The energy industry is facing mounting demands for stronger environmental and safety standards. The climate has made reliable, round-the-clock leak monitoring even more critical for high-consequence facilities such as well sites, pump stations, terminals, and production platforms, which generally are remotely located, are near sensitive environments, and have limited staffing.
Progress has been made in liquid leak prevention, detection, response, and remediation, with significant money poured into instrumentation, control room management, and digital twins. Still, there is considerable opportunity for improvement to increase asset condition monitoring capability. In leak detection, improvements have centered on increasing sensitivity, identifying locality, and reducing false alarms. The comprehensiveness and effectiveness of leak detection methods, however, are heavily influenced by how their individual strengths and limitations come into play collectively.
For example, traditional systems such as computation pipeline monitoring (CPM) and acoustic sensors automatically generate alarms but often necessitate a supplementary data source to confirm accuracy and severity because of a lack of visuals. In the presence of reading inaccuracies and the absence of ground truth, personnel are dispatched to the location for incident verification and condition assessment. Confirmatory visits cost time and energy and expose responders to site and road hazards as the leak progresses in severity.
Intermittent monitoring through visual inspections via boots on the ground or vehicle or aerial cameras add “eyes” to the sensory mix. The labor and operating costs associated with increased frequency or continuous implementation are prohibitive, however, and the extensive blind spots created by their periodic nature allow new leaks to be missed between inspections. These techniques may be well suited for baseline measurements and post-event confirmation but are not ideal for early leak detection and response.
Deploying multiple technologies and approaches does not automatically establish adequate coverage. When uncertainty and hesitation persist in critical applications, consequential events can remain undiscovered, unverified, or unaddressed for longer than necessary, thereby exacerbating the effects of a leak.
The Future is Automation
Alongside health, safety, and environmental (HSE) objectives, there is the pressure to maintain business profitability, operational efficiencies, and control over costs, pushing operators toward centralized operations management and automation tools.
“Remote maintenance, remote monitoring and control, and human/robot collaboration” are areas of greatest automation potential in the upstream and midstream sectors, according to a McKinsey Global Institute study (Fig. 1). The findings also indicated a 50% increase in worker efficiency and asset use as well as a 40% reduction in maintenance cost when repetitive work automation is combined with other approaches.
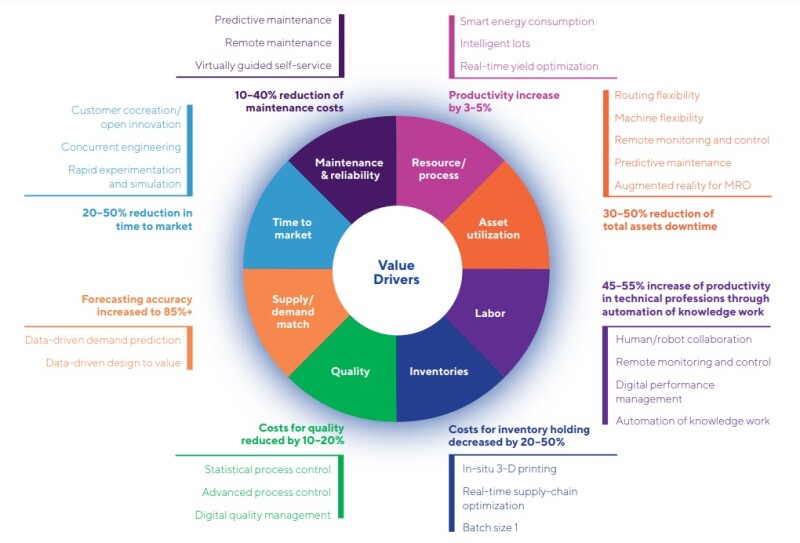
For decades, surveillance cameras have dominated the security and safety arena for the quality of information provided and their role in improving resource efficiency. Recent hardware and software advancements have not only made camera technology viable but also catapulted it to “standard” status for certain leak-detection applications in the oil and gas industry. Handheld optical gas imagers (OGI), for one, are considered best practice and regulatorily mandated for inspections in North America.
Thermal Cameras for Automated Leak Detection
In choosing an imaging sensor for fixed, site-based leak monitoring automation, some key factors are acquisition and operation costs, performance reliability in challenging situations, and the optimal accuracy-versus-sensitivity equilibrium for its intended application.
Longwave infrared (LWIR) imagers or microbolometers reveal things invisible to the naked eye and are relatively low cost with no maintenance, unlike their cooled counterparts. The technology creates grayscale images by converting the heat signature of objects as absorbed within 7–14 μm IR spectrum range. LWIR provides consistent day/night images without ambient lighting and is impervious to heavy rain, fog, and snow, making it suitable for both indoor and outdoor environments. It visualizes fluids (water, oil, bitumen, brine), vapor, natural gas liquids, fire, flame, people, vehicles, and wildlife and sees temperature differences at least 2°C down to the pixel.
LWIR OGIs recently emerged as an economical alternative to cooled midwave IR cameras. The technology detects large methane releases (at least 3 g/s or 10 kg/h) that come from superemitters, which include well pads, pipelines, and gas production, gathering, and compressing facilities, according to NASA.
Outside of security surveillance, color cameras have seen limited use for leak monitoring. This is partly because illumination is vital to their deployment, which restricts suitability to indoor or electricity-powered environments. Color videos also lack the sophistication of radiometric data collected from IR sensors. The technology is adequate for detecting larger and more-prominent leaks in less-complex environments but struggles with targets smaller and harder to distinguish from the background.
Combining color cameras with thermal cameras, either as supporting or duplicate sensors, is more effectual and beneficial (Fig. 2). High-definition color videos and pictures enhance thermal video evaluations and remote inspections. In conventional dual/multicamera configurations, the imagers are separate distinct products. The distance created by mounting limitations makes aligning both fields of view challenging. Newer setups incorporate the thermal and color sensors inside one housing.
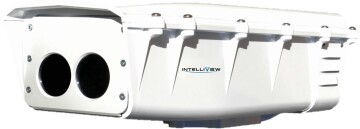
The performance and capabilities of imaging sensors will differ depending on their technical specifications. Field of view (FOV), sensitivity, and form factor (e.g., all-weather, dust resistance, explosion proof) determine sensor fit with the intended site or application. For instance, a camera with a narrow FOV would be appropriate for expansive areas while a camera with a wider FOV will provide visibility of closer subjects in tighter, equipment-dense spaces. The architecture can consist of a single camera or multiple cameras of the same or varying specifications to achieve optimal coverage (Fig. 3).
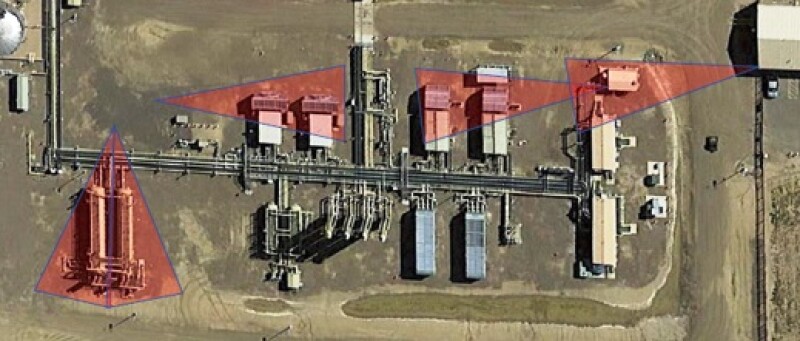
Artificial Intelligence Powered Vision
Powering cameras with artificial intelligence (AI) automates time-intensive and costly manual monitoring. AI allows cameras to be installed at the edge and operate autonomously and continually. In addition to leak detection automation, AI vision generates alerts that include video/photographic evidence to assist with remote confirmation (Fig. 4). If available, pre/post videos can be examined for supplemental forensics.
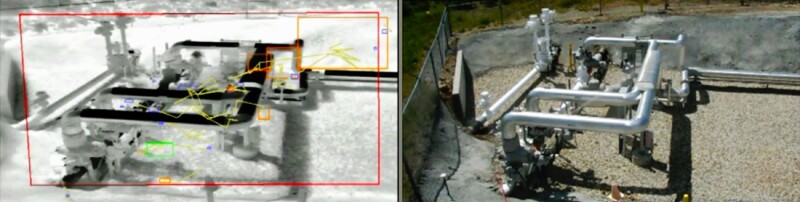
AI processing is executed in the cloud or on site. Cloud-based AI, although a favorite buzzword, necessitates continuous video streaming to a remote server for analysis. This process incurs high data charges. Another drawback of cloud processing is pretransmission raw footage compression diminishes thermal video quality, which may affect alarm reliability.
There is also the limitation in running cloud AI on color videos, which typically involves applying classification to the image snapshot rather than to motion. This technique can hinder analytic potential overall and affect accuracy because fluid, vapor, and gas leaks are dynamic elements that may look different frame by frame.
In oil and gas applications, placing the analytic engine locally has several advantages over cloud computing. Edge AI works faster (real time) and transmits only alarm information, which keeps data costs to a minimum. The analytic software is applied to uncompressed, highest quality radiometric data the sensors can produce at the pixel level. This results in improved accuracy and sensitivities and greater machine vision visibility of smaller or distant targets.
It is imperative to define the role of AI when pairing with vision technology. Using a higher sensitivity sensor does not necessarily lead to improved automated decisions. The formula should factor AI software intelligence, encompassing accuracy range, proclivity to err, and ability to determine whether the leak identification from all the collected data is reliable.
Key Capabilities of AI-Powered Thermal Cameras
- Continuous monitoring, automated detection and alerting (including visual evidence)
- Multiregion detection per camera for simultaneous monitoring of different materials or events (e.g., leaks and intrusion)
- Dual-sensor configuration
- Side-by-side display of thermal and color videos, matching perspectives and improving ease of operator confirmation
- Individual analytic programming of the thermal and color sensors
- Use of multiple analytic tools (e.g., algorithms, detection parameters, environmental filters, machine learning, deep learning neural net object classification, and edge and cloud processing)
- Solution optimization and customization—cost-effective and faster if provided by a supplier with in-house development expertise compared with a third-party AI partner
An easy-to-use, efficient, and scalable video management system (VMS) is essential to any AI vision solution. Long-term value can be gained from a VMS platform that supports future expansions through addition of cameras, accessories, and new capabilities by combining leak detection with other solutions, such as security and access control. Must-have VMS features include multiple display options (multiple views, zoom/full screen), various reporting interfaces [e.g., control room, email, supervisory control and data acquisition (SCADA) system, and phone/web app], and secure remote access to on-site devices, settings, and media.
Improving HSE Performance by Accelerating Detection and Decision-Making
Early leak detection and fast response are key to improving asset and worker safety and reducing environmental footprint. Computer vision arms operators with prevalidated, visually supported information and the confidence needed to take prompt action.
Enhanced Awareness and Decision-Making. Visual alerts from AI cameras aid in discovery, location, and validation of leaks. Unlike periodic visual methods and automated nonvisual detectors, continuous AI vision can detect new product releases within seconds of starting and provide confirmation by sight. Leak alert videos and live feeds can be used by first responders and maintenance staff to remotely confirm the event, safely assess asset condition, and determine the right response toolset. The same knowledge may be imparted to warn unaware workers onsite of the location and status of emerging dangers, allowing them to proceed with care.
Leveraging the industrial Internet of Things and wireless infrastructure presents an opportunity to oversee distributed assets via a single access point from the control room, with the possibility to extend to other web or mobile interfaces. Integrability with third-party sensors and end-user control systems, such as SCADA systems, promotes greater control and coordination to facilitate effort reduction and quicker response times. A specific case in point is a midstream company in Texas that paired an AI leak-detection camera with secondary sensors serving as a Modbus voting system to augment the effective range of detection and initiate autonomous shutdown.
Improved Leak Detection and Validation Capabilities. Fixed AI vision technology enhances monitoring in various ways. It expands coverage when implemented with existing systems or replaces less-efficient approaches. Despite the absence of a light source, LWIR or thermal cameras possess good nighttime vision and expose objects indiscernible to an operator.
Higher specification thermal cameras may be the automatic choice for better image resolution and detection sensitivities, but effective AI is required to translate that information into a reliable autonomous decision that a leak has begun. Advanced AI vision should be able to detect small releases from a range of 60–80 m, for example 0.4 L/s, which is up to 40 times smaller than CPM’s detection limit. It works in areas of the physical plant subject to normal operational flow changes and pressure variations and can isolate issues to the point of failure. The technology is also effective for monitoring other events that can affect oil and gas operations: fire, flare stack flame ignition failure/restoration, and critical temperatures.
As with any automated technology, false alarms are a universal quandary. An excessive number of untrue alarms can lead to inefficiencies in response and worker fatigue. Environmental noise, obstructions, and the technology’s limited performance bracket are some of the main contributors of false alarms. Reduction techniques typically involve a combination of software technologies, including video analytics, machine learning, supervised object classification, environmental filters, settings finetuning, and other tools specific to the cause of the imprecisions. Accuracy also is affected by analysis time and detection sensitivity levels. Both shorter analysis periods and excessive sensitives can lead to generation of too many false alarms. Determining the optimum balance of detection sensitivity and rate of acceptable daily false alerts at the solution design phase leaves sufficient time to fine tune and risk optimize at the configuration and commissioning stages.
The industry is increasingly recognizing the combined capabilities of AI vision as demonstrated by the technology’s standardization by an oil and gas major and international awards dedicated to HSE contribution.
Business Benefits
Wide-ranging business benefits can be expected from implementing continuous automated vision. Facilitating speedy leak response helps reduce or eliminate expensive effects, including product loss, cleanup bills, financial liabilities, regulatory fines, and safety compensations. Minimalized human involvement, fewer field trips, and better workforce allocation all contribute to operational cost savings, with freed labor hours redistributed to more important tasks, such as repair and maintenance.
These outcomes are supported by research, including a 2021 third-party-led efficiency review of a global energy producer. The study showed that, after installing AI leak detection cameras at onshore and offshore sites, the company was able to cut down site-visit-related wages and fares by 50% and decrease manual video monitoring efforts by up to 90%.
Thanks to the industrial Internet of Things, adding cameras to the system to adapt to growth carries minimal effect on operating expenses and workforce requirements. Business-smart alternatives to consider include green, renewable energy to reduce billable energy consumption and leveraging existing structures for mounting, such as piping racks and poles, to lower installation costs.
Advancements in AI Vision Leak Detection
Driven by the rising demand and interest around AI, future advancements hold significant promise in the continued enhancement of the capabilities, processing power, and accuracies of automated vision leak detection systems. The computing skills of video AI, previously only offered in OGIs, are being applied to LWIR imaging for liquid leak quantification. When measurable data (e.g., flow rate) is combined with visual proof and leak location, the alert package becomes more definitive and actionable. The performance envelope of AI-driven vision can be expanded further by running the analytics on both sensors of a dual camera system and capitalizing on their combined advantages.
Overall, continuous intelligent vision presents an effective, economical, and scalable approach to enhancing leak-monitoring capabilities and expediting response times, which, in turn, deliver significant advantages in HSE, profitability, and compliance. A robust integrity system consists of preventative, defensive, and reparative elements. An overlapping approach to leak detection—encompassing predictive, balance, sight, smell and sound—is equally warranted for high-risk and sensitive facilities to ensure thorough coverage. A system that models facility types, volumes, and locations and applies most suitable elements of an integrated best-in-class toolset will emerge to streamline and reduce costs in the planning process. As industry directives become more stringent and visual verification gains significance in executive decision making and action, AI cameras are well poised to assume a more prominent role in automating monitoring processes and expanding human potential for decision making and capacity.