This paper delves into the framework of a new application that leverages advanced machine-learning (ML) techniques in conjunction with metocean forecasts to predict vessel motions and thruster loads. The discussion encompasses the key role of diverse input factors in shaping prediction accuracy and outlines strategies to address inherent uncertainties associated with methods, weather forecasts, and the stochastic nature of the underlying problem.
Methods, Procedures, and Process
Traditional Physics-Based Vessel Model. A physics-based model of the vessel is used to train the ML model. As such, the accuracy of the physics-based model is critical to the success of the ML model. The model is built using vessel-specific hydrodynamic data, mass data, and thruster-control software.
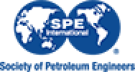