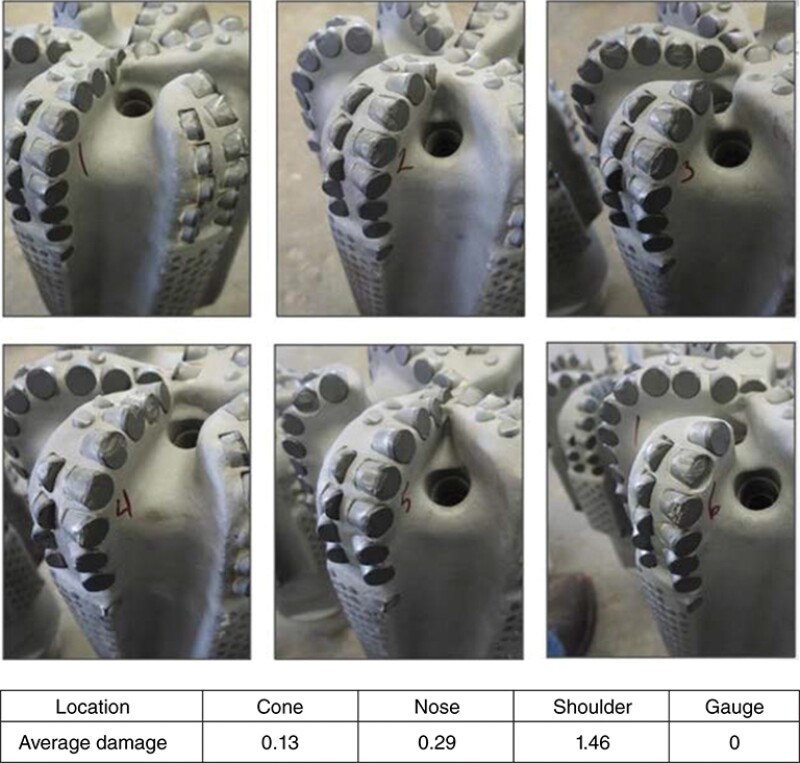
A year ago Teradata announced its BitBox, which houses cameras and lights to create 3D bit images. It is now on the market; other companies are working on competing products.
Cameras have gotten small and cheap at a time when innovations like self-driving cars have driven tech companies to speed development of computer-vision tools.
Schlumberger’s goal is to convert video from rig cameras into a constant record of the rig state. It is being done with data gathered by sensors while drilling, or by people if the sensors stop working or generate bad data.
The system leaves gaps when sensor issues are missed or workers are otherwise occupied, which is a maddening problem for those who need to know what is going on at any moment to interpret drilling data.
“We need to understand what the rig was doing when we look at and try to solve problems” using digital analysis, said Crispin Chatar, a drilling subject matter expert for Schlumberger, who presented the paper at the virtual conference.
The bit-evaluation program used phone camera images as a lower-cost substitute for 3D imaging, like the system sold by Teradata.
Mobile phone images come with the risk of bad lighting and varying angles, which the authors said affected the analysis. But those devices are small, and there are plenty of backups available.
“Everybody has a mobile phone at a rig site,” said Pradeep Ashok, a research scientist in the petroleum engineering department at UT-Austin. He believes cameras on mobile devices are the future because “if you could build something that requires no added equipment it will allow more rapid adoption.”
Mobile phone owners also worry about breaking their phone. They may not be so concerned about rig equipment, which is a concern to those selling more-complex imaging devices.
Both projects were early efforts to test what can be done with camera data, which is likely to expand over time.
Schlumberger’s goal of tracking the rig state more reliably and cheaper than when using sensors may not be enough to convince users to change their ways at a time when sensors are proliferating to support more digitally controlled activities. The Schlumberger data were gathered at a test well for an automated rig.
“An automated system command defines the [rig] state,” while drilling, said John de Wardt, a drilling consultant who has worked for years on an automated-drilling-systems roadmap to track the transition toward automation and project where it is going.
While he sees value in consistent rig state reporting, he expects Schlumberger is working toward other uses.
“I would postulate that the next step is using cameras for observing flat time,” he said. Now done by people on site, “visual reporting with AI [artificial intelligence] would be a real step forward in broadening and automating states reporting.”
A First Look
Chatar described the work presented as the “start of a journey.”
The project was run by staffers in Schlumberger’s Menlo Park office in California using tools more commonly associated with self-driving cars. One of the authors of the paper has since moved from Schlumberger to Uber AI, which is working on automated transport.
The program used to visually identify what the rig was doing was chosen because it could learn quickly, a critical skill when dealing with drilling rigs equipped with many different combinations of parts.
The Schlumberger test was done on a rig with 18 cameras, but only two were needed. One was focused on the floor of the automated rig, where no workers were present in the picture used in the report. The second one tracked cuttings moving through the shaker, providing physical evidence of activity in the hole.
The models were trained using images drawn from a 3-million frame dataset, labeled with information on the rig state at that moment, and the programs learned what visual cues were associated with a short list of common rig states.
That program was a “convolutional neural network,” which is software designed to mimic the pattern-recognition process used by the brain.
The paper reported that the accuracy rate for visual tracking ranged from a low of 71% for “ream down with flow” up to 98% for when the rig was in slips. The system had trouble distinguishing one rig state from another when the fluid flow was similar, such as when tripping in or out.
The rationale for developing the system—the way it is now done means the data are sometimes bad or missing—poses a quandary for those looking for a benchmark to measure the accuracy of video rig state tracking.
An experienced rig worker’s interpretation of the images is an option, but there are simply too many images to consider for that to be a practical one.
Faced with a similar problem, tech companies developing driving applications created industry benchmarks to define what is being measured (the paper noted that rig state definitions vary from company to company) and datasets to use for performance testing.
“What is needed is both a standard and a standard dataset to measure it by, so when a new benchmark comes along you can determine if it measures it better than the previous one,” said Soumya Gupta, now a machine-learning engineer at Uber AI.
The Meaning of the Measure
The idea that “a drill bit is still the best indicator of what happened downhole” sounds like a good one.
Ashok made that observation at the start of a presentation providing an early look at how it could be done.
Experienced drillers could do the same, but their judgment “is based on experience and that can be very subjective,” he said.
What they are working toward is a standard view of reality based on 2D photos of variable quality shot using phone cameras.
Despite those limits, the test concluded the bit-evaluation software was able to accurately map the location of the cutters, with a few exceptions, by systematically imaging, starting at the bottom and counterclockwise. The shape of each diamond-tipped cutter is compared to its original condition.
The first use for bit imaging will be grading cutter wear after drilling. These numbers matter because they are a factor in the cost of leasing a bit. In the UT-Austin test, the condition was graded generally close to those of human experts, Ashok said.
The next step is tougher: using the cutter-wear data to diagnose the cause of the damage.
To simplify this test, the paper limited the diagnosis to three possibilities: whirl, stick/slip, and bit bounce. The data on the location of the wear were entered into a decision tree. Boxes on the triangular grid were associated with various sorts of wear, which led to a conclusion indicating the likely cause.
In one example, cutter wear was focused on the shoulder with lesser amounts on the cone and nose, leading to a diagnosis of bit whirl. Based on downhole data, the system seemed to work. But a 24-bit sample falls far short of enough to pass judgment.
The paper said the accuracy could be improved with better imaging data, comparing the wear to pre-drill data rather than manufacturers’ specifications, and creating a phone app that would limit variations in the picture quality.
To measure the quality of this digital imaging analysis, the researchers used the judgment of human experts and sensor data, which led to a question about what makes this new system better.
Ashok noted that the computerized system would be based on a select group of experts in the bit-grading system developed by the International Association of Drilling Contractors. “They could do it better than thousands of people doing that,” Ashok said.
For Further Reading
SPE/IADC 204086 Determining Rig State from Computer Vision Analytics by C. Chatar and S. Suresha, Schlumberger; L. Shao, Stanford University, et al.
SPE/IADC 204124 Drill Bit Failure Forensics using 2D Bit Images Captured at the Rig Site by P. Ashok, J. Chu, Y. Witt-Doerring, et al., The University of Texas at Austin.