In the complete paper, the authors propose an artificial-intelligence (AI)-assisted work flow that uses machine-learning (ML) techniques to identify sweet spots in carbonate reservoirs. This process involves annotation of geologic features using a well database, with supervision from subject-matter experts (SMEs). The resulting ML model is tested on new wells and can identify pay zones, perforation intervals, and stress analysis. The models successfully detect fractures, breakouts, bedding planes, vugs, and slippage passages with pixel-level precision, reducing borehole-image (BHI) analysis time.
BHI Interpretation and Preprocessing
The use of BHIs requires manual interpretation and data identification, heavily relying on the expertise and time of SMEs. A widely adopted approach to address this challenge is the use of supervised computer-vision algorithms, a subfield of AI. These algorithms optimize the task function or model based on examples they have learned from data during training.
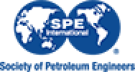