Decline-curve analysis (DCA) is one of the more widely used forms of data analysis that evaluates well behavior and forecasts future well and field production and reserves. In the complete paper, the authors develop and deploy technologies that apply DCA methods to wells in an unbiased, systematic, intelligent, and automated fashion. This method contrasts with manual DCA, the common practice of the industry.
Introduction
DCA is used commonly to estimate reservoir and well productivity and ultimate recovery and evaluate reserves. Such analyses are usually performed manually through a curve-fitting process by reservoir and production engineers using their best judgement and experience.
×
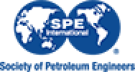
Continue Reading with SPE Membership
SPE Members: Please sign in at the top of the page for access to this member-exclusive content. If you are not a member and you find JPT content valuable, we encourage you to become a part of the SPE member community to gain full access.