The time needed to eliminate complications and accidents accounts for 20–25% of total well construction time, according to a 2020 SPE paper (SPE 200740). The same paper notes that digital twins have proven to be a key enabler in improving sustainability during well construction, shrinking the carbon footprint by reducing overall drilling time and encouraging and bringing confidence to contactless advisory and collaboration. The paper also points out the potential application of digital twins to activities such as geothermal drilling.
Advanced data analytics and machine learning (ML) potentially can reduce engineering hours up to 70% during field development, according to Boston Consulting Group. Increased field automation, remote operations, sensor costs, digital twins, machine learning, and improved computational speed are responsible. It is no surprise, then, that digital twins are taking on a greater sense of urgency for operators, service companies, and drilling contractors working to improve asset and enterprise safety, productivity, and performance management. For 2021, digital twins appear among the oil and gas industry’s top 10 digital spending priorities.
DNV GL said in its Technology Outlook 2030 that this could be the decade when cloud computing and advanced simulation see virtual system testing, virtual/augmented reality, and machine learning progressively merge into full digital twins that combine data analytics, real-time, and near-real-time data for installations, subsurface geology, and reservoirs to bring about significant advancements in upstream asset performance, safety, and profitability. The biggest challenges to these advancements, according to the firm, will be establishing confidence in the data and computational models that a digital twin uses and user organizations’ readiness to work with and evolve alongside the digital twin. JPT looked at publications from inside and outside the upstream industry and at several recent SPE papers to get a snapshot of where the industry stands regarding uptake of digital twins in well construction and how the technology is affecting operations and outcomes.
Why Digital Twins
Gartner Information defines a digital twin as a digital representation of a real-world entity or system. “The implementation of a digital twin,” Gartner writes, “is an encapsulated software object or model that mirrors a unique physical object, process, organization, person or other abstraction.” Data from multiple digital twins can be aggregated for a composite view across several real-world entities and their related processes.
In upstream oil and gas, digital twins focus on the well—and, ultimately, the field—and its lifecycle. Unlike a digital simulation, which produces scenarios based on what could happen in the physical world but whose scenarios may not be actionable, a digital twin represents actual events from the physical world, making it possible to visualize and understand real-life scenarios to make better decisions. Digital well construction twins can pertain to single assets or processes and to the reservoir/subsurface or the surface. Ultimately, when process and asset sub-twins are connected, the result is an integrated digital twin of the entire asset or well. Massive sensor technology and the ability to store and handle huge amounts of data from the asset will enable the full digital twin to age throughout the lifecycle of the asset, along with the asset itself (Fig. 1).
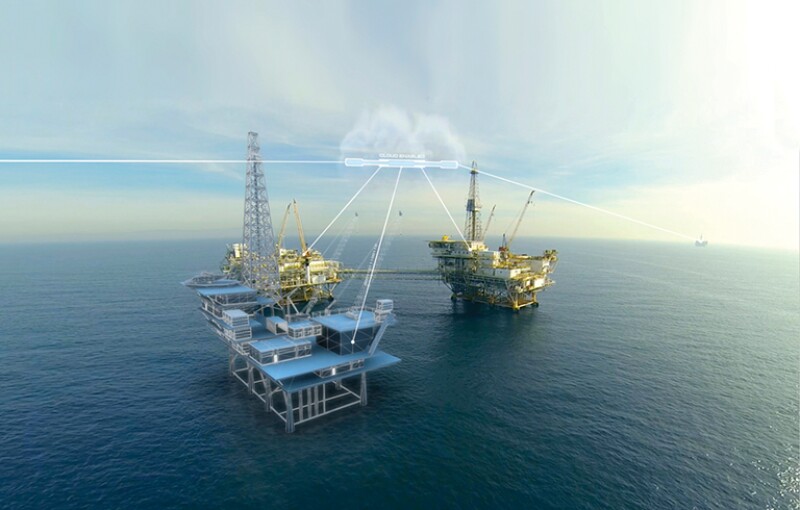
As the industry moves from automation of individual tasks toward automation of the full well construction process—from plan to completed borehole—digital twin technology will play an important role in the process by providing automatic quality control and calibration of drilling data; automated, forward-looking diagnostics and decision support; and, eventually, real-time automatic or augmented-intelligence optimization of the drilling process.
Major Investments and Results
Among the first industry end-to-end digital transformation implementations that included work flow automation and integrated data management was ADNOC Onshore’s North East Bab field in 2006. During the past 15 years, this implementation has expanded to include all of the company’s onshore assets.
BP has developed a highly sophisticated simulation and surveillance system called APEX, which it has implemented to create virtual models of all its production systems. Engineering time has been the biggest payback.
“A system optimization could take 24–30 hours. In APEX, it takes 20 minutes,” said Carlos Stewart, lead petroleum engineer at BP, in a press release from the company. “It can also be used to safely test ‘what if’ scenarios. By pairing the model with the actual data, irregularities can be detected hourly and the impact of procedures can be simulated to show engineers how they can tweak flow rates, pressures, and other parameters to safely optimize production,” Stewart said.
Eni is using digital twins to construct virtual versions of its wells in challenging operational conditions and simulate the effect of decisions in a safe environment. The company is also using digital twins to deliver innovative operational training, using 3D rooms, computer models, and virtual reality to simulate walkthroughs of real facilities, operational playback of real field activity, and advanced safety drills training.
Equinor’s digital twin of the entire Johan Sverdrup field is helping optimize operations at the field that will account for up to 25% of Norway’s total offshore production. The asset, which operators now can see on their tablets and phones, is the first for which Equinor is using a digital twin to give an up-to-date overview of real-time operating conditions.
Recent Concept and Pilot Examples
Researchers at the Rig Automation and Performance Improvement in Drilling (RAPID) consortium at the University of Texas at Austin have successfully tested a new concept that uses physics-based models with historical data from offset wells together with real-time data acquired while drilling new wells to predict time to total depth accurately and continuously and improve drilling performance. The concept was tested successfully using data from a four-well pad in the Bakken shale play. The researchers compare the concept to navigational aids for cars, which not only provide initial time estimates based on routing options but also update those estimates in real time to account for ever-changing outside circumstances.
Two exciting digital twin developments are being presented at the SPE/IADC Virtual International Drilling Conference and Exhibition, set for 8–12 March. One paper proposed for the conference, SPE 204050, describes the role of a digital twin in helping an operator, a drilling contractor, and a service provider create a collaborative business model for more-effective multiparty well planning and execution. The objective of the collaborative model is to deliver a new digital system that enables treating the well construction process as a single system made up of multiple systems owned by different stakeholders. This will enhance well construction planning and execution speed, completeness of data transfer, ease of access, and availability for building new data-based work flows and reporting.
One key issue the model addresses is the different technologies and capabilities supported by the different collaborating entities, which can lead to variability in assumptions and objectives in standardized plans. These, in turn, can result in execution risk and variations in operational safety, quality, delivery, and cost (SQDC) performance. For example, a major source of variability is the disconnected way people move data among one another in well planning documents and then to field operations.
An operator, service provider, and drilling contractor sought a new way to manage this process across organization and discipline boundaries to reduce the variability. They determined that implementing a digital twin representing each well in the asset could link the current-state process from one of limited discrete opportunities to a collective system that could reduce drilling costs by up to 25%. Each well’s digital twin includes the well program design, related engineering models, procedures or activity programs, risk and mitigation models, costs, and barrier assurance details.
The first phase of the model was delivered, and the first-generation digital system was safely installed. The process digitally linked the operator’s high-level standard work requirements to the drilling contractor’s more-detailed rig action plan and delivered the digital rig action plan to the driller. The system provides a clear visualization to the driller of the steps to deliver the well from spud to rig release. Rig personnel were onboarded and trained and had used the system to drill four wells when the paper was written. The work flows and processes built into the system resulted in the target SQDC performance and laid the groundwork for further process improvement (Fig. 2).
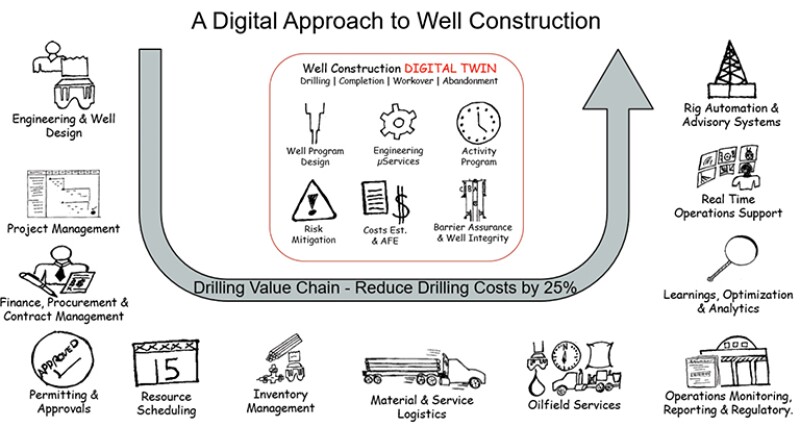
Another proposed 2021 Drilling Conference paper (SPE 204069) discusses an approach to drilling automation that illustrates how a digital twin of the well deployed in real time can update boundary conditions constantly while drilling to increase the ability of monitoring applications to both predict and react to potential drilling risks and to identify optimization opportunities while staying within a safe operating envelope that protects the wellbore. The digital twin also enables higher levels of automation because the delicate process part of drilling operations can be handed over to control applications that ensure safe execution.
The validity of the approach has been corroborated in multiple locations around the world using the same setup but different drilling objectives, proving the versatility of the solution. The next steps target the incorporation of new services from prejob planning into the real-time world to leverage the existing drilling automation ecosystem built during the development of borehole-pressure, tripping, and torque-and-drag monitoring services.
Building Trust
A consistent message from another 2020 paper, SPE 203251, on drilling automation in the digital age is, “Industry has yet to benefit on a large scale from these advancements and … significant value remains untapped.”
Digital twins represent what may be the biggest and best opportunity available for performance optimization, avoidance of nonproductive time, and hazard prevention in the energy industry. Scaling digital twins, however, and realizing its potential benefits requires addressing the elephant in the room—trust.
“Solving the digital trust challenge will be key to the dramatic evolution that we expect to see in digital twin technology in the years to come,” said Liv A. Hovem, chief executive officer of DNV Oil and Gas. “If more-sophisticated digital twins are to be widely accepted and developed at scale by the oil and gas industry, they need to be supported by accurate, valuable, and trusted technology. Physical oil and gas assets are built to perform to the highest standards and undergo rigorous assurance processes throughout their life. However, there has been no requirement for their digital counterparts to go through the same procedures. As the technology begins a path of significant scaling across the sector, it is time to prove that twins can be trusted and that the investments made in them give the right return.”
To that end, DNV, in collaboration with TechnipFMC, recently published DNVGL-RP-A204, the oil and gas industry’s first recommended practice on how to build and quality-assure digital twins. The recommended practice sets a benchmark for the sector’s varying approaches to building and operating the technology and is the first systematic plan to show how a digital twin is delivering as expected. The framework provides clarity on the definition of a digital twin, required data quality and algorithm performance, and requirements on the interaction between the digital twin and the operating system.
“When there is no common language between stakeholders as to quality assurance, it hinders scaling of technology,” said Kjell Eriksson, vice president of digital partnering for DNV Oil and Gas. “Our methodology is a process of providing evidence that a digital twin will provide valid information, predict system performance within well-defined limits and to a stated level of confidence over time.”
According to a press release, the recommended practice guides industry professionals through assessing whether a digital twin will deliver to stakeholders’ expectations from the inception of a project, establishing confidence in the data and computational models and evaluating an organization’s readiness to work with and evolve alongside a digital twin.
An article in the January 2021 issue of JPT (Artificial Intelligence in the E&P Industry: What Have We Learned So Far aned Where to Next?) presented another perspective. According to the article’s authors, three truths about artificial intelligence (AI) in oil and gas provide insight into where the industry stands with regard to digital twins and what will need to happen for the technology to scale fully. The first truth is that relatively little AI has occurred from the outset in a way that had the oil and gas sector in mind.
The second truth is that augmented intelligence, whereby AI supplements human judgment rather than supplants it, is not merely an alternative way of approaching artificial intelligence; rather, it is coming into focus that this is probably the most sensible way forward for this technology and that it is indispensable to have the benefit of a seasoned veteran to teach the algorithm what is actually important in the physical system. “We, therefore, will need to continue to safeguard and actively manage the deep technical expertise within the oil and gas industry’s people because that knowhow will prove to be a necessary foundation for taking AI to the next level in the sector,” wrote the authors. “Combining the petroleum engineering community’s deep understanding of the industry’s technical dimensions—for example, the complex physics behind subsurface phenomena—with the predictive power of AI will yield far better results than either physics or pure AI could achieve on their own.”
The third truth—a logical outcome of the first two—is that the industry and digital communities will need to collaborate to take AI to the next level in oil and gas.
Early examples of digital twins exist today, but this is only the beginning of development of true digital twins for complex assets and systems. There is still a great deal of work to be done to build the many interconnected models and to create the robust data streams from operations that will form the building blocks for widescale digital twins. The outlook, however, is optimistic. The consensus is that the industry will begin to see true digital twins for large, complex assets, including well construction, between 2025 and 2030.
For Further Reading
SPE 203251 Drilling in the Digital Age: Harnessing Intelligent Automation To Deliver Superior Well Construction Performance in Major Middle Eastern Gas Field by B. Goodkey, G. Hernandez, Andres Nunez, et al., Schlumberger.
SPE 200740 Digital Twins for Well Planning and Bit Dull Grade Prediction by M. Shirangi, E.Furlong, and K. Sims, Baker Hughes.
SPE 204050 A Novel Use of Digital Technologies for More Effective Multiparty Well Planning and Execution by M. Isbell, Hess Corporation, M. Manocha, Nabors Corporate Services, B. Mangold, Halliburton Landmark, et al.
SPE 204069 Integrated Real-Time Simulation in an Earth Model—Automating Drilling and Driving Efficiency by P. Arévalo, O. Hummes, and M. Forshaw, Baker Hughes.