Finding information across multiple databases, formats, and documents remains a manual job in the drilling industry. Large language models (LLMs) have proven effective in data-aggregation tasks, including answering questions. However, using LLMs for domain-specific factual responses poses a nontrivial challenge. The expert-labor cost for training domain-specific LLMs prohibits niche industries from developing custom question-answering bots.
×
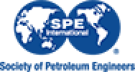
Continue Reading with SPE Membership
SPE Members: Please sign in at the top of the page for access to this member-exclusive content. If you are not a member and you find JPT content valuable, we encourage you to become a part of the SPE member community to gain full access.