Ever since the first commercial well was spudded, operators have looked for ways to drill wells faster without sacrificing safety or incurring huge costs. While saving time and money through efficient drilling is not a new concept, the more recent adoption of drilling optimization and automation services has certainly become one of the biggest drivers to achieving those goals.
As the current downturn has shown limited signs of recovery, it has continued to evolve in ways never imagined, and the effects are taking their toll in every facet of the oil and gas industry. While rigs and drilling equipment can be set aside to ride out the storm, what about the drilling teams who are working on the rigs and in remote operation centers? As these teams are being removed from the field, the expectation is that many of them won’t return for a myriad of reasons. So, what happens when that experience is lost?
The exodus of seasoned crews, otherwise known as the “great crew change,” has been discussed for several years, but recent conditions could expedite the process. Considering the recent shutdown of rigs and the loss of personnel, the question remains whether we will see a noticeable gap in knowledge and experience once crews return to the drilling rigs in full force.
The lack of individual skills can be offset over time with hands-on experience, but a drilling crew needs to operate at the highest level possible, preferably with few to no gaps in experience. To assist the drilling process, NOV’s M/D Totco division recently launched its KAIZEN intelligent drilling optimization application, which performs as an adaptive autodriller. The system features continuous learning capabilities, enabling it to provide proactive drilling dysfunction mitigation while maximizing rate of penetration (ROP) and optimizing mechanical specific energy. It also reduces human dependence in the drilling process, lowering the risk of slow or incorrect responses to drilling dysfunction. In turn, the system assesses wellbore conditions and drilling performance, then automatically applies appropriate parameters to mitigate those dysfunctions.
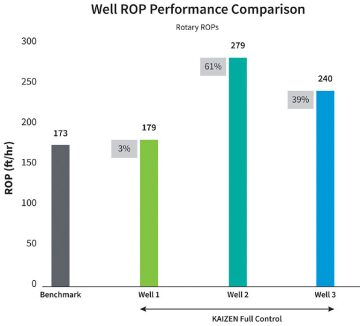
Intelligent Drilling Optimizer
When faced with distinct interbedded formations, drillers often encounter drilling dysfunction due to varying formations, and optimal setpoints are required to identify and proactively mitigate dysfunction.
While drillers are inundated with large amounts of data, the system takes the human dependence away and employs artificial intelligence (AI) to continuously optimize the drilling process. Utilizing an array of machine-learning algorithms and a digital twin that is updated each second, the AI system builds a store of knowledge that the drilling application leverages to make more accurate and timely decisions. This automated parameter application approach enables the system to remove distractions from the driller so their focus can be on critical items such as keeping the crew safe and the well under control, while the system instantly responds to changing conditions and provides optimal weight on bit (WOB) and revolutions per minute (rev/min) setpoints.
The AI and machine-learning feature stores thousands of hours of processed drilling data. This capability allows the system to recommend surface parameters that deliver the best expected performance as well as select the correct dataset to mitigate changes detected in drilling dynamic behaviors.
Additionally, the system’s digital twin uses an advanced, physics-based model to analyze several key dysfunctions in real time by way of distributed stress modeling, torque and drag modeling, and critical rev/min calculations. The result is a system that is capable of providing guidance to the parameter search engine, identifying which parameters to avoid. Drillstring buckling, downhole vibration (torsional, axial, and lateral), and mud motor stalls can all be diagnosed, and thus, mitigated in real time.
The system’s optimization functions can be run in either advisory or control mode. The advisory mode sends recommended rev/min and WOB setpoints for the driller to implement, while control mode sends those same setpoints directly to the autodriller.
Advisory mode is typically run if a rig’s control system is not fully compatible with the control mode. When a rig has a control system that is compatible with the KAIZEN command requests, control mode is activated, and the system consistently achieves a higher level of performance by continuously and automatically adjusting the setpoints.
The KAIZEN system has been successfully integrated into multiple control systems, including NOV’s NOVOS reflexive drilling system. The application is currently compatible in closed-loop mode with approximately 50% of the active North American rig fleet.
The drilling system’s installation comprises a minor update to the rig control layer to accept the system’s commands, along with the physical installation of a KAIZEN system box. The rig must be running NOV’s RigSense electronic drilling recorder, and the system box is simply plugged into both the rig’s control network and the RigSense system. The system is then accessible on the RigSense screen and operated through the control system interface.
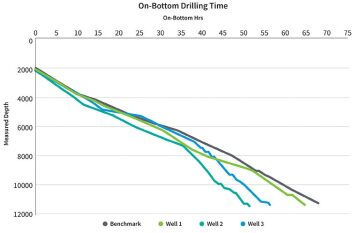
Case History: Marcellus Shale
The use of KAIZEN control mode on the NOVOS control system has recently shown immediate improvements in the field.
A drilling contractor operating in the Marcellus Shale sought a solution to complete each hole section in a single-bit run while improving the ROP and reducing drilling time with less damage to the bit. The target formation showed shale with interbedded limestone as well as concentrations of iron pyrite and siderite, making for a challenging well.
The intelligent drilling optimizer applied its continuous learning capabilities, successfully demonstrating the ability to optimize the drilling program by way of dysfunction mitigation and parameter optimization. It was noted that the system handled formation changes exceptionally well and exhibited creative solutions to solve downhole vibration, resulting in smooth drilling. This allowed the system to exploit this parameter map and drill at the limit of efficiency, and the system performed those functions consistently.
The use of the drilling system’s control mode on the NOVOS system showed immediate improvements. All three wells that used the system were compared against the customer-provided benchmark well; the system collectively saved the customer 38.6 drilling hours. The cumulative hourly savings translated to an average of $37,518 per well for a total savings of $112,554 over the three wells, based on a $70,000/day spread rate.
Conclusion
The need for greater efficiency through optimized drilling systems has pushed service companies into new and exciting areas of product development, with features like automation and AI leading the way. As the KAIZEN system’s performance has shown, the application of automated control systems delivers cumulative operational benefits that continue to increase independent of the crews’ experience levels. As the industry looks to optimize processes in all areas of operations, intelligent systems will become the accepted, pragmatic approach to achieving safer and more efficient performance at the wellsite.
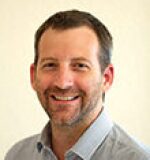
Christopher Jeffery is the product line director for NOV’s M/D Totco’s Digital Wellsite portfolio. He has worked at NOV for 12 years and has a background in drilling optimization. Jeffery currently works in the Houston headquarters.
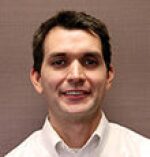
Andrew Creegan serves as the product line manager for NOV’s M/D Totco’s Digital Wellsite portfolio. He has worked at NOV for 7 years and has a background in performance drilling applications. Creegan currently works in the Houston headquarters.